Reducing Manual Effort To Label Stock Market Data By Applying A Metaheuristic Search: A Case Study From The Saudi Stock Market
IEEE ACCESS(2021)
摘要
Computational intelligence and machine learning techniques have been widely considered for a variety of domains, including financial and data analysis applications. Stock market trading, as a part of the financial domain, has benefited from these techniques in learning models to forecast the direction of stock prices. Traders typically rely on these models, trained using historical stock market data, for monitoring market events to make the right decisions, which can result in more profits while exchanging stocks. One observed limitation of the practices currently being utilized for learning stock market models is the use of manually labeled datasets. This reduces the effectiveness of the data labeling and can cause a lower model prediction accuracy. To address this limitation, this paper proposes an automatic labeling approach that exploits a metaheuristic search to perform the labeling task for stock market data. The results of empirical experiments demonstrate that this approach is very promising as it outperforms the current manual approaches for stock data labeling and achieves higher labeling effectiveness.
更多查看译文
关键词
Labeling, Stock markets, Task analysis, Predictive models, Search problems, Manuals, Simulated annealing, Automatic labeling, machine learning, metaheuristic search algorithms, stock data labeling, stock market trading
AI 理解论文
溯源树
样例
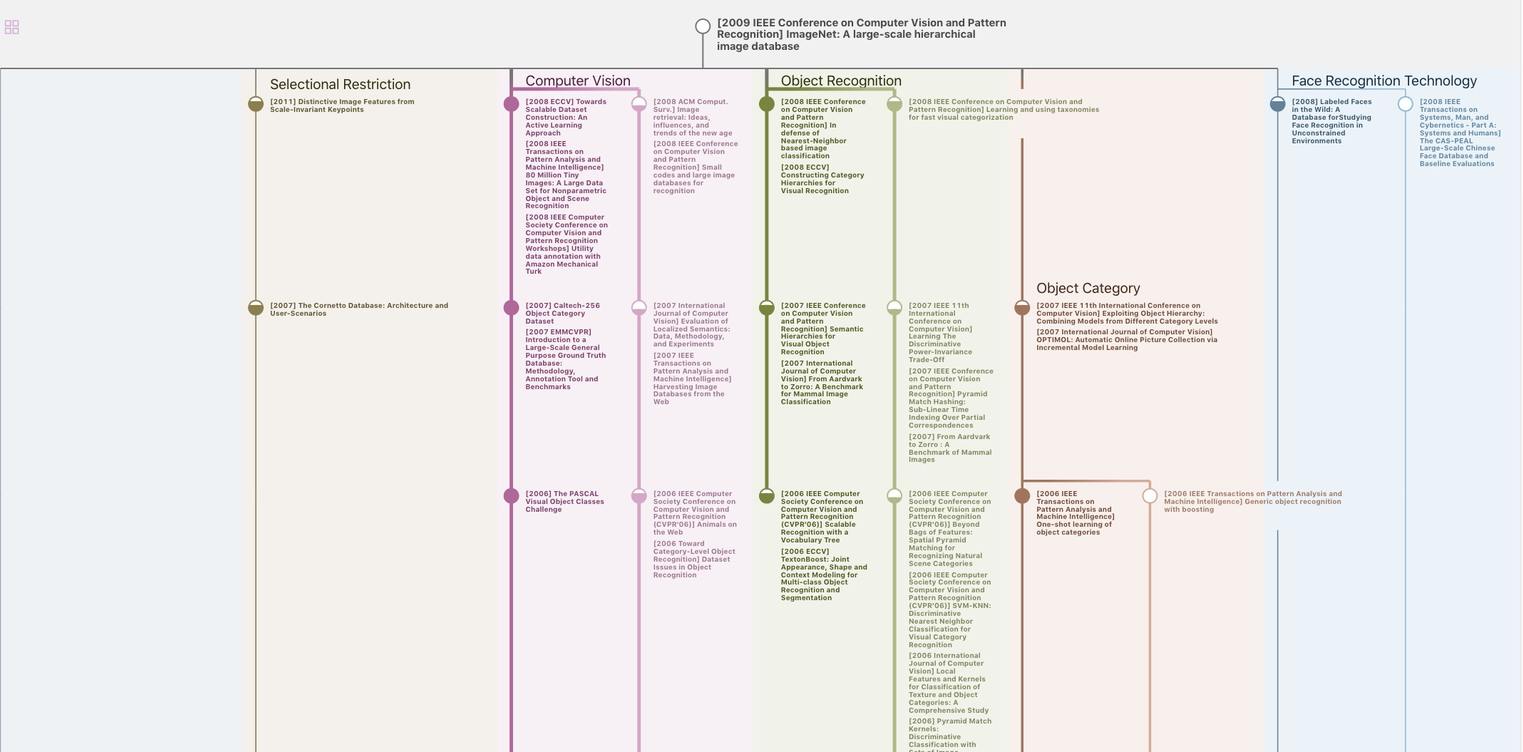
生成溯源树,研究论文发展脉络
Chat Paper
正在生成论文摘要