Multilabel Over-Sampling And Under-Sampling With Class Alignment For Imbalanced Multilabel Text Classification
JOURNAL OF INFORMATION AND COMMUNICATION TECHNOLOGY-MALAYSIA(2021)
摘要
Simultaneous multiple labeling of documents, also known as multilabel text classification, will not perform optimally if the class is highly imbalanced. Class imbalance entails skewness in the fundamental data for distribution that leads to more difficulty in classification. Random over-sampling and under-sampling are common approaches to solve the class imbalance problem. However, these approaches have several drawbacks; under-sampling is likely to dispose of useful data, whereas over-sampling can heighten the probability of overfitting. Therefore, a new method that can avoid discarding useful data and overfitting problems is needed. This study proposed a method to tackle the class imbalance problem by combining multilabel over-sampling and under-sampling with class alignment (ML-OUSCA). In the proposed ML-OUSCA, instead of using all the training instances, it drew a new training set by over-sampling small size classes and under-sampling big size classes. To evaluate the proposed ML-OUSCA, evaluation metrics of average precision, average recall, and average F-measure on three benchmark datasets, namely Reuters-21578, Bibtex, and Enron datasets, were performed. Experimental results showed that the proposed ML-OUSCA outperformed the chosen baseline random resampling approaches: K-means SMOTE and KNN-US. Therefore, based on the results, it can be concluded that designing a resampling method based on class imbalance together with class alignment will improve multilabel classification even better than just the random resampling method.
更多查看译文
关键词
Data mining, multilabel text classification, class imbalance problem, resampling method, class alignment
AI 理解论文
溯源树
样例
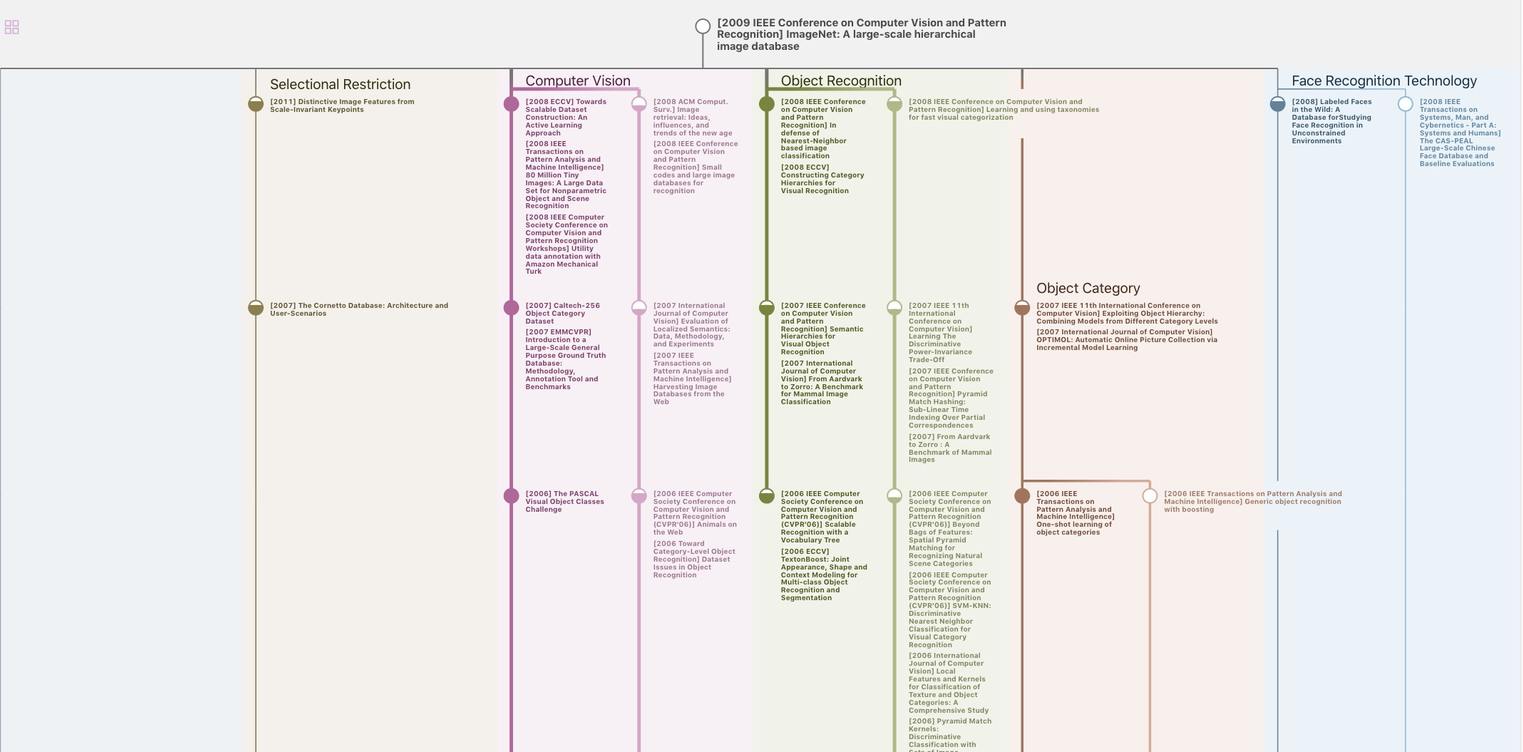
生成溯源树,研究论文发展脉络
Chat Paper
正在生成论文摘要