Financial Feature Embedding With Knowledge Representation Learning For Financial Statement Fraud Detection
2020 INTERNATIONAL CONFERENCE ON IDENTIFICATION, INFORMATION AND KNOWLEDGE IN THE INTERNET OF THINGS (IIKI2020)(2021)
摘要
Identifying fraudulent financial statement is critical for capital market regulation and is generally formulated as a classification problem. Feature selection in traditional machine learning methods does not consider correlation information among financial features which may influence performance of classifiers. To explore correlation information on conducting financial statement fraud detection (FSFD), we combine traditional features with knowledge graph models, and learn new representations enriched with feature embedding of various financial categories. These feature relations defined by correlation types may form knowledge graphs with features as nodes and correlation relations as edges. Experimentations demonstrate that financial feature representations with correlation information significantly improve classification performances for SVM and K-NN, marginally better than decision trees and logistic regression, but not outperforming naive Bayes (Kernel). (C) 2021 The Authors. Published by Elsevier B.V. This is an open access article under the CC BY-NC-ND license (https://creativecommons.org/licenses/by-nc-nd/4.0) Peer-review under responsibility of the scientific committee of the International Conference on Identification, Information and Knowledge in the internet of Things, 2020.
更多查看译文
关键词
Financial statement fraud, Graph embedding, Correlation information
AI 理解论文
溯源树
样例
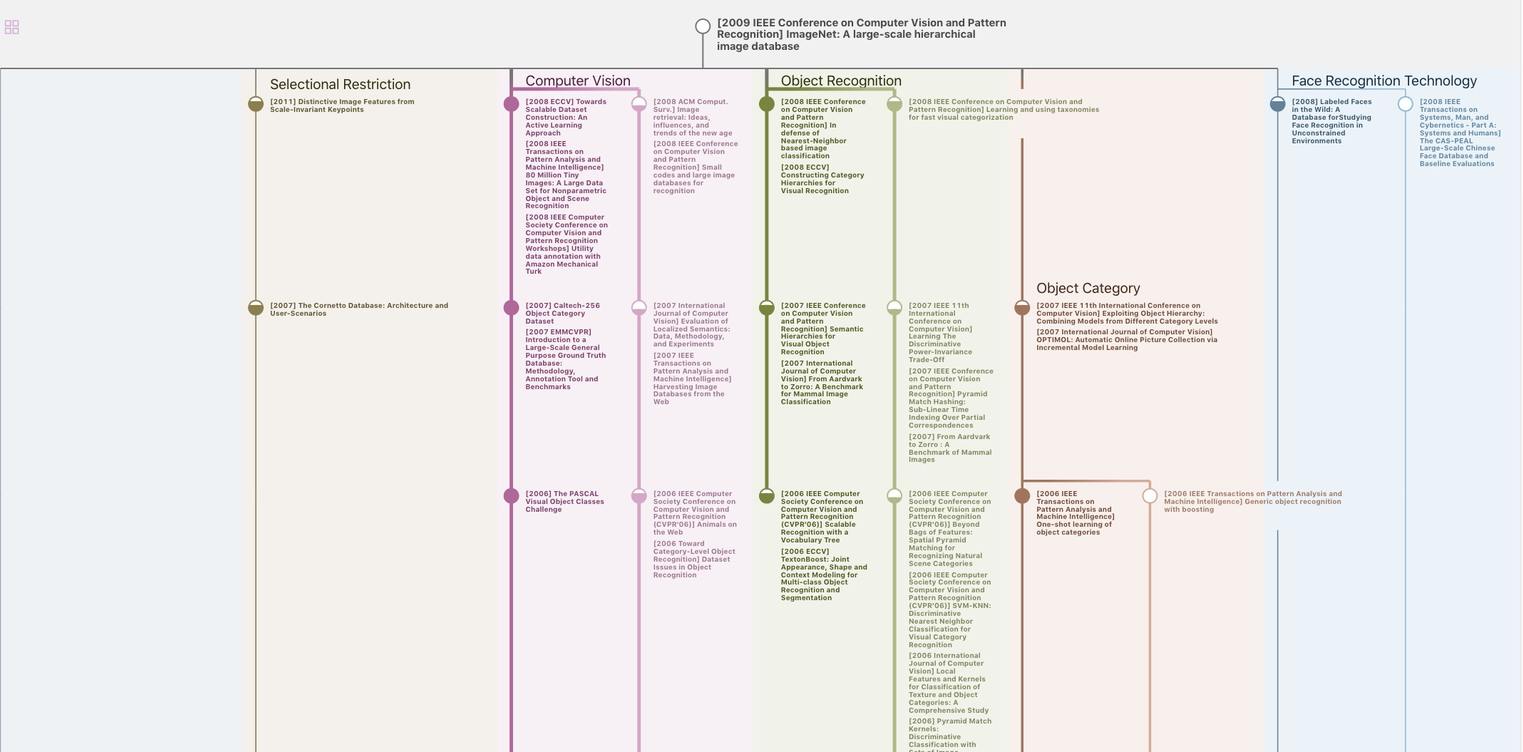
生成溯源树,研究论文发展脉络
Chat Paper
正在生成论文摘要