Coconet: A Hybrid Machine Learning Framework For Coconut Farm Identification And Its Cyclonic Damage Assessment On Bitemporal Sar Images
JOURNAL OF APPLIED REMOTE SENSING(2021)
摘要
The coconut tree is an important cash crop in coastal tropical regions. These trees undergo substantial damage during annual cyclones. Early and effective assessment of damage is important to farmers for appropriate monetary compensation. However, the identification of coconut trees presents multiple challenges: (i) the trees are with other vegetation and (ii) cloud cover during monsoons and cyclones precludes using simple imaging techniques. Although automated approaches based on classical machine learning techniques have been attempted for other crops on remote sensed images, these are not adequate for identifying coconut trees from different land cover. We present a hybrid approach termed CocoNet that combines (i) unsupervised K-means for pixel-based coarse classification of land cover and (ii) a patch- based convolutional neural network for fine classification of vegetation to identify coconut farms. The challenge presented by cloud cover is addressed using synthetic aperture radar (SAR) images. Change detection is then performed on bitemporal Sentinel-1 SAR images taken before and after a cyclone to obtain the change map depicting the damage caused. Experimental results show that the proposed two-phase CocoNet model gives a classification accuracy of 92.5% and a change detection accuracy of 89.1%. (C) 2021 Society of Photo-Optical Instrumentation Engineers (SPIE)
更多查看译文
关键词
synthetic aperture radar, K-means, convolutional neural network, coconut farm identification, change detection
AI 理解论文
溯源树
样例
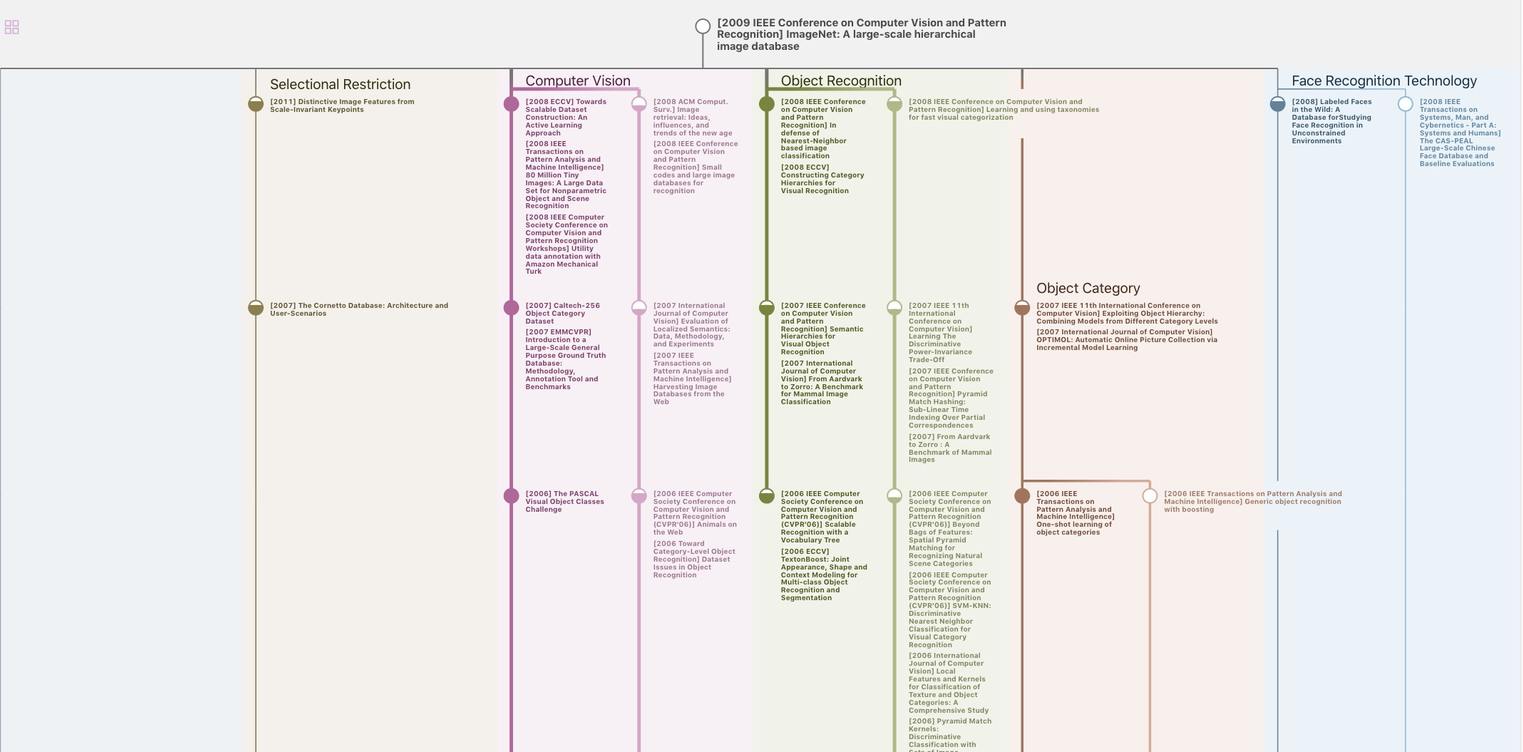
生成溯源树,研究论文发展脉络
Chat Paper
正在生成论文摘要