Deterministic Modeling Of Solidification Microstructure Formation In Directed Energy Deposition Fabricated Ti6al4v
ADDITIVE MANUFACTURING(2021)
摘要
Metal additive manufacturing (MAM) technology is now widely applied in the manufacturing and remanufacturing industry, among which microstructure simulation gradually shows its importance. Traditional solidification microstructure simulation methods all have their merits as well as drawbacks when applied in MAM cases. In this work, a deterministic solidification microstructure model, named "invasion model", is established to avoid the essential defects of traditional methods. This model focuses on the interaction between the neighboring bi-crystals instead of simulating the growth kinetics of each columnar grain or deriving the field form of variables. Within a bi-crystal system, the grain boundary tilt from thermal gradient direction is understood as a transient invasion behavior of one grain to another, and the competitive grain growth behavior along the buildup process of MAM is a summary of all the invasions in bi-crystal systems. To fill the gaps in the rapid solidification theory, a database recording the anisotmpic growth effect under a rapid directional solidification condition was established with the help of an artificial neural network (ANN). Wire feed directed energy deposition (DED) fabricated Ti6Al4V thin-wall samples with full dendritic columnar grains (prior beta) are used as a benchmark to test the validation of the novel simulation model. The grain geometrical structure of reconstructed prior beta grains along the build-up direction has a good agreement with the simulation result. The model can also be applied to other cases of MAM or combine with various models to achieve real-time as-solidified crystallographic feature prediction when it meets the scope of application.
更多查看译文
关键词
Additive manufacturing, Microstructure, Modeling, Solidification
AI 理解论文
溯源树
样例
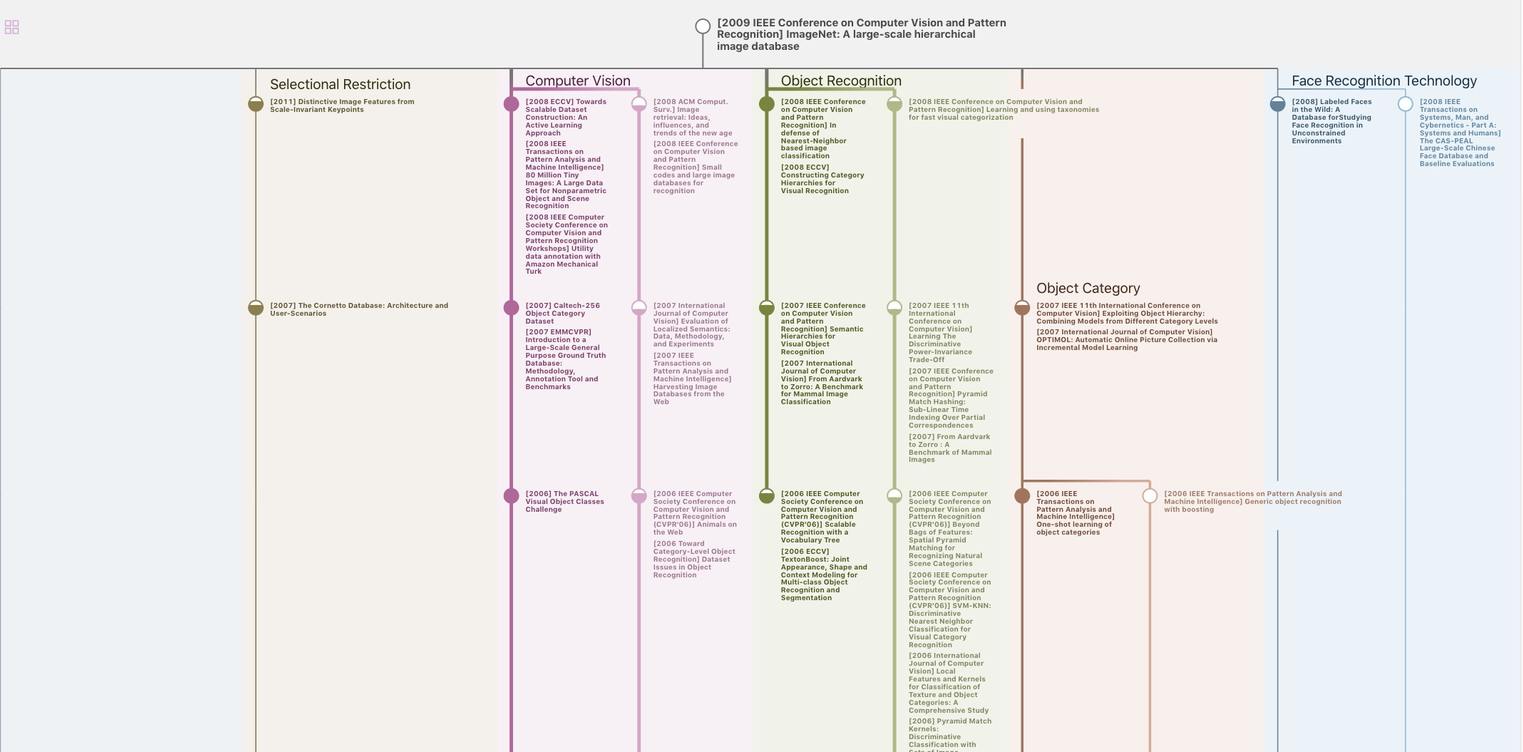
生成溯源树,研究论文发展脉络
Chat Paper
正在生成论文摘要