A Compact Deep Learning Network for Temporal Sleep Stage Classification
2018 IEEE Life Sciences Conference (LSC)(2018)
摘要
Sleep stage classification is usually performed by trained professionals using visual inspection of bio-electrical recordings from a subject and is the first step in quantifying the quality of sleep and diagnosing sleep disorders. We introduce an extensible, modality-agnostic deep learning system to automate the task of temporal sleep stage classification from raw electroencephalography, electrooculography and electromyography signals. The proposed architecture uses a combination of convolutional neural networks (CNN) and recurrent neural networks (RNN). The compact size of the system makes it not only computationally efficient but also more appropriate for smaller datasets. We evaluated the proposed system on a sleep dataset collected in a home environment from healthy subjects and found that the incorporation of temporal information (sleep stage transitions) boosted overall performance in terms of macro-average F1 scores, and in particular provided a significant improvement for the worst performing class, N1 compared to other approaches.
更多查看译文
关键词
Automatic sleep stage classification,Deep learning,Convolutional neural network (CNN),Long Short-Term Memory (LSTM)
AI 理解论文
溯源树
样例
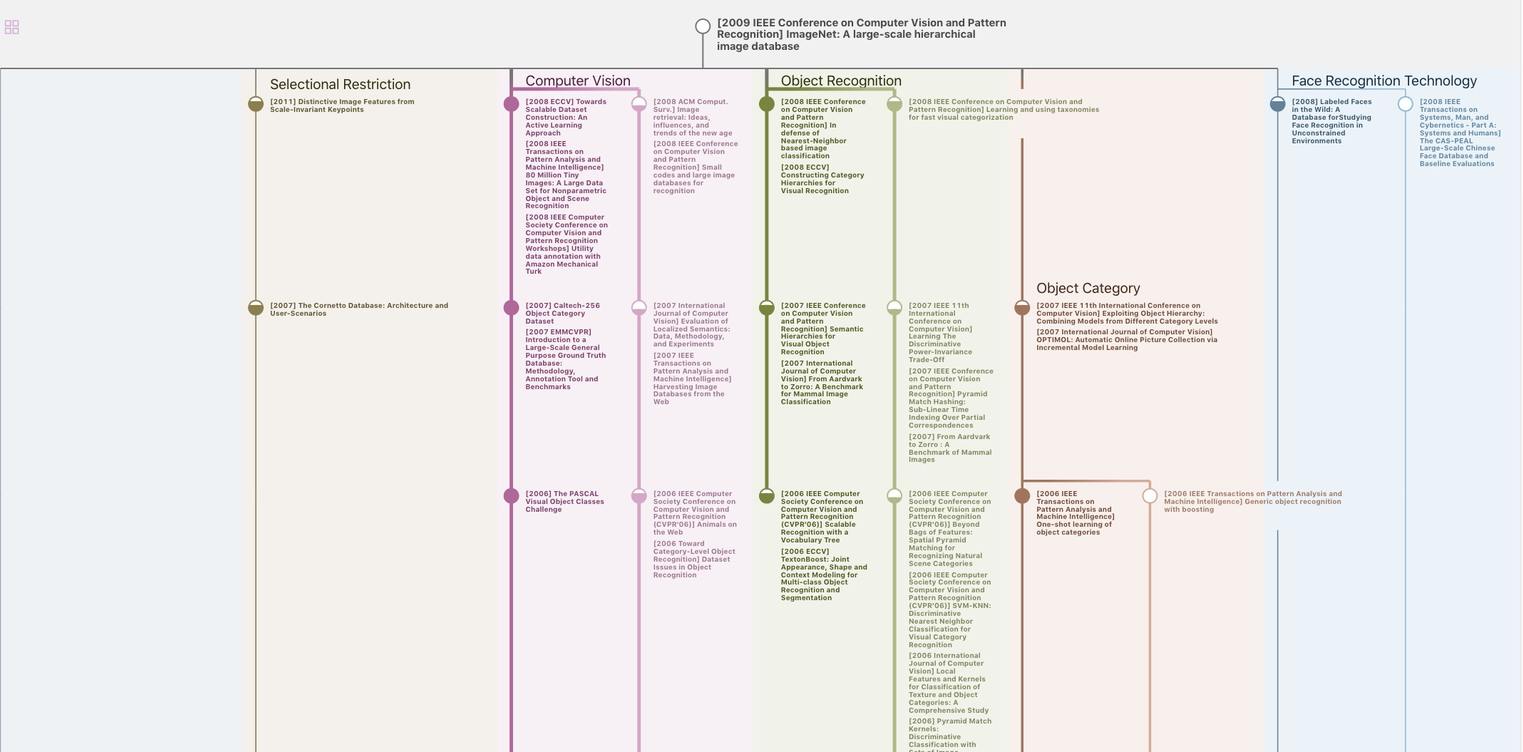
生成溯源树,研究论文发展脉络
Chat Paper
正在生成论文摘要