Application of BPNN optimized by chaotic adaptive gravity search and particle swarm optimization algorithms for fault diagnosis of electrical machine drive system
Electrical Engineering(2021)
摘要
This paper proposes a fault diagnosis method for electrical machine drive system by using backpropagation neural network (BPNN) optimized by chaotic adaptive gravity search algorithm (GSA) and particle swarm optimization (PSO) algorithm. In this method, an adaptive gravitational constant factor based on iteration times and chaotic mapping is introduced to balance the global search ability and local development ability of GSA. Then, it is combined with PSO algorithm to solve the problem of prematurity and local optimum of PSO algorithm. Finally, combined with BPNN, a fault diagnosis model based on chaos adaptive GSA-PSO-BPNN is established. The experimental results show that the introduction of the attenuation factor of adaptive gravitational constant and the chaotic mapping can improve the classification performance of GSA-PSO-BPNN, and the feasibility and effectiveness of the chaotic adaptive GSA-PSO Algorithm are also proved.
更多查看译文
关键词
Backpropagation neural network,Gravity search algorithm,Chaotic mapping,Particle swarm optimization,Fault diagnosis
AI 理解论文
溯源树
样例
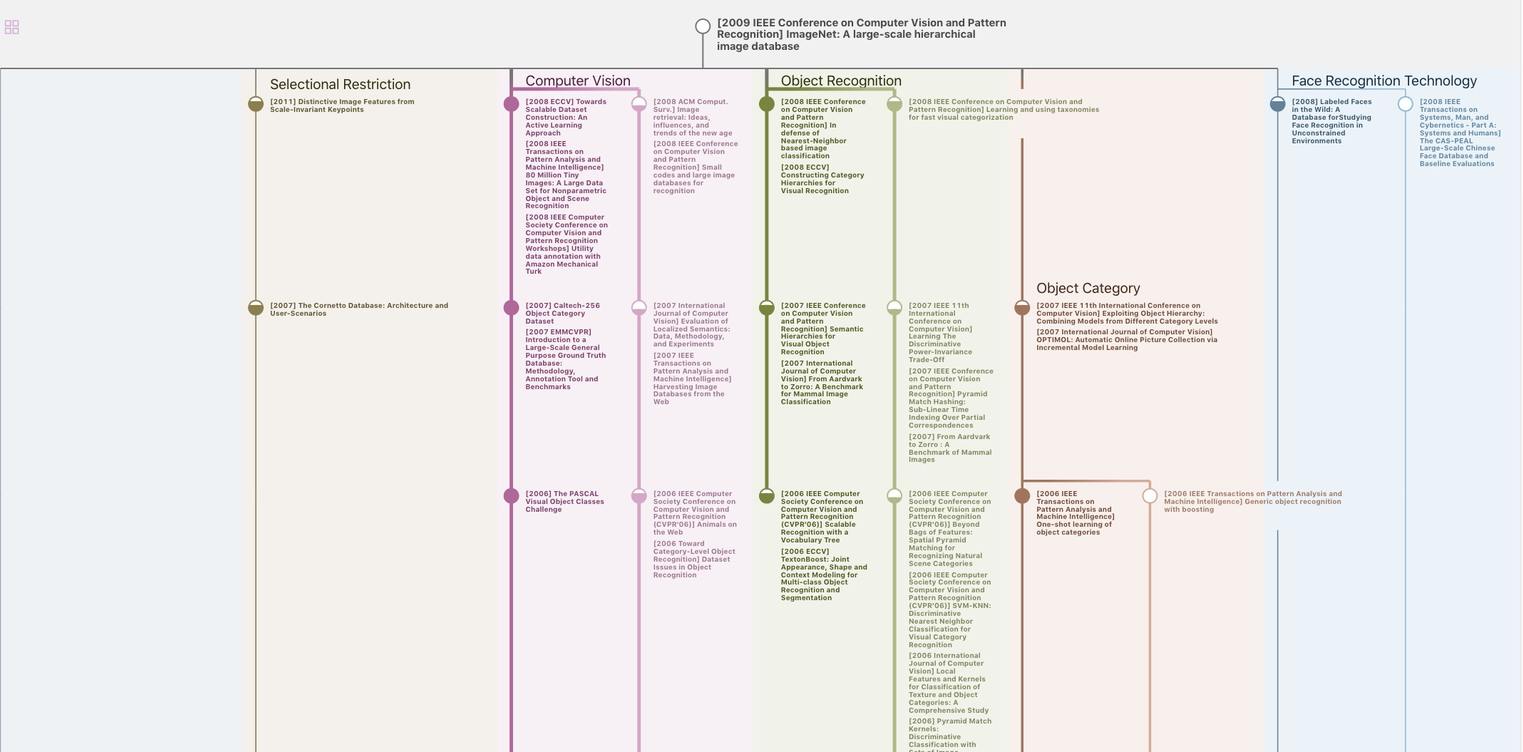
生成溯源树,研究论文发展脉络
Chat Paper
正在生成论文摘要