Adaptive obstacle avoidance in path planning of collaborative robots for dynamic manufacturing
JOURNAL OF INTELLIGENT MANUFACTURING(2021)
摘要
Gaussian Mixture Model (GMM)/Gaussian Mixture Regression (GMR) is a paramount technology of learning from demonstrations to perform human–robotic collaboration. However, GMM/GMR is ineffective in supporting dynamic manufacturing where random obstacles in the applications generate potential safety concerns. In this paper, an improved GMM/GMR-based approach for collaborative robots (cobots) path planning is designed to achieve adaptive obstacle avoidance in dynamic manufacturing. The approach is realised via three innovative steps: (i) new quality assessment criteria for a cobot’s paths produced by GMM/GMR are defined; (ii) based on the criteria, demonstrations and parameters of GMM/GMR are adaptively amended to eliminate collisions and safety issues between a cobot and obstacles; (iii) a fruit fly optimisation algorithm is incorporated into GMM/GMR to expedite the computational efficiency. Case studies with different complexities are used for approach validation in terms of feature retention from demonstrations, regression path smoothness and obstacle avoidance effectiveness. Results of the case studies and benchmarking analyses show that the approach is robust and efficient for dynamic manufacturing applications.
更多查看译文
关键词
Human-robotic collaboration, Obstacle avoidance, Gaussian mixture modelling, Gaussian mixture regression
AI 理解论文
溯源树
样例
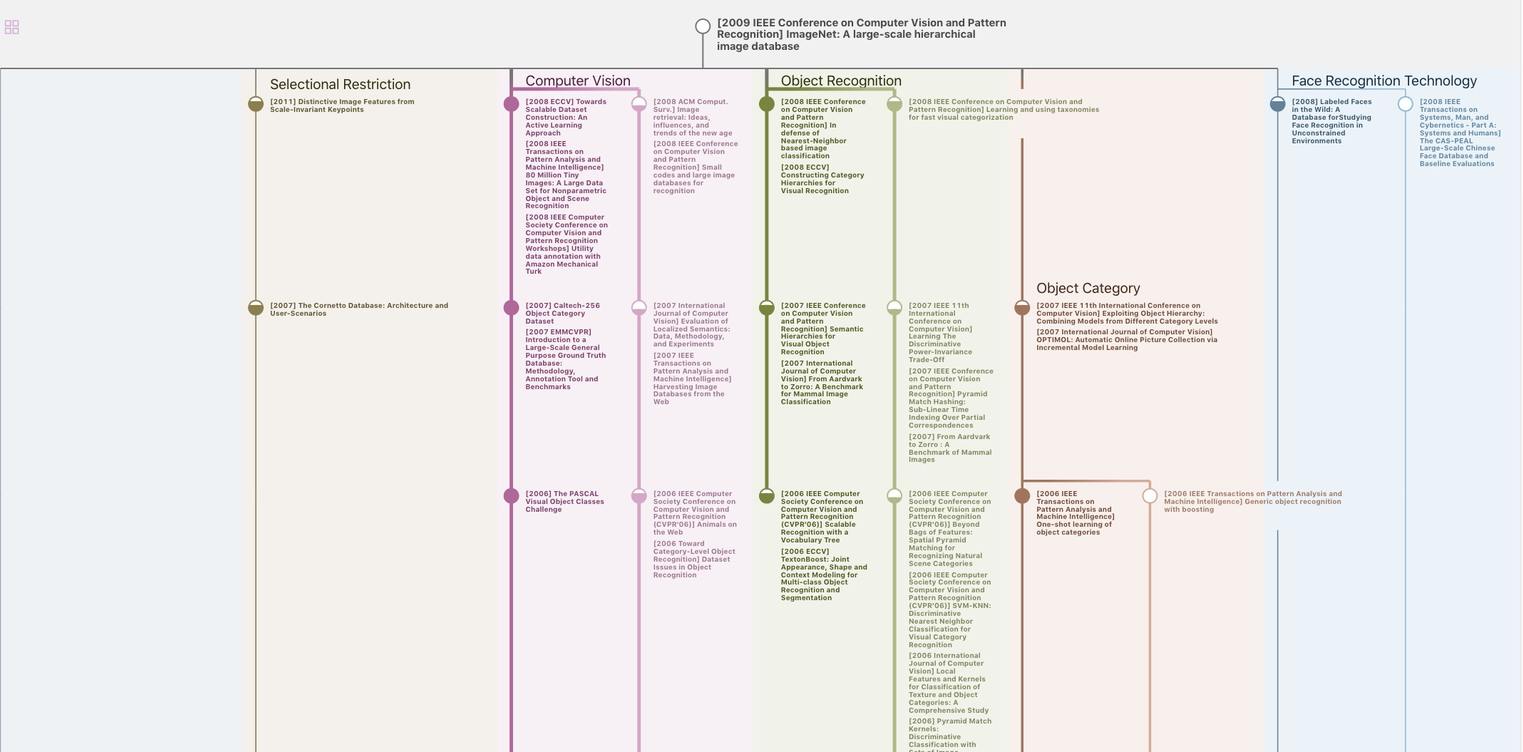
生成溯源树,研究论文发展脉络
Chat Paper
正在生成论文摘要