A Novel Unsupervised Learning Model for Diffeomorphic Image Registration
MEDICAL IMAGING 2021: IMAGE PROCESSING(2021)
摘要
Deformable medical image registration is vital for doctor's diagnosis and quantitative analysis. In this paper, we propose a novel unsupervised learning model (denoted as BSADM) for 3D diffeomorphic medical image registration. Inspired by spatial attention module, we propose a new network architecture BSAU-Net by introducing a novel Binary Spatial Attention Module (BSAM) into skip connection, which can take full advantages of the spatial information extracted from the encoding path and corresponding decoding path. In addition, from variational method in differential geometry, monitor function f is used to control the Jacobian determinant (JD) of registration field phi. So, we also propose a novel orientation-consistent regularization loss to penalize the local regions with negative Jacobian determinant, which further encourages the diffeomorphic property of the transformations. We verify our method on two datasets including ADNI and PPMI dataset, and obtain excellent improvement on magnetic resonance (MR) image registration with higher average Dice scores and better diffeomorphic registration fields (lower non-positive Jacobian locations) compared with other state-of-the-art methods. The experimental results show the method can achieve better performance in brain MR image registration.
更多查看译文
关键词
Medical image registration,Binary Spatial Attention Module,Monitor function,Negative Jacobian determinant,Orientation-consistent regularization loss
AI 理解论文
溯源树
样例
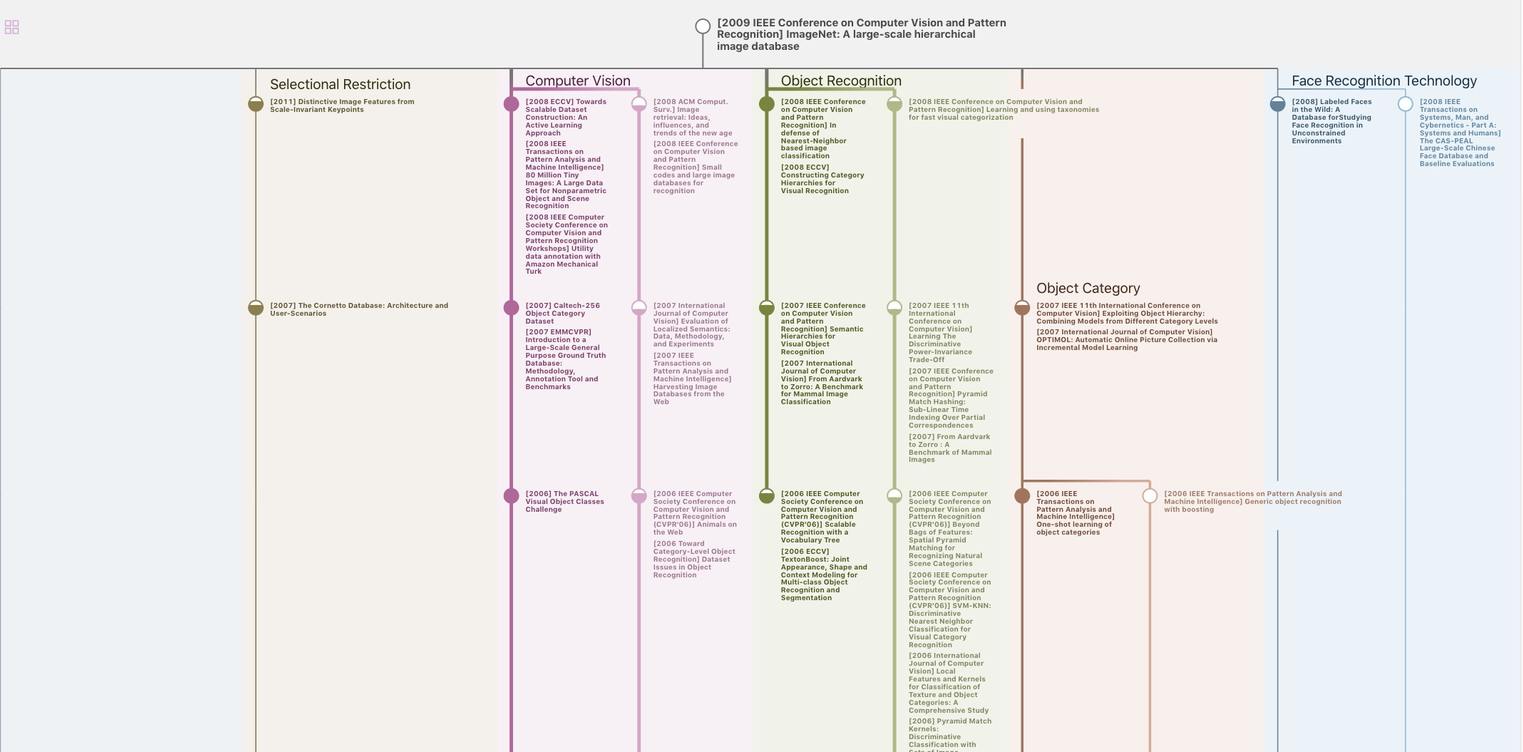
生成溯源树,研究论文发展脉络
Chat Paper
正在生成论文摘要