Limited-Angle Ct Reconstruction Via Data-Driven Deep Neural Network
MEDICAL IMAGING 2021: PHYSICS OF MEDICAL IMAGING(2021)
摘要
An increasing use of computed tomography (CT) in modern medicine has raised radiation dose issues. Strategies for low-dose CT imaging are necessary in order to prevent side effects. Among the strategies, limited-angle CT scans are being used for reducing radiation dose. However, the limited angle scans cause severe artifacts in the images reconstructed by using conventional reconstruction algorithms, such as filtered back-projection (FBP), due to insufficient data. To solve this issue, various methods have been proposed to replace conventional reconstruction algorithms. In this study, we proposed a data-driven deep learning-based limited-angle CT reconstruction method. The proposed method, called Recon-NET, consisted of 3 fully connected (FC) layers, 5 convolution layers and 1 deconvolution layer, and the Recon-NET learned the end-to-end mapping between projection and reconstructed image data. The FBP algorithm was implemented for comparison with the Recon-NET. Also, we evaluated the performance of the Recon-NET in terms of image profile, mean-squared error (MSE), peak signal-to-noise ratio (PSNR) and structural similarity (SSIM). The results showed that the proposed model could reduce artifacts and preserve image details comparing to the conventional reconstruction algorithm. Therefore, the Recon-NET has the potential to provide high-quality CT images with low-dose and reject the complexity of conventional techniques.
更多查看译文
关键词
Deep learning, computed tomography (CT), limited-angle, image reconstruction
AI 理解论文
溯源树
样例
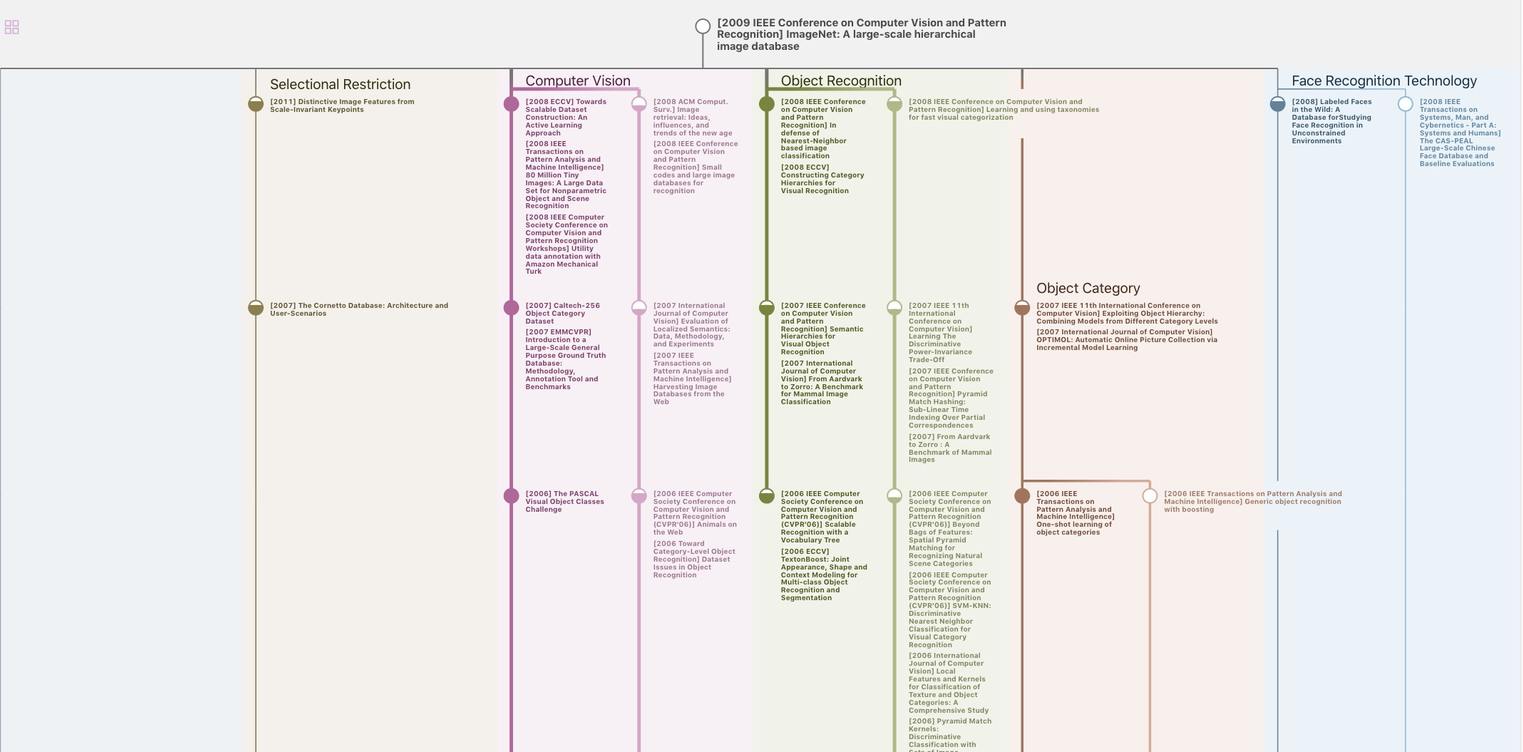
生成溯源树,研究论文发展脉络
Chat Paper
正在生成论文摘要