Generalized Iterative Sparse-View Ct Reconstruction With Deep Neural Network
MEDICAL IMAGING 2020: PHYSICS OF MEDICAL IMAGING(2021)
摘要
Low-dose computed tomography (CT) has attracted much attention in clinical applications since X-ray radiations can cause serious health risks to patients. Sparse-view CT imaging is one of the major ways to reduce radiation dose. However, if reconstructing the sparse-view CT images with conventional filtered backprojection (FBP) algorithm, image quality may be significantly degraded due to the severe streaking artifacts. Therefore, iterative sparse-view CT image reconstruction (IR) algorithms have been developed and utilized to improve image quality. One drawback of using the IR algorithms is they usually spend long computation time. Additionally, adjusting and optimizing the hyper-parameters that are needed during iteration procedures is also time-consuming, sometimes may even depend on individual experience. These potential drawbacks strongly limit the wide applications of IR algorithms. Aiming at partially overcome such difficulties, in the present work, we propose a deep iterative reconstruction (DIR) framework to generalize the conventional IR algorithms by mapping them into the deep neural network (DNN) technique. With this proposed DIR algorithms, the prior term, the data fidelity term, and the hyper-parameters can all be represented and learned by network. By doing so, some generalized iterative models can be used to perform high quality sparse-view CT image reconstructions. Numerical experiments based on clinical patient data demonstrated that the proposed DIR algorithms can mitigate the streaking artifacts more effectively while well preserving the subtle structures.
更多查看译文
关键词
Low-dose CT, Sparse-view CT, iterative reconstruction, deep neural network
AI 理解论文
溯源树
样例
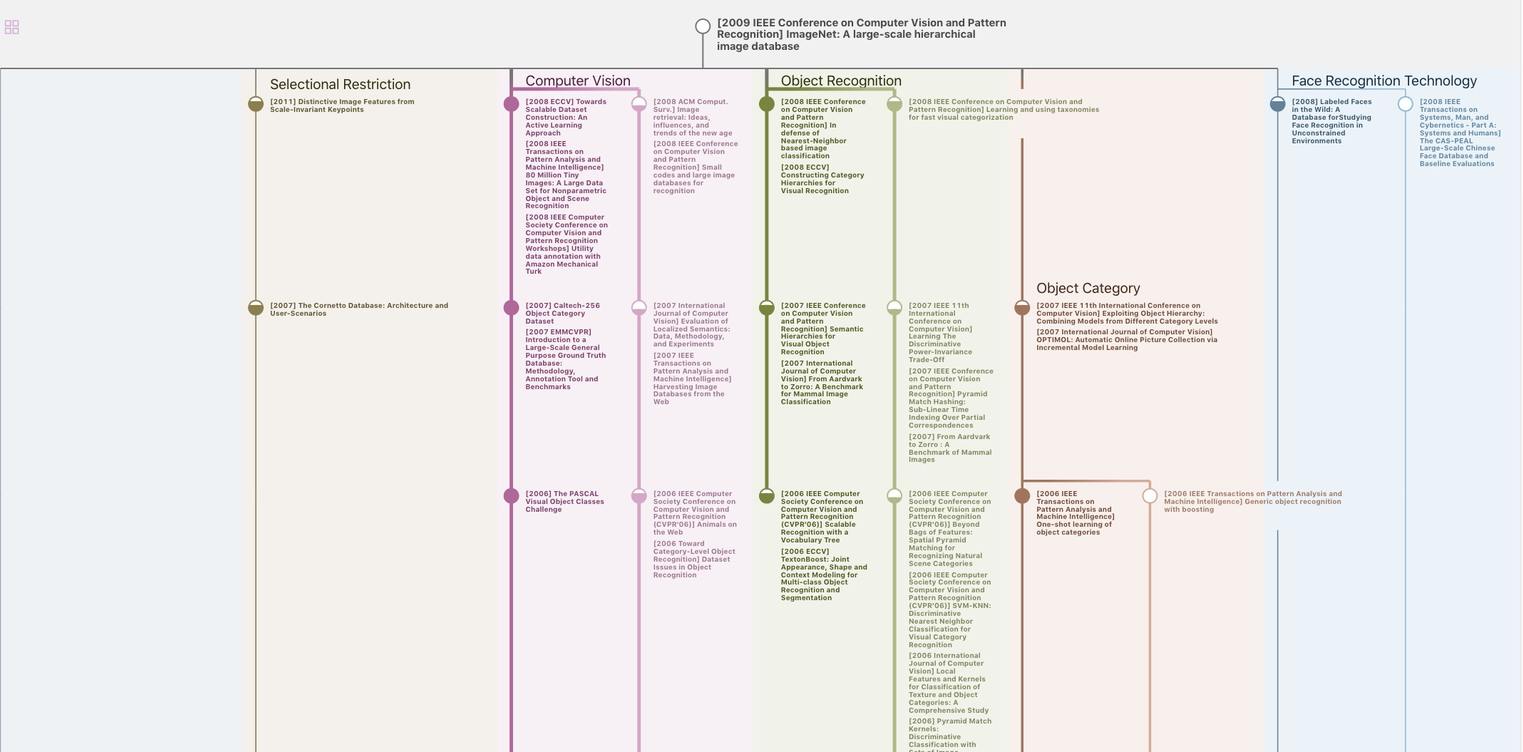
生成溯源树,研究论文发展脉络
Chat Paper
正在生成论文摘要