Dual-Energy Ct Reconstruction Using Deep Mutual-Domain Knowledge For Basis Decomposition And Denoising
MEDICAL IMAGING 2020: PHYSICS OF MEDICAL IMAGING(2021)
摘要
As a quantitative CT imaging technique, the dual-energy CT (DECT) imaging method attracts a lot of research interests. However, material decomposition from high energy (HE) and low energy (LE) data may suffer from magnified noise, resulting in severe degradation of image quality and decomposition accuracy. To overcome these challenges, this study presents a novel DECT material decomposition method based on deep neural network (DNN). In particular, this new DNN integrates the CT image reconstruction task and the nonlinear material decomposition procedures into one single network. This end-to-end network consists of three compartments: the sinogram domain decomposition compartment, the user-defined analytical domain transformation operation (OP) compartment, and the image domain decomposition compartment. By design, both the first and third compartments are responsible for complicated nonlinear material decomposition, while denoising the DECT images. Natural images are used to synthesized the dual-energy data with assumed certain volume fractions and density distributions. By doing so, the burden of collecting clinical DECT data can be significantly reduced, therefore the new DECT reconstruction framework becomes more easy to be implemented. Both numerical and experimental validation results demonstrate that the proposed DNN based DECT reconstruction algorithm can generate high quality basis images with improved accuracy.
更多查看译文
关键词
Dual-energy CT, Material decomposition, Deep neural network
AI 理解论文
溯源树
样例
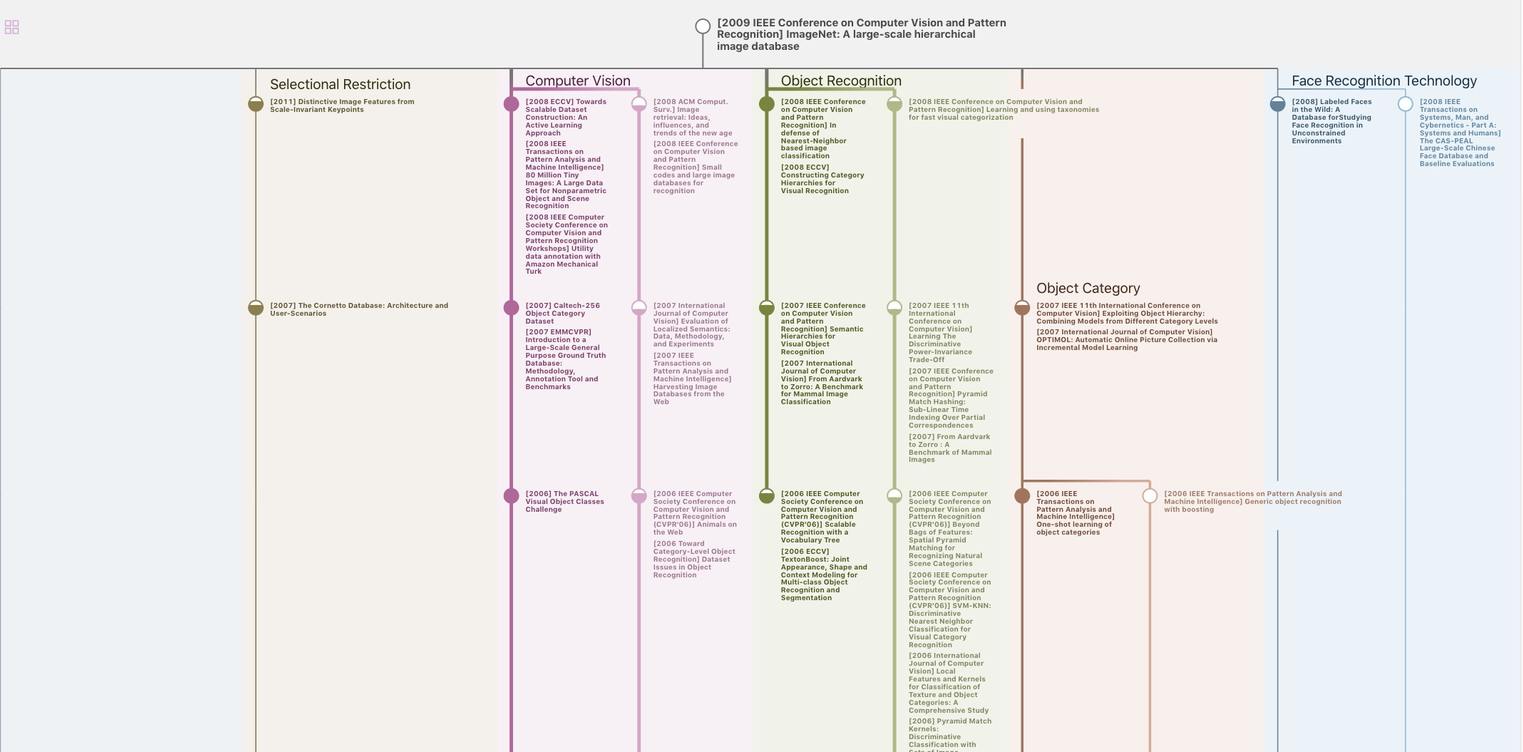
生成溯源树,研究论文发展脉络
Chat Paper
正在生成论文摘要