Effective Rate Evaluation of RIS-Assisted Communications Using the Sums of Cascaded α-μ Random Variates
IEEE ACCESS(2021)
摘要
This paper begins with the characterization of the sum of $M$ versions of cascaded $\alpha $ - $\mu $ (equivalently, $N*(\alpha $ - $\mu$ )) distributed random variates (RVs), and later on, explores the feasibility and applicability of the obtained results in the recent proposed reconfigurable intelligent surface (RIS)-assisted communications. More specifically, the sum of cascaded $\alpha $ - $\mu $ distributed RVs is exactly characterized by the probability density function (PDF) expressed in terms of the programmable multivariate Fox’s $H$ -function, and approximated with the mixture of Gaussian (MoG) distribution. The approximation accuracy is further validated by the Kolmogorov-Smirnov (KS) goodness of fit test. Based on the obtained statistical results, a promising application, i.e., RIS-assisted communication, is considered and evaluated from the effective rate perspective. The exact, asymptotic, and approximated effective rate are derived with closed-form expressions. Finally, our analytical results are further validated using the Monte-Carlo simulation, their accuracy is confirmed with the analytical error indicator. One can conclude that (i) the exact effective rate expression is complex in the manner of mathematical representation; (ii) the MoG-based effective rate expression is simple and provides a highly tight approximation to the exact expression, besides it indeed acts as a highly tight upper bound for the exact effective rate expression; and (iii) the MoG-based solution outperforms the multivariate Fox’s $H$ -function based approach with reducing complexity.
更多查看译文
关键词
Effective rate, quality-of-service (QoS), cascaded alpha-mu, reconfigurable intelligent surfaces (RIS), Kolmogorov-Smirnov (KS) goodness of fit test, Fox's H-function, mixture of Gaussian (MoG)
AI 理解论文
溯源树
样例
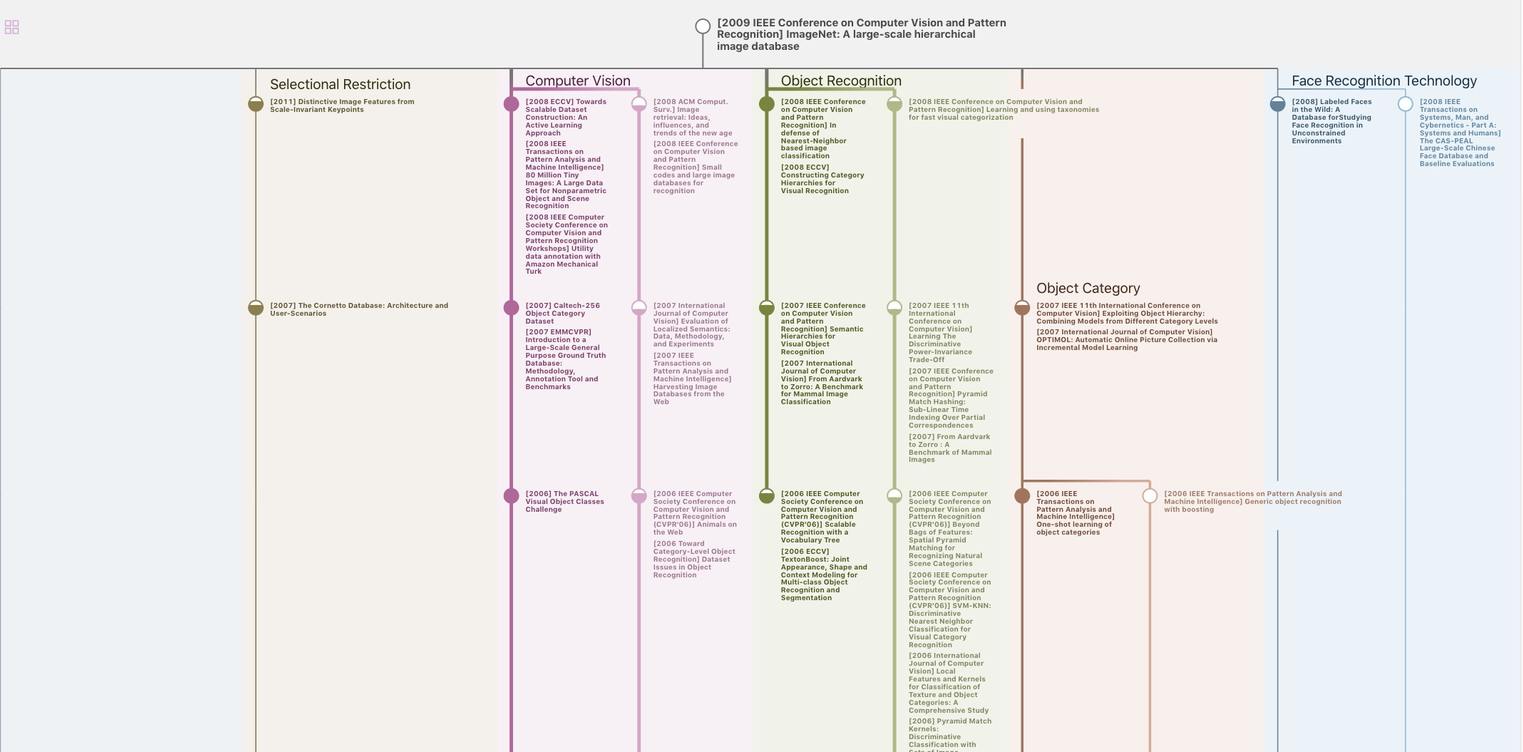
生成溯源树,研究论文发展脉络
Chat Paper
正在生成论文摘要