Business Process Event Prediction Through Scalable Online Learning
IEEE ACCESS(2021)
摘要
Predictive process monitoring techniques aim to forecast outcomes of running business process instances. These techniques are based on using predictive models built from past observed behavior, i.e., in an offline setting. However, business process behavior usually changes over time and predictive models are therefore at risk of becoming obsolete. Because of this, the definition of systems that build predictive models through an online setting has recently gained attention. Nevertheless, the scalability of this kind of setting within a context where the amount of data available is experiencing rapid growth is an outstanding issue. To solve this problem, this paper aims to define a framework for event sequence prediction capable of taking advantage of modern distributed processing platforms. An implementation over this framework based on Apache Flink is presented and it is tested upon two different case studies to prove its validity and its capacity to scale.
更多查看译文
关键词
Predictive models, Proposals, Hidden Markov models, Process monitoring, Data models, Scalability, Real-time systems, Data mining, distributed processing, monitoring, predictive models
AI 理解论文
溯源树
样例
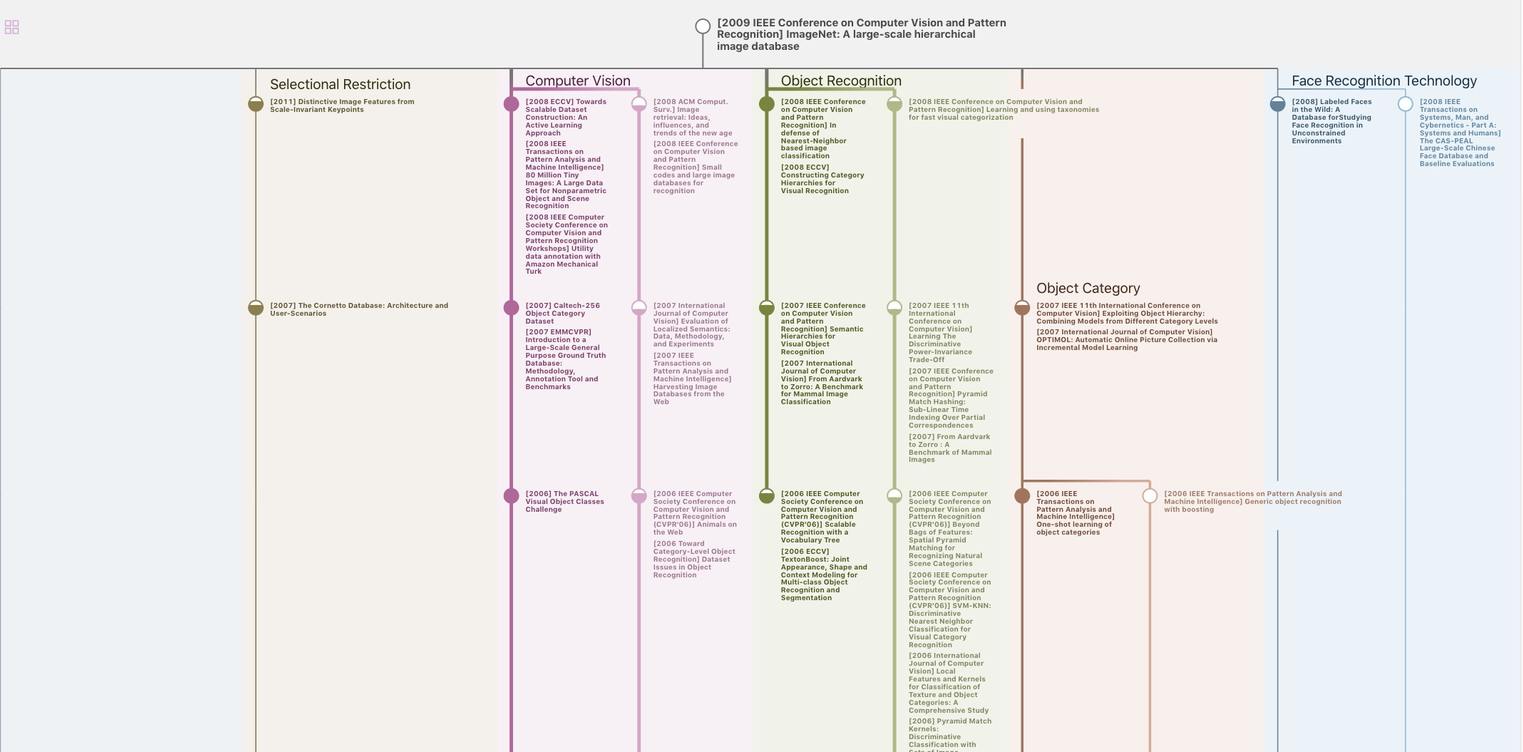
生成溯源树,研究论文发展脉络
Chat Paper
正在生成论文摘要