A Neural N-Gram-Based Classifier For Chinese Clinical Named Entity Recognition
APPLIED SCIENCES-BASEL(2021)
摘要
Clinical Named Entity Recognition (CNER) focuses on locating named entities in electronic medical records (EMRs) and the obtained results play an important role in the development of intelligent biomedical systems. In addition to the research in alphabetic languages, the study of non-alphabetic languages has attracted considerable attention as well. In this paper, a neural model is proposed to address the extraction of entities from EMRs written in Chinese. To avoid erroneous noise being caused by the Chinese word segmentation, we employ the character embeddings as the only feature without extra resources. In our model, concatenated n-gram character embeddings are used to represent the context semantics. The self-attention mechanism is then applied to model long-range dependencies of embeddings. The concatenation of the new representations obtained by the attention module is taken as the input to bidirectional long short-term memory (BiLSTM), followed by a conditional random field (CRF) layer to extract entities. The empirical study is conducted on the CCKS-2017 Shared Task 2 dataset to evaluate our method and the experimental results show that our model outperforms other approaches.
更多查看译文
关键词
clinical named entity recognition, n-gram character embeddings, self-attention, BiLSTM
AI 理解论文
溯源树
样例
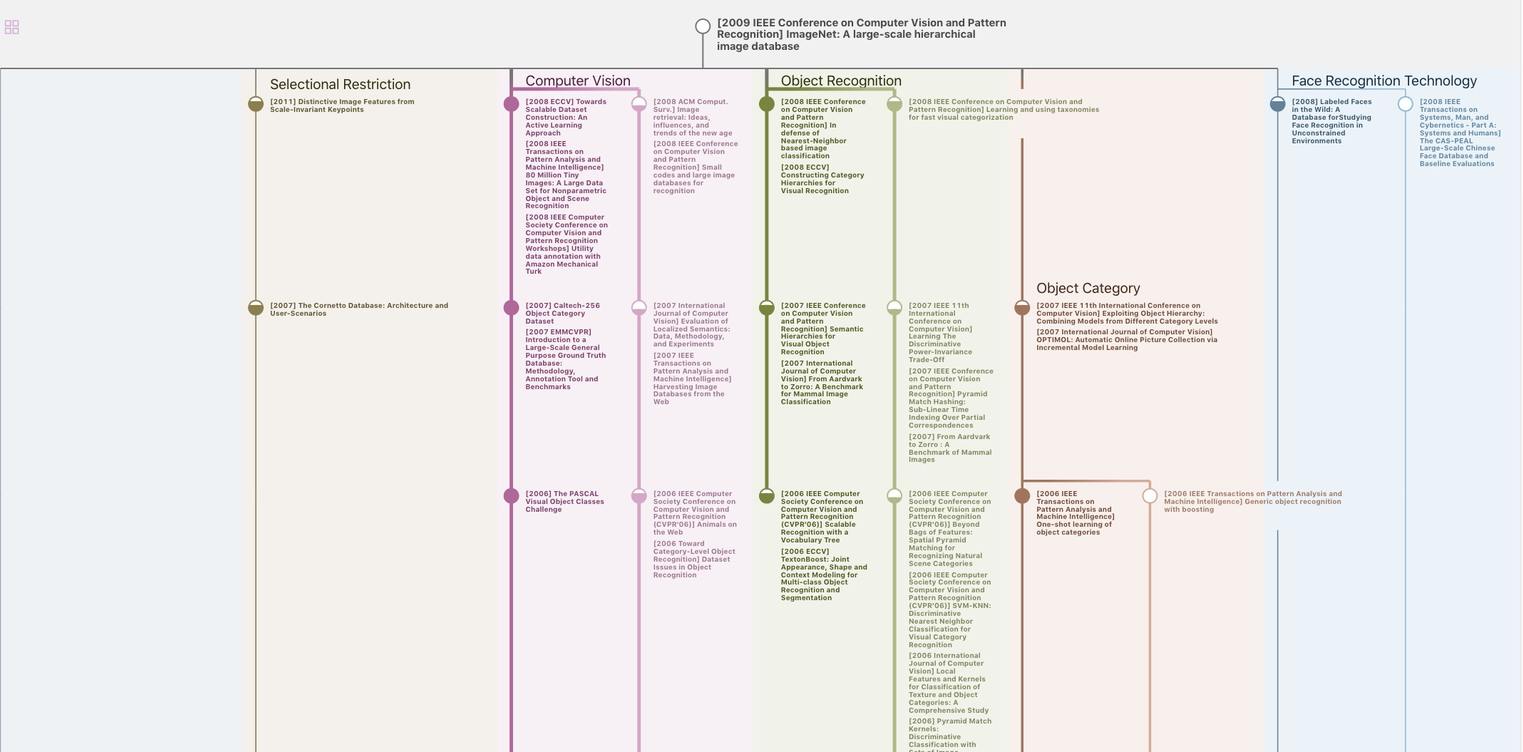
生成溯源树,研究论文发展脉络
Chat Paper
正在生成论文摘要