A One-Step Bayesian Inversion Framework For 3d Reservoir Characterization Based On A Gaussian Mixture Model - A Norwegian Sea Demonstration
GEOPHYSICS(2021)
摘要
We have developed a one-step approach for Bayesian prediction and uncertainty quantification of lithology/fluid classes, petrophysical properties, and elastic attributes conditional on prestack 3D seismic amplitude-variation-with-offset data. A 3D Markov random field prior model is assumed for the lithology/fluid classes to ensure spatially coupled lithology/fluid class predictions in the lateral and vertical directions. Conditional on the lithology/fluid classes, we consider Gauss-linear petrophysical and rock-physics models including depth trends. Then, the marginal prior models for the petrophysical properties and elastic attributes are multivariate Gaussian mixture models. The likelihood model is assumed to be Gauss-linear to allow for analytic computation. A recursive algorithm that translates the Gibbs formulation of the Markov random field into a set of vertical Markov chains is proposed. This algorithm provides a proposal density in a Markov chain Monte Carlo algorithm such that efficient simulation from the posterior model of interest in three dimensions is feasible. The model is demonstrated on real data from a Norwegian Sea gas reservoir. We evaluate the model at the location of a blind well, and we compare results from the proposed model with results from a set of 1D models in which each vertical trace is inverted independently. At the blind well location, we obtain at most a 60% reduction in the root-mean-square error for the proposed 3D model compared to the model without lateral spatial coupling.
更多查看译文
关键词
3d reservoir characterization,bayesian inversion framework,gaussian mixture model,reservoir characterization,one-step
AI 理解论文
溯源树
样例
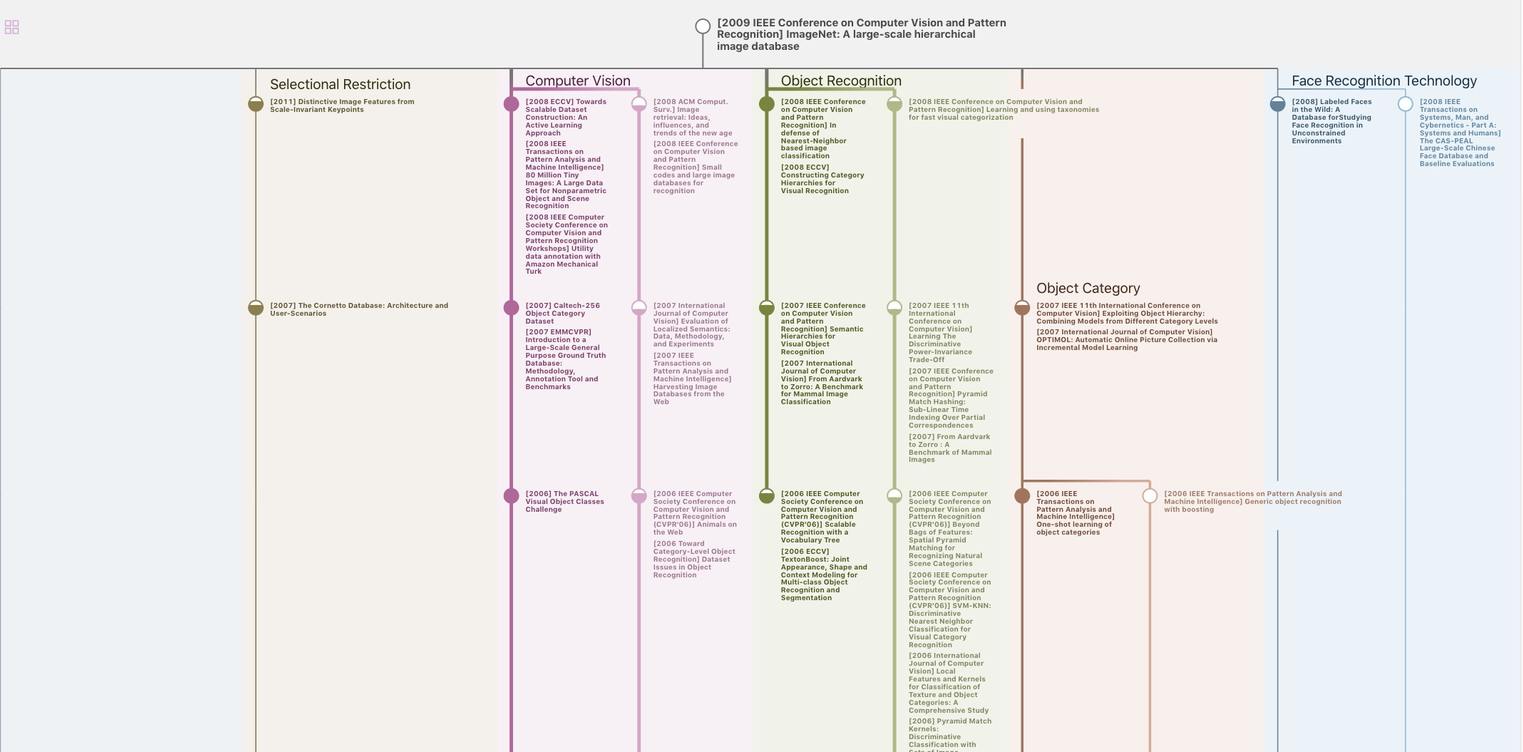
生成溯源树,研究论文发展脉络
Chat Paper
正在生成论文摘要