Near-Real Time Burst Location And Sizing In Water Distribution Systems Using Artificial Neural Networks
WATER(2021)
摘要
The current paper proposes a novel methodology for near-real time burst location and sizing in water distribution systems (WDS) by means of Multi-Layer Perceptron (MLP), a class of artificial neural network (ANN). The proposed methodology can be systematized in four steps: (1) construction of the pipe-burst database, (2) problem formulation and ANN architecture definition, (3) ANN training, testing and sensitivity analyses, (4) application based on collected data. A large database needs to be constructed using 24 h pressure-head data collected or numerically generated at different sensor locations during the pipe burst occurrence. The ANN is trained and tested in a real-life network, in Portugal, using artificial data generated by hydraulic extended period simulations. The trained ANN has demonstrated to successfully locate 60-70% of the burst with an accuracy of 100 m and 98% of the burst with an accuracy of 500 m and to determine burst sizes with uncertainties lower than 2 L/s in 90% of tested cases and lower than 0.2 L/s in 70% of the cases. This approach can be used as a daily management tool of water distribution networks (WDN), as long as the ANN is trained with artificial data generated by an accurate and calibrated WDS hydraulic models and/or with reliable pressure-head data collected at different locations of the WDS during the pipe burst occurrence.
更多查看译文
关键词
burst location, burst quantification, water distribution networks, Artificial Neural Networks
AI 理解论文
溯源树
样例
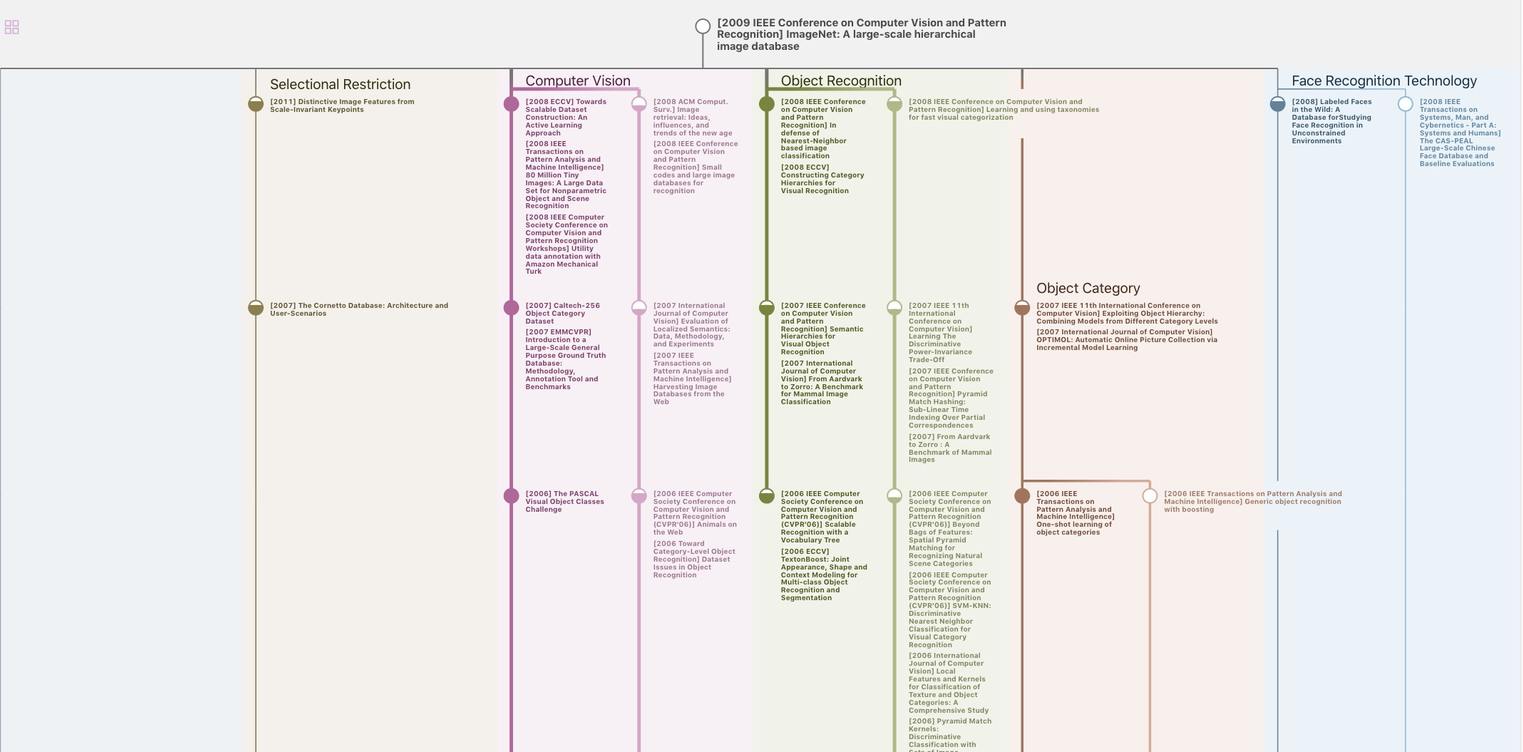
生成溯源树,研究论文发展脉络
Chat Paper
正在生成论文摘要