Estimating Abundance Based On Time-To-Detection Data
METHODS IN ECOLOGY AND EVOLUTION(2021)
摘要
1. Many studies in ecology and management aim at quantifying absolute abundance based on counts at a set of surveyed sites. As time for data collection is typically limited, methods for reliable estimation of occupancy or abundance from low-cost data are desirable. Time-to-detection (TTD) models have shown promise for the estimation of occupancy. However, they remain heavily underutilized, and restricted to inference about occupancy, rather than abundance.2. We developed a binomial N-mixture model for species-level TTD protocols that allows estimation of abundance with multiple-or single-visit data. An extension of the multi-visit version allows estimating availability per visit, given temporary emigration is random. We provide JAGS code and a new function (nmixTTD) in the R package unmarked for fitting a variety of such models.3. Simulations showed accurate parameter estimation from single-visit species-level TTD data if individual detection probability is high (>=similar to 0.7) and the number of visited sites is in the hundreds (>=similar to 300). Additional visits improved the accuracy of estimates considerably. A comparison with the Royle-Nichols-and the classic binomial N-mixture-model revealed that the performance of our model is between these two, but requires data that are less expensive and less error-prone than count data required for binomial N-mixture-models. In a case study, we found similar results when analysing data with the Royle-Nichols-, the binomial N-mixture-model or the multi-visit version of our TTD model. Analysing single-visit data with our model yielded lower abundance and higher detectability estimates. Presumably these differences are due to temporary emigration, as the single-visit method estimates the abundance of individuals available at one sampling occasion, whereas the multi-visit methods refer to the superpopulation, that is, the number of individuals present over the study period.4. Our new TTD-N-mixture model shows promise because it enables estimation of abundance, corrected for imperfect detection, for single-and multiple-visit data, based on data that are less expensive and that will be available in large quantities in the near future thanks to technical advances like autonomous recording units. The effects of unmodelled heterogeneity in detection rate and imperfect availability require further study.
更多查看译文
关键词
binomial mixture models, imperfect detection, Royle-Nichols model, species-level TTD, time-to-detection, time-to-event
AI 理解论文
溯源树
样例
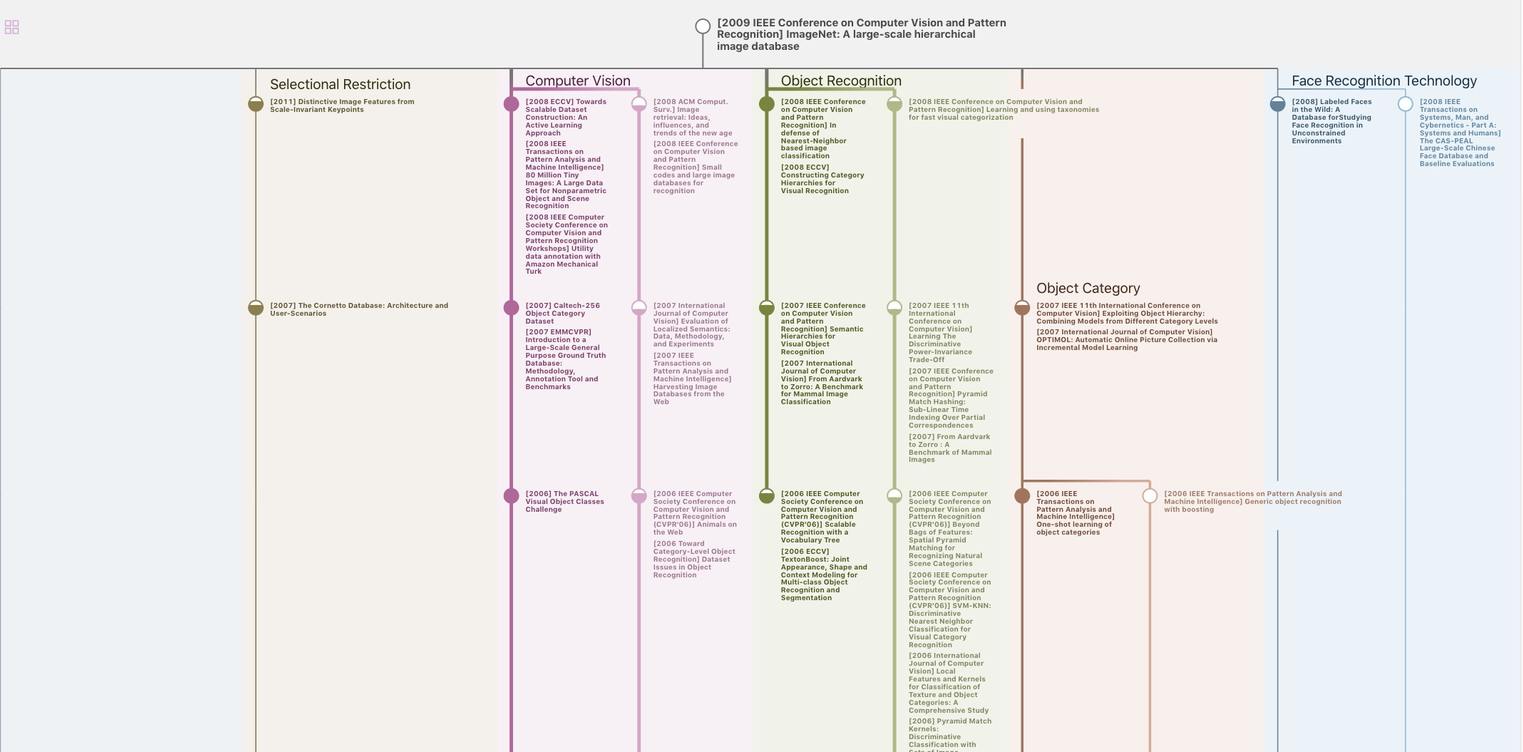
生成溯源树,研究论文发展脉络
Chat Paper
正在生成论文摘要