Approach For Improving Yolov5 Network With Application To Remote Sensing Target Detection
JOURNAL OF APPLIED REMOTE SENSING(2021)
摘要
Remote sensing images have the characteristics of a multi-scale, high resolution, and complex background, making the accuracy of small target detection too low. We propose an improved method based on the "you-only-look-once" v5 network model to make it more suitable for small target detection. First, we design a four-scale detection layer that improves the depth of the network and the accuracy of small target detection. Then, we introduce complete intersection over union-non-maximum suppression (NMS) to replace the commonly used NMS as a suppression of the prediction bounding box, and it also considers the overlap area, center distance, and aspect ratio to improve the accuracy of predicting bounding boxes. Furthermore, the remote sensing image is segmented into several fixed-size smaller images, making its size suitable for the input of the model to avoid target loss caused by directly scaling the size. The experimental results show that, compared to the four available detection methods, the improved algorithm significantly improves the detection accuracy of targets from small aircraft and ships in complex environments. The miss detection rate is also greatly reduced, especially for small targets, which verifies the effectiveness and robustness of the algorithm. Moreover, the detection rate has been greatly improved, which can meet the demand for real-time detection. (C) 2021 Society of Photo-Optical Instrumentation Engineers (SPIE)
更多查看译文
关键词
remote sensing image, YOLOv5, multi-scale, complete intersection over union-non-maximum suppression
AI 理解论文
溯源树
样例
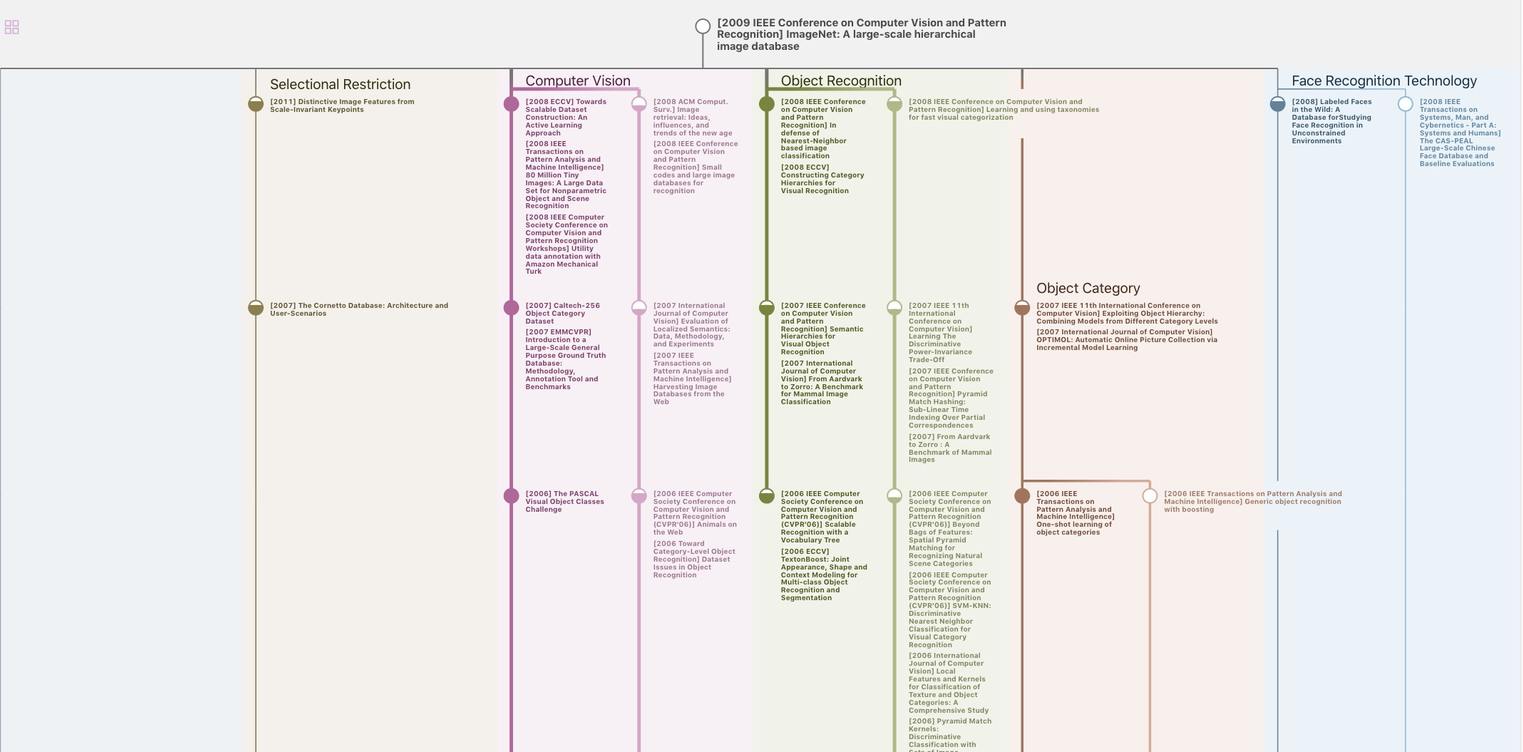
生成溯源树,研究论文发展脉络
Chat Paper
正在生成论文摘要