A Time-Efficient Factorial Hidden Semi-Markov Model For Non-Intrusive Load Monitoring
ELECTRIC POWER SYSTEMS RESEARCH(2021)
摘要
Hidden Markov Model (HMM) and its variations are widely used in Non-Intrusive Load Monitoring (NILM) due to their relatively small requirement for computing resources and label information. Hidden Semi-Markov Model (HSMM), as one of the most representative models, allows the user to characterize the residual time of a hidden state using a specific probability distribution. Yet, because of its time complexity, the algorithm is not practical in case a large number of appliances are present in the dataset. In this paper, a Time-Efficient Factorial Hidden Semi-Markov Model (TE-FHSMM) is presented to improve the computing efficiency, which is more applicable in real world scenarios. Experiments are conducted on two publicly available datasets and one dataset collected from the laboratory to compare the proposed algorithm with six state-of-the-art NILM algorithms. Results show that the proposed TE-FHSMM model could achieve at least 24.5% reduction in time consumption than the classical Factorial Hidden Semi-Markov Model (FHSMM) while maintaining the performance when dealing with a publicly available dataset with different number of appliances. In addition, experiments on a real world scenario and two publicly available datasets demonstrate that the proposed TE-FHSMM model outperforms six stateof-the-art algorithms in terms of Accuracy and F1 score.
更多查看译文
关键词
Load disaggregation, Hidden Markov model, Hidden Semi-Markov model, Computational efficiency
AI 理解论文
溯源树
样例
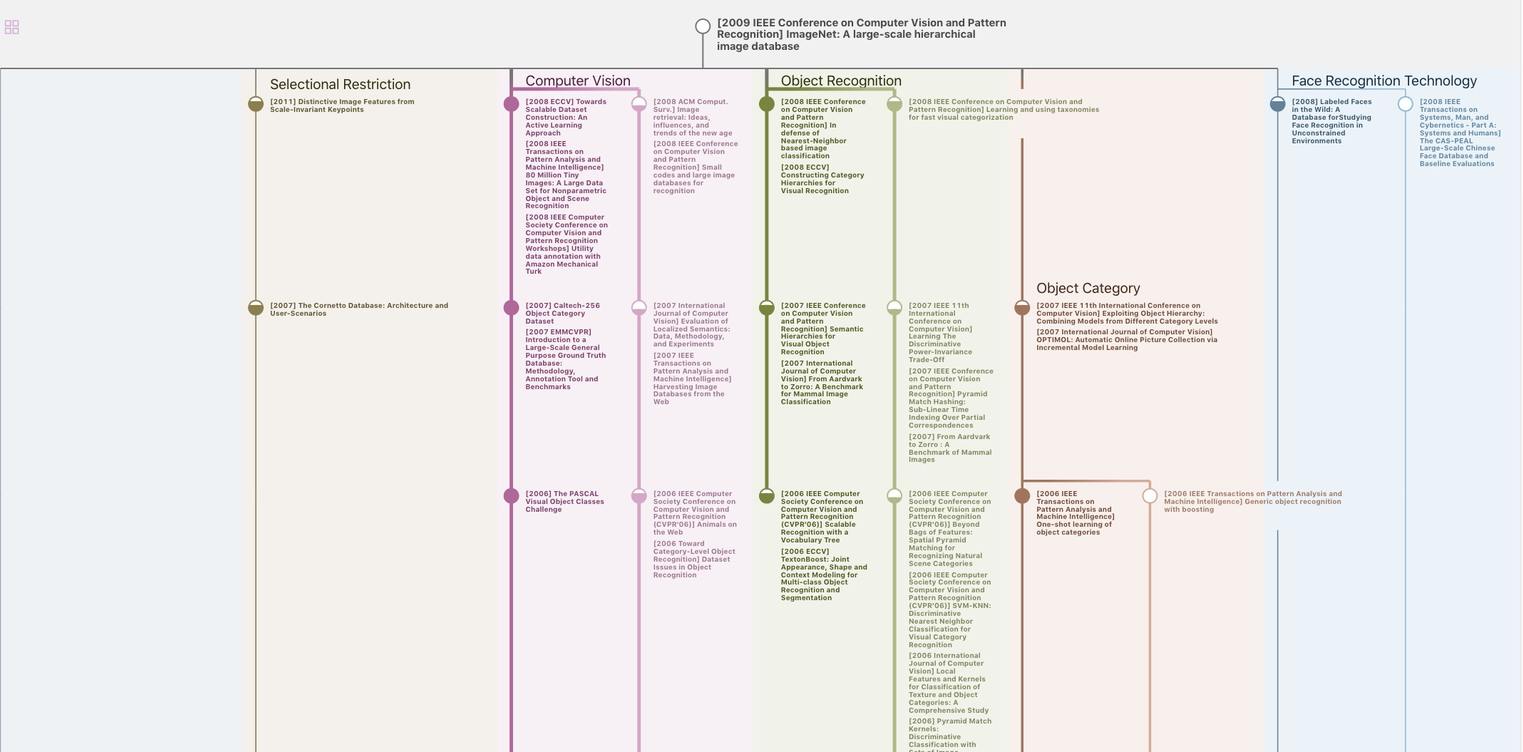
生成溯源树,研究论文发展脉络
Chat Paper
正在生成论文摘要