Soft Computing-Based Estimation Of Ultimate Axial Load Of Rectangular Concrete-Filled Steel Tubes
STEEL AND COMPOSITE STRUCTURES(2021)
摘要
In this study, we estimate the ultimate load of rectangular concrete-filled steel tubes (CFST) by developing a novel hybrid predictive model (ANN-BCMO) which is a combination of balancing composite motion optimization (BCMO) -a very new optimization technique and artificial neural network (ANN). For this aim, an experimental database consisting of 422 datasets is used for the development and validation of the ANN-BCMO model. Variables in the database are related with the geometrical characteristics of the structural members, and the mechanical properties of the constituent materials (steel and concrete). Validation of the hybrid ANN-BCMO model is carried out by applying standard statistical criteria such as root mean square error (RMSE), coefficient of determination (R-2), and mean absolute error (MAE). In addition, the selection of appropriate values for parameters of the hybrid ANN-BCMO is conducted and its robustness is evaluated and compared with the conventional ANN techniques. The results reveal that the new hybrid ANN-BCMO model is a promising tool for prediction of the ultimate load of rectangular CFST, and prove the effective role of BCMO as a powerful algorithm in optimizing and improving the capability of the ANN predictor.
更多查看译文
关键词
CFST column, artificial neural network, ultimate axial load, balancing composite motion optimization
AI 理解论文
溯源树
样例
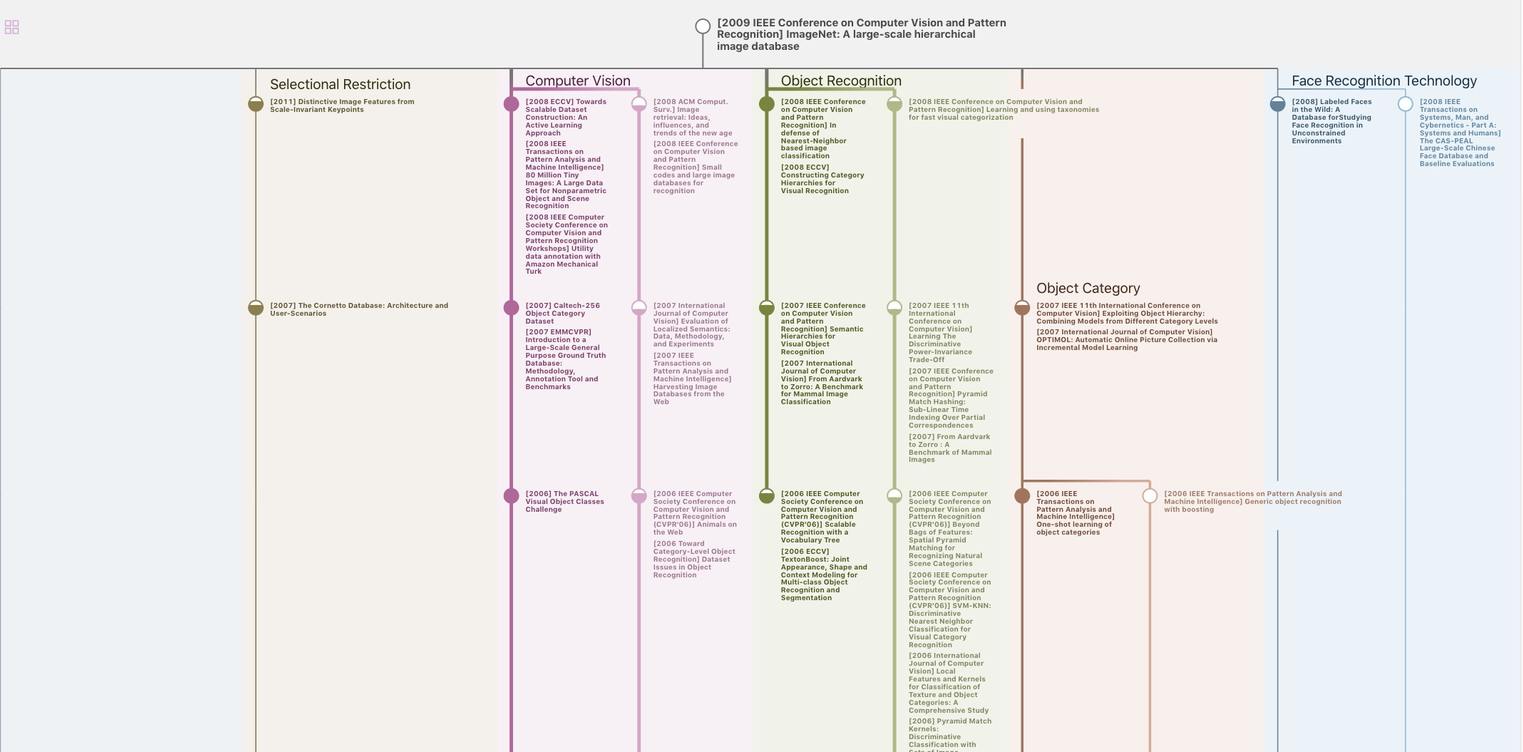
生成溯源树,研究论文发展脉络
Chat Paper
正在生成论文摘要