Rapid Prescreening Of Organic Compounds For Redox Flow Batteries: A Graph Convolutional Network For Predicting Reaction Enthalpies From Smiles
BATTERIES & SUPERCAPS(2021)
摘要
Identifying interesting redox-active couples from the vastness of organical chemical space requires rapid screening techniques. A good initial indicator for couples worthy of further investigation is the heat of reaction Delta H degrees. Traditional methods of calculating this quantity, both experimental and computational, are prohibitively costly at large scale. Instead, we apply a graph convolutional network to estimate the heats of reaction of arbitrary redox couples orders of magnitude faster than conventional computational methods. Our graph takes only SMILES strings as input, rather than full three-dimensional geometries. A network trained on a dataset of atomization enthalpies for approximately 45,000 hydrogenization reactions, applied to a separate test set of 235 compounds and benchmarked against experimental heats of reaction, produces promisingly accurate results, and we anticipate that this methodology can be extended to other RFB-relevant reactions. However, lower predictivity for compounds in regions of chemical space not covered by the training dataset reinforces the pivotal importance of the particular chemistries presented to a model during training.
更多查看译文
关键词
ab initio calculations, electrochemistry, graph convolutional network, reaction enthalpies, redox flow batteries
AI 理解论文
溯源树
样例
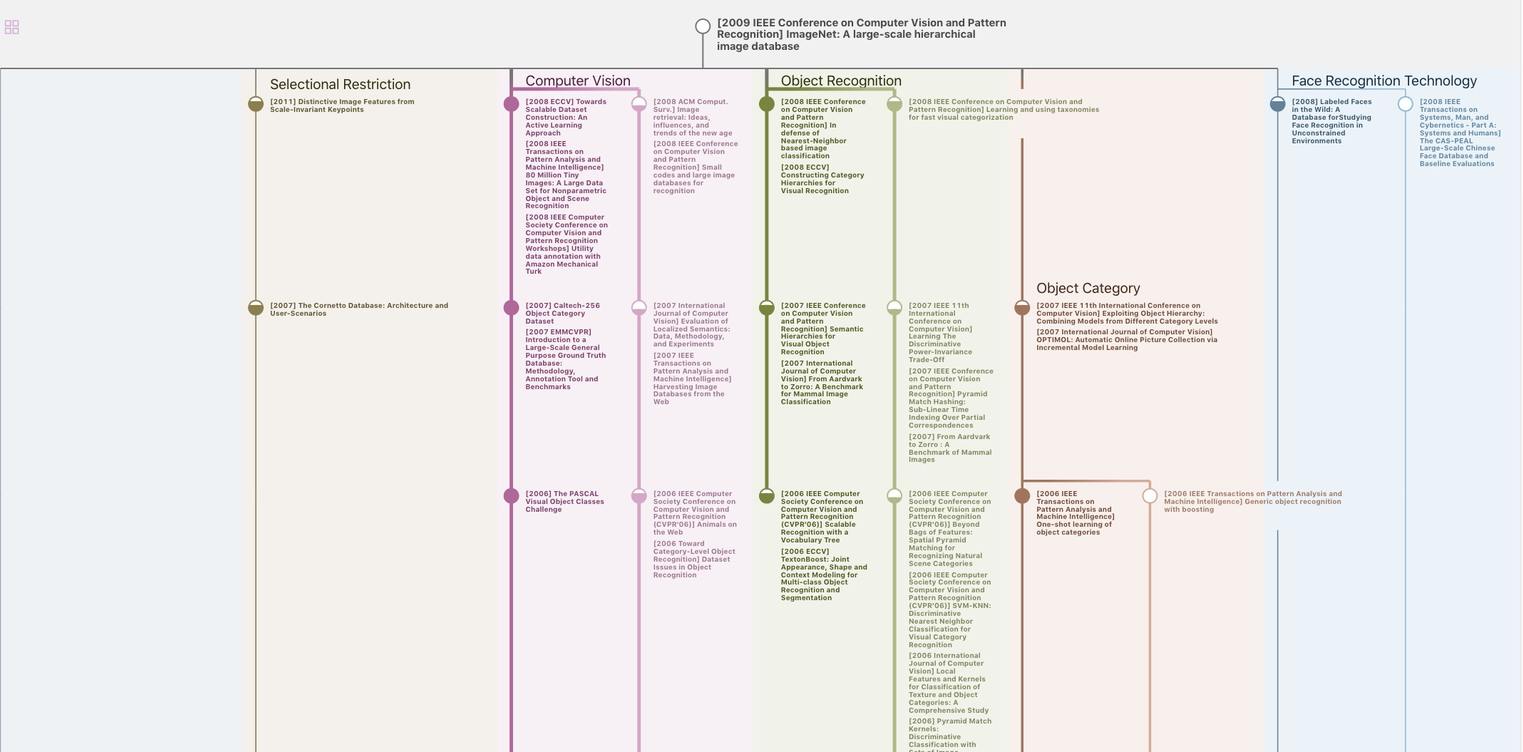
生成溯源树,研究论文发展脉络
Chat Paper
正在生成论文摘要