A Statistical Robust Glaucoma Detection Framework Combining Retinex, Cnn, And Doe Using Fundus Images
IEEE ACCESS(2021)
摘要
Motivated by the challenge that manual glaucoma detection is costly and time consuming, and that existing automated glaucoma detection processes lack either good performance or any statistical robustness testing procedures, we proposed an effective, robust, and automated framework for glaucoma detection based on fundus images. The proposed framework using 1450 color fundus images provided by Kaohsiung Chang Gung (KCG) Memorial Hospital in Taiwan. The proposed framework combines the use of convolutional neural networks (CNN) with the proposed generalized loss function, robust design of experiment (DOE), and Retinex theory to improve the results of fundus photography flash by restoring the original colors via removing the light effect. The proposed framework outperformed most archival automatic glaucoma detection approaches in its effectiveness and simplicity. The effectiveness was demonstrated via the estimated sensitivity 0.95, specificity 0.98, and accuracy 0.97. The simplicity was shown via the adopted basic CNN model compared to deep CNNs such as GoogleLeNet and ResNet152. Further, the proposed framework outperformed all relevant archival work in terms of its robustness, illustrated in the associated standard errors (all less than 0.03). This paper demonstrated the proposed framework via intuitive graphs and clear mathematical notations to make it easy for others to reproduce our results. The proposed framework and demonstration have the potential to become the standard automated glaucoma detection approaches in practice.
更多查看译文
关键词
Image color analysis, Support vector machines, Standards, Sensitivity, Principal component analysis, Optical sensors, Optical imaging, Glaucoma, CNN, DOE, robust, Retinex
AI 理解论文
溯源树
样例
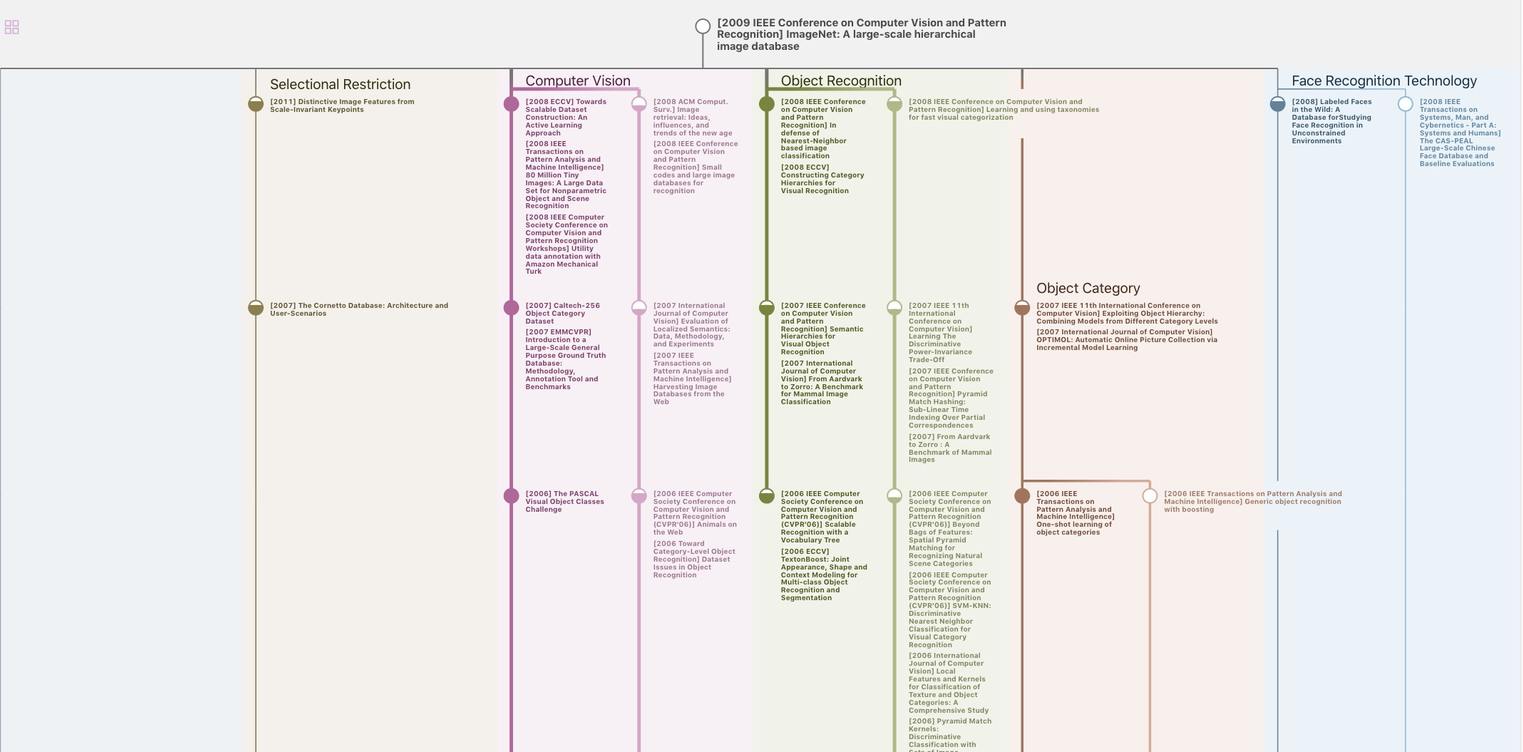
生成溯源树,研究论文发展脉络
Chat Paper
正在生成论文摘要