Cross-Domain Classification Of Physical Activity Intensity: An Eda-Based Approach Validated By Wrist-Measured Acceleration And Physiological Data
ELECTRONICS(2021)
摘要
Performing regular physical activity positively affects individuals' quality of life in both the short- and long-term and also contributes to the prevention of chronic diseases. However, exerted effort is subjectively perceived from different individuals. Therefore, this work explores an out-of-laboratory approach using a wrist-worn device to classify the perceived intensity of physical effort based on quantitative measured data. First, the exerted intensity is classified by two machine learning algorithms, namely the Support Vector Machine and the Bagged Tree, fed with features computed on heart-related parameters, skin temperature, and wrist acceleration. Then, the outcomes of the classification are exploited to validate the use of the Electrodermal Activity signal alone to rate the perceived effort. The results show that the Support Vector Machine algorithm applied on physiological and acceleration data effectively predicted the relative physical activity intensities, while the Bagged Tree performed best when the Electrodermal Activity data were the only data used.
更多查看译文
关键词
physical activity, electrodermal activity, wearable device, machine learning
AI 理解论文
溯源树
样例
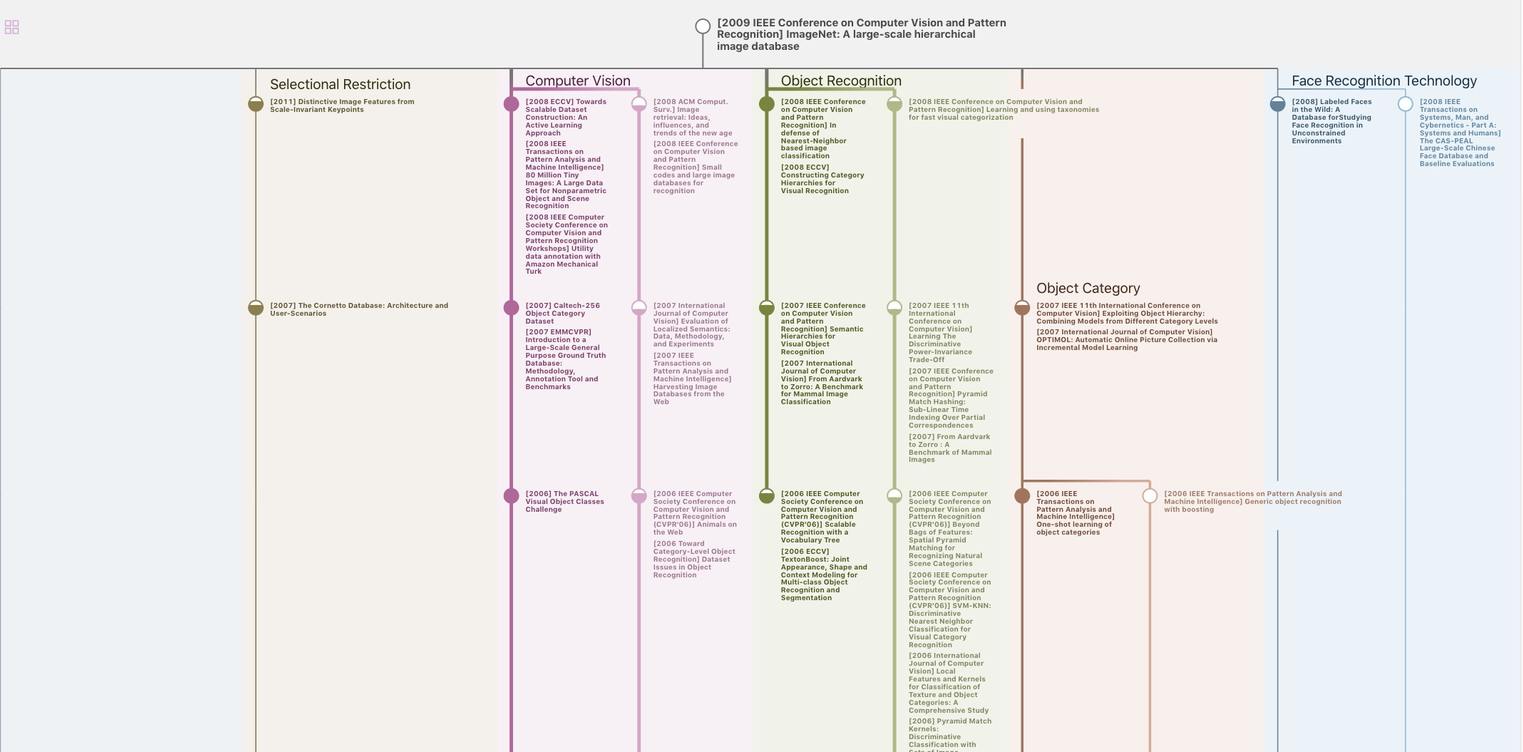
生成溯源树,研究论文发展脉络
Chat Paper
正在生成论文摘要