Decoupling Non-Polynomial Functions: A Neural Network Example
IFAC PAPERSONLINE(2021)
摘要
The decoupling algorithm proposed by Dreesen et al. (SIAM J. Matrix Anal. Appl., 2015) was developed for MIMO polynomials. Polynomial based models are commonly used in nonlinear system identification despite their poor extrapolation behaviour and the often poor numerical conditioning of the resulting estimation problems. Several alternatives have been used in the identification literature including: various spline representations, neural networks, and support vector machines. In this contribution, we consider the approximation of non-polynomial nonlinearities using a decoupled structure. The derivation of the decoupling algorithm is repeated, but without assuming a polynomial structure at any stage of the development. As an example, a NARX model employing a feedforward sigmoidal neural network as its nonlinearity is fitted to data from the Silverbox benchmark system. The resulting nonlinearity is then decoupled using the approach proposed in this contribution. The non-parametric filtered CPD is used to impose smoothness on the solution without imposing a polynomial constraint. Copyright (C) 2021 The Authors.
更多查看译文
关键词
Nonlinear System Identification, Decoupled Nonlinearity Approximation, Non-Polynomial Functions, Nonlinear Autoregressive Exogenous Input Models, NARX
AI 理解论文
溯源树
样例
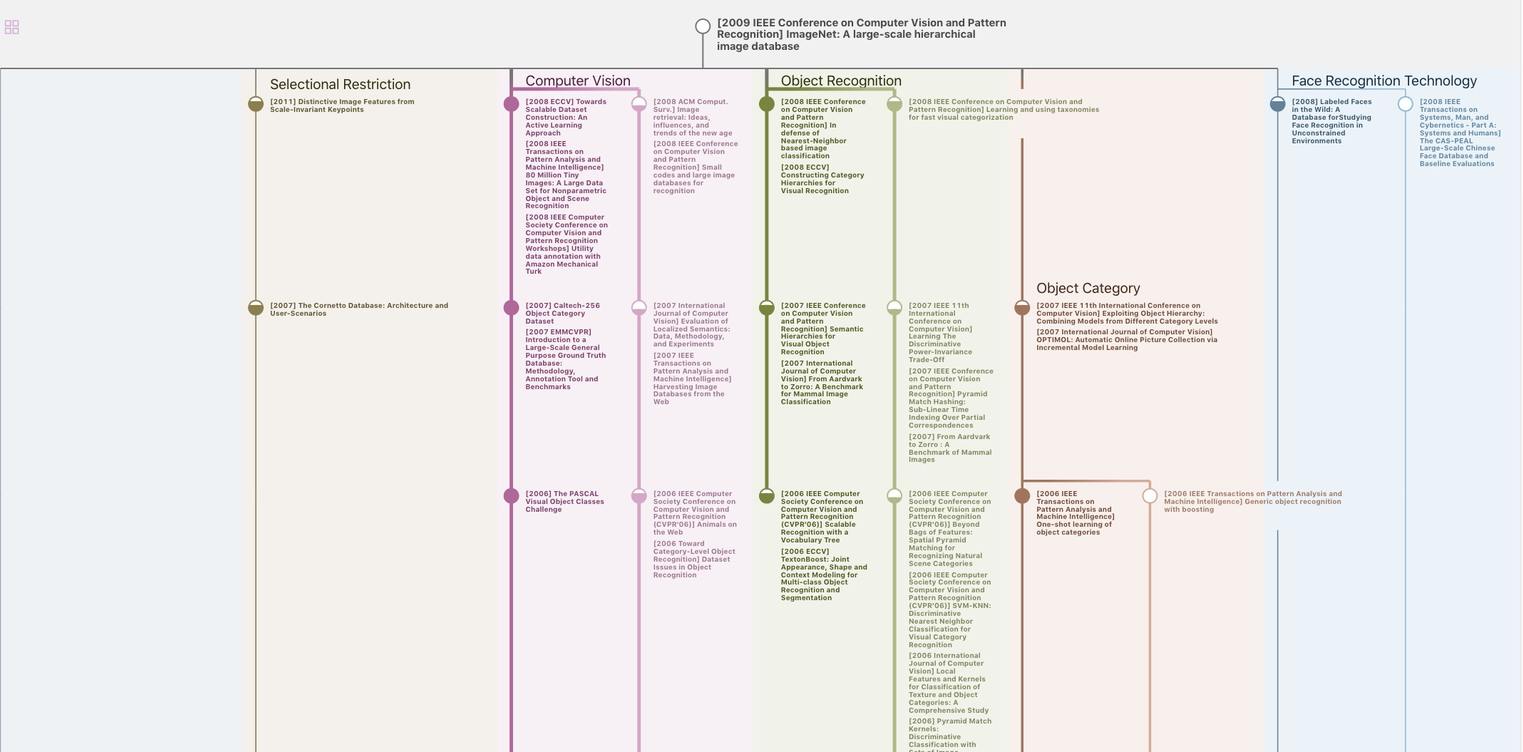
生成溯源树,研究论文发展脉络
Chat Paper
正在生成论文摘要