Fault Diagnosis Of Rolling Bearing Based On Whvg And Gcn
IEEE TRANSACTIONS ON INSTRUMENTATION AND MEASUREMENT(2021)
摘要
In recent years, emerging intelligent algorithms have achieved great success in the domain of fault diagnosis due to effective feature extraction and powerful learning ability. However, the current models can only handle the data in Euclidean space, ignoring latent structure relationships of the signal, which can provide additional helpful information to distinguish diverse fault patterns. To address this issue, a graph convolution network (GCN) incorporating the weighted horizontal visibility graph (WHVG) is proposed for bearing faults diagnosis. The WHVG is utilized to transform time series to graph data from a geometric perspective. Edges are weighted by the difference between the sampling indexes to weaken the influence of remote nodes that are considered as noise. Furthermore, the graph isomorphism network (GIN) is improved as GIN+ to learn the graph representation and perform fault classification. Finally, the validity of WHVG and GIN+ is testified by three real-world bearing datasets. Meanwhile, the GIN+ model is compared with other machine learning models, multilayer perceptron (MLP), long short-term memory (LSTM), and two GCN models. The experimental results show that GIN+ boosts the performance and the internal structure relationships of the data contribute to the bearing faults diagnosis.
更多查看译文
关键词
Fault diagnosis, graph convolution network (GCN), rolling bearing, weighted horizontal visibility graph (WHVG)
AI 理解论文
溯源树
样例
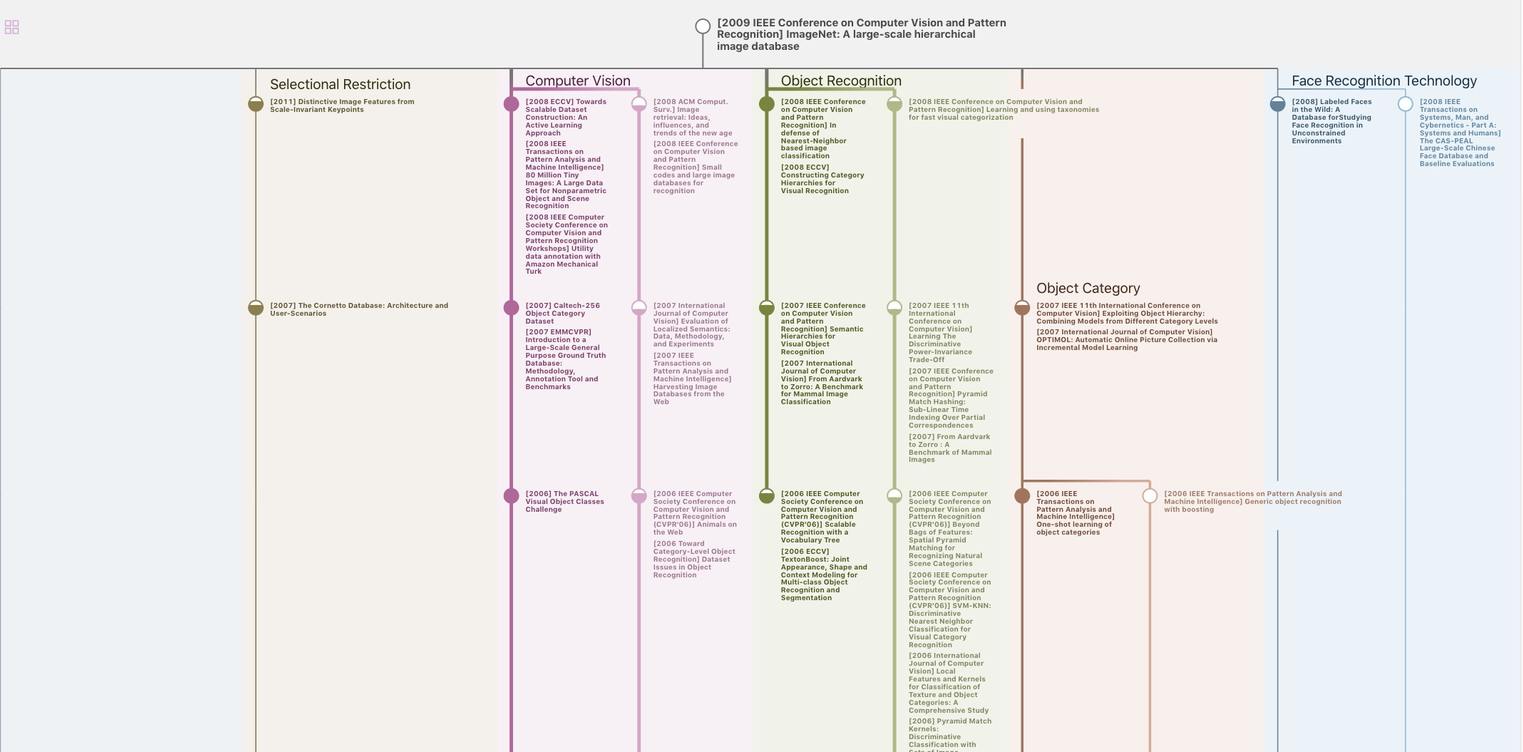
生成溯源树,研究论文发展脉络
Chat Paper
正在生成论文摘要