Reducing The X-Ray Phase Contrast Image Bias Via Deep Computed Signal Estimation Technique
MEDICAL IMAGING 2020: PHYSICS OF MEDICAL IMAGING(2021)
摘要
Studies have shown that the conventionally estimated visibility and differential phase signals in grating-based Talbot-Lau imaging system are intrinsically biased signals. Since such bias are mainly caused by applying the conventional signal estimation approach on noisy data, therefore, it remains an open question whether there has a better signal estimation method to reduce such bias. To answer this question, we proposed an end-to-end supervised deep computed signal estimation network (XP-NET) to extract the three unknown signals, i.e., the absorption, the dark-field, and the phase contrast. Numerical phase stepping data generated from natural images are utilized to train the network. Afterwards, both numerical and experimental studies are performed to validate the performance of the proposed XP-NET method. Results show that for high radiation dose levels, signals retrieved from the XP-NET method are identical as obtained from the conventional analytical method. However, the XP-NET method has the capability of reducing phase signal bias by as much as 15% when the radiation dose levels gets lower. As the phase signal becomes more unbiased, the phase images get more accurate.
更多查看译文
关键词
X-ray phase contrast imaging, Signal estimation bias, Convolutional neural network (CNN)
AI 理解论文
溯源树
样例
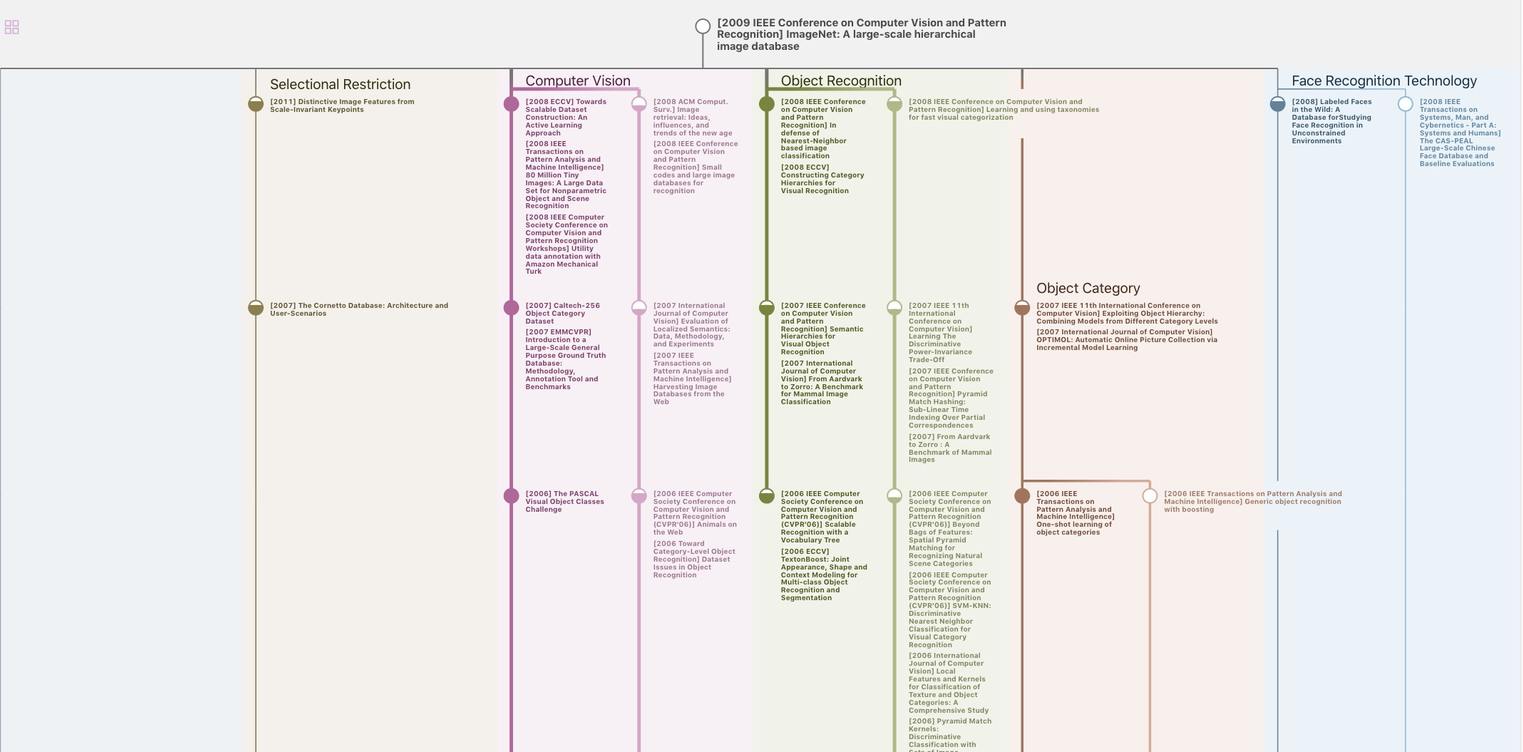
生成溯源树,研究论文发展脉络
Chat Paper
正在生成论文摘要