Classification Of Round Lesions In Dual-Energy Ffdm Using A Convolutional Neural Network: Simulation Study
MEDICAL IMAGING 2021: PHYSICS OF MEDICAL IMAGING(2021)
摘要
The presence of round cystic and solid mass lesions identified at mammogram screenings account for a large number of recalls. These recalls can cause undue patient anxiety and increased healthcare costs. Since cystic masses are nearly always benign, accurate classification of these lesions would be allow a significant reduction in recalls. This classification is very difficult using conventional mammogram screening data, but this study explores the possibility of performing the task on dual-energy full field digital mammography (FFDM) data. Since clinical data of this type is not readily available, realistic simulated data with different sources of variation are used. With this data, a deep convolutional neural network (CNN) was trained and evaluated. It achieved an AUC of 0.980 and 42% specificity at the 99% sensitivity level. These promising results should motivate further development of such imaging systems.
更多查看译文
AI 理解论文
溯源树
样例
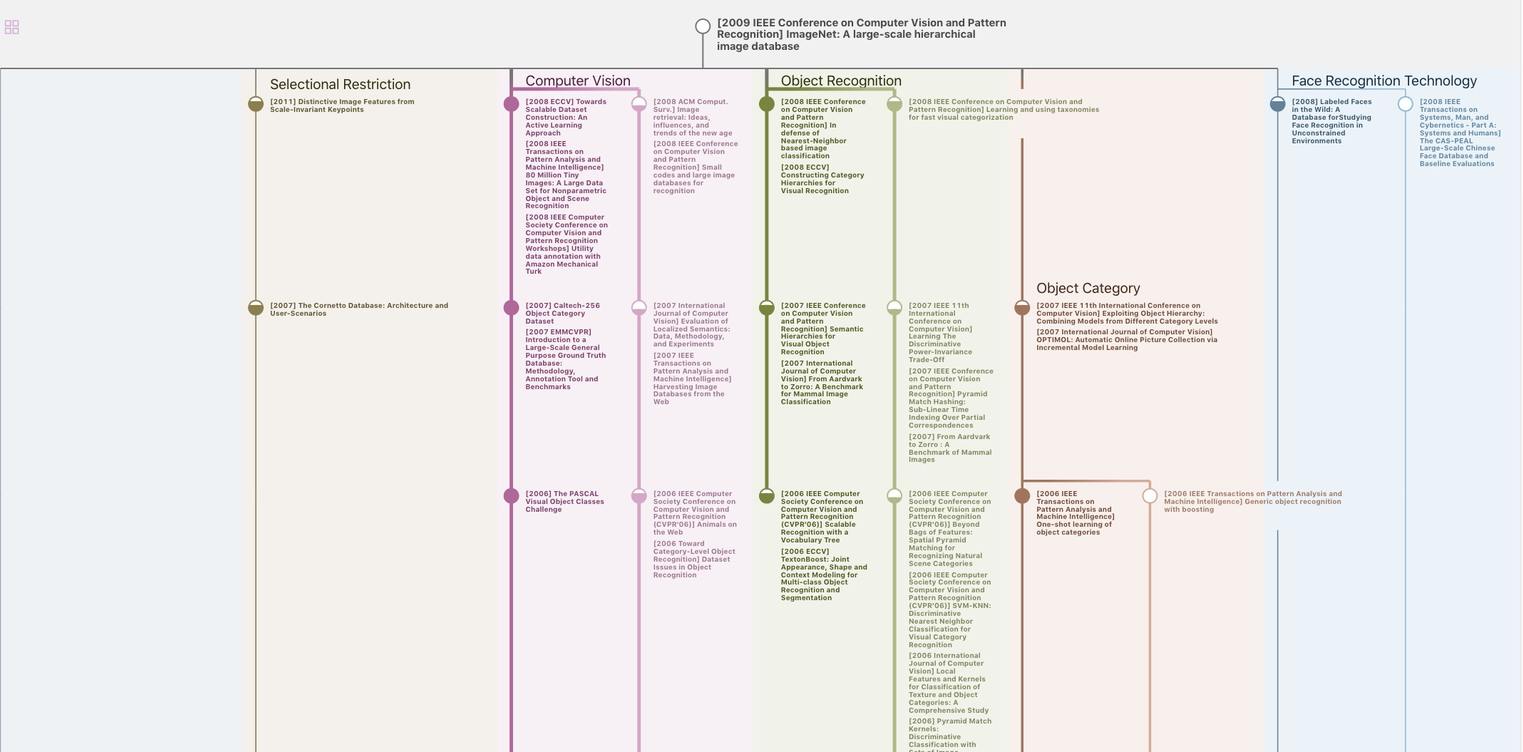
生成溯源树,研究论文发展脉络
Chat Paper
正在生成论文摘要