6d Pose Estimation For Subsea Intervention In Turbid Waters
ELECTRONICS(2021)
摘要
Manipulation tasks on subsea instalments require extremely precise detection and localization of objects of interest. This problem is referred to as "pose estimation ". In this work, we present a framework for detecting and predicting 6DoF pose for relevant objects (fish-tail, gauges, and valves) on a subsea panel under varying water turbidity. A deep learning model that takes 3D vision data as an input is developed, providing a more robust 6D pose estimate. Compared to the 2D vision deep learning model, the proposed method reduces rotation and translation prediction error by (-Delta 0.39 degrees) and translation (-Delta 6.5 mm), respectively, in high turbid waters. The proposed approach is able to provide object detection as well as 6D pose estimation with an average precision of 91%. The 6D pose estimation results show 2.59 degrees and 6.49 cm total average deviation in rotation and translation as compared to the ground truth data on varying unseen turbidity levels. Furthermore, our approach runs at over 16 frames per second and does not require pose refinement steps. Finally, to facilitate the training of such model we also collected and automatically annotated a new underwater 6D pose estimation dataset spanning seven levels of turbidity.
更多查看译文
关键词
subsea, pose estimation, object detection, 3D vision, AUV, ROV, turbidity
AI 理解论文
溯源树
样例
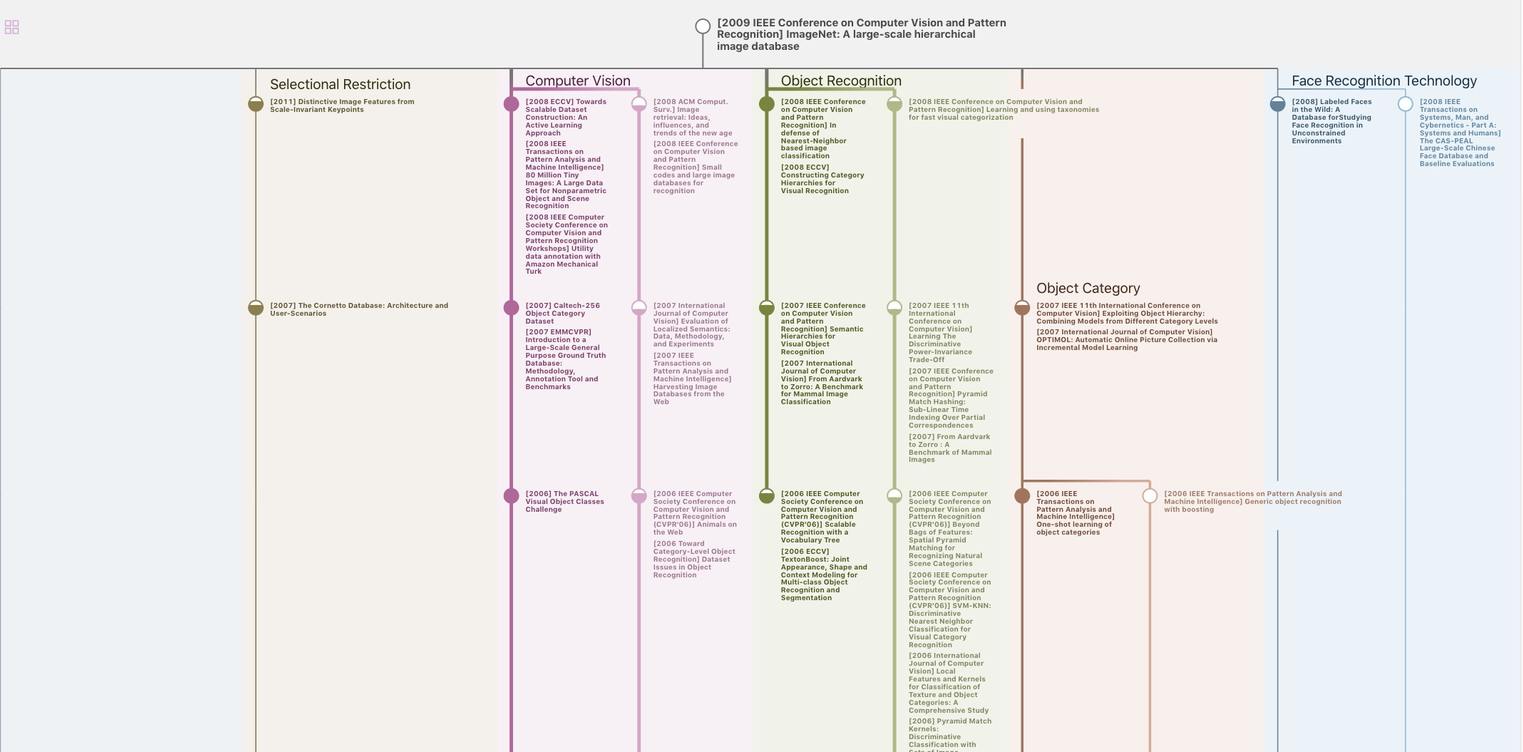
生成溯源树,研究论文发展脉络
Chat Paper
正在生成论文摘要