Energy-aware disaster backup among cloud datacenters using multiobjective reinforcement learning in software defined network
CONCURRENCY AND COMPUTATION-PRACTICE & EXPERIENCE(2022)
摘要
The transmission process of disaster backup with long distance and massive data causes huge energy consumption. Reducing the number of occupied intermediate forwarding devices and shortening the transmission completion time are two key factors for energy saving. Not jointly considering them, previous work can hardly realize optimal energy-aware transmission only by single objective optimization. For the first time, we leverage multiobjective reinforcement learning to simultaneously minimize the number of occupied intermediate forwarding devices and the transmission completion time in software defined network. We propose two-level reinforcement learning, consisting of search and selection operation. In the internal reinforcement learning, we aim to reduce hop number, improve node sharing degree, and give priority to the links with larger residual capacity. Then in the external level, we aim to reduce the total number of occupied devices and increase the total backup flow. We leverage Chebyshev scalarization function based on pseudo-random proportional rule to simplify weight selection, and enforce exploration to avoid falling into local optimum. We design the vector of rewards for different objectives, and update Pareto approximate set by multiple state steps to approach the optimal solution. Our strategy solves the weight selection problem successfully and achieves lower energy consumption.
更多查看译文
关键词
Chebyshev scalarization function, energy-aware disaster backup, software defined network, the number of intermediate forwarding devices, transmission completion time, two-level multiobjective reinforcement learning
AI 理解论文
溯源树
样例
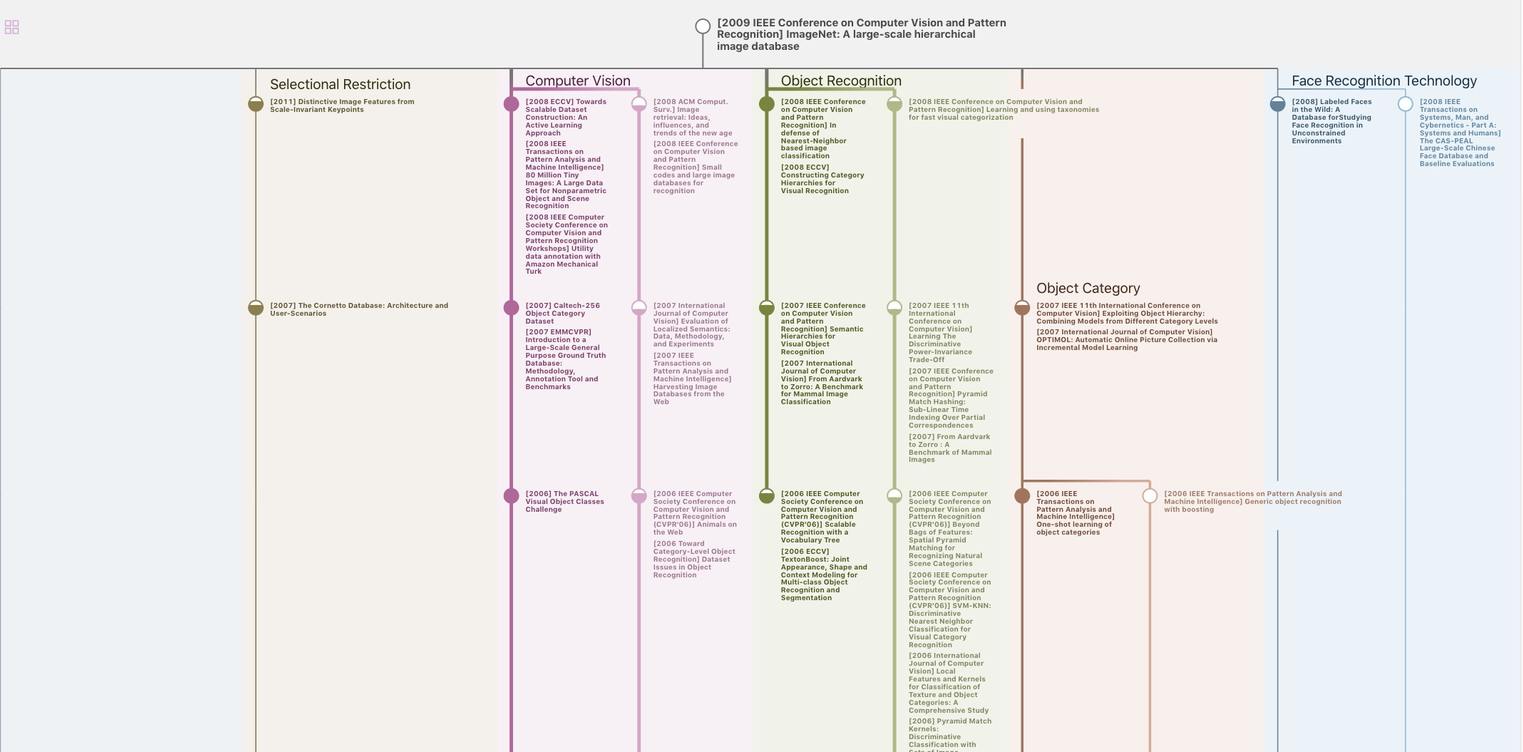
生成溯源树,研究论文发展脉络
Chat Paper
正在生成论文摘要