An Ensemble Approach For Classification Of Accident Narratives
COMPUTING IN CIVIL ENGINEERING 2017: SMART SAFETY, SUSTAINABILITY, AND RESILIENCE(2017)
摘要
There is an increased interest in using text mining techniques to automatically classify text-based accident descriptions in industries such as aviation, medical and construction. The ability to automatically classify accident narratives enables large text databases to be analyzed to provide insights on accidents and near misses. Several machine learning and text mining approaches such as support vector machines (SVM), naive Bayes, and neural networks have been adopted in the literature in order to classify accident narratives. In the recent years, ensemble approach has gained popularity among machine learning applications due to the fact that, ensemble approach combines multiple machine learning algorithms into a much stronger learning algorithm yielding better results. Thus, this study evaluates the effectiveness of an ensemble approach which often performs better than a single learning algorithm, using popular machine learning algorithms: support vector machine, decision tree, linear regression, k nearest neighbor, naive Bayes and neural network. Analyzing the accident narratives reported in the construction safety data yields useful knowledge that can be used to improve the understanding of what went wrong in the past and take necessary precautionary measures to prevent future accidents. One thousand accident narratives obtained from the US OSHA website are used to facilitate this study. Uni-gram tokenization, tf-idf document term matrix representation along with 11 class labels are used to perform the research approach. The precision of ensemble model ranged from 0.6 to 1.0; recall ranged from 0.18 to 0.96, and F1 score was 0.14 to 0.96 across the 11 labels of accident types. The highest average F1 score reported herein was 0.69.
更多查看译文
关键词
Text Mining, Ensemble, Accident classification, Construction Safety
AI 理解论文
溯源树
样例
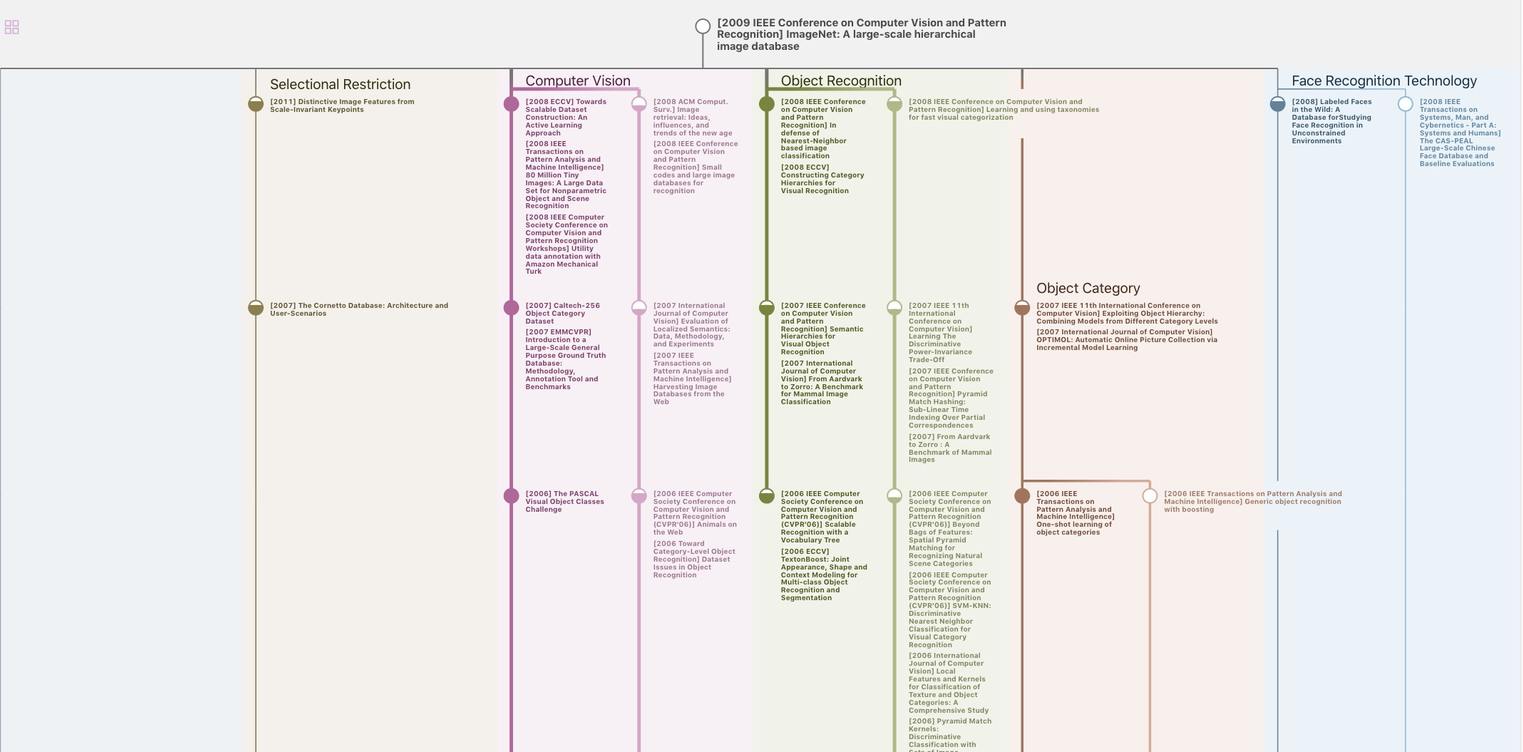
生成溯源树,研究论文发展脉络
Chat Paper
正在生成论文摘要