Training Of Quantized Deep Neural Networks Using A Magnetic Tunnel Junction-Based Synapse
SEMICONDUCTOR SCIENCE AND TECHNOLOGY(2021)
摘要
Quantized neural networks (QNNs) are being actively researched as a solution for the computational complexity and memory intensity of deep neural networks. This has sparked efforts to develop algorithms that support both inference and training with quantized weight and activation values, without sacrificing accuracy. A recent example is the GXNOR framework for stochastic training of ternary and binary neural networks (TNNs and BNNs, respectively). In this paper, we show how magnetic tunnel junction (MTJ) devices can be used to support QNN training. We introduce a novel hardware synapse circuit that uses the MTJ stochastic behaviour to support the quantize update. The proposed circuit enables processing near memory (PNM) of QNN training, which subsequently reduces data movement. We simulated MTJ-based stochastic training of a TNN over the MNIST, SVHN, and CIFAR10 datasets and achieved an accuracy of 98.61% , 93.99% 83.02% , respectively (less than 1% degradation compared to the GXNOR algorithm). We evaluated the synapse array performance potential and showed that the proposed synapse circuit can train TNNs in situ, with 18.3TOPs/W 3TOPs/W for weight update.
更多查看译文
关键词
magnetic tunnel junction, memristor, deep neural networks, quantized neural networks
AI 理解论文
溯源树
样例
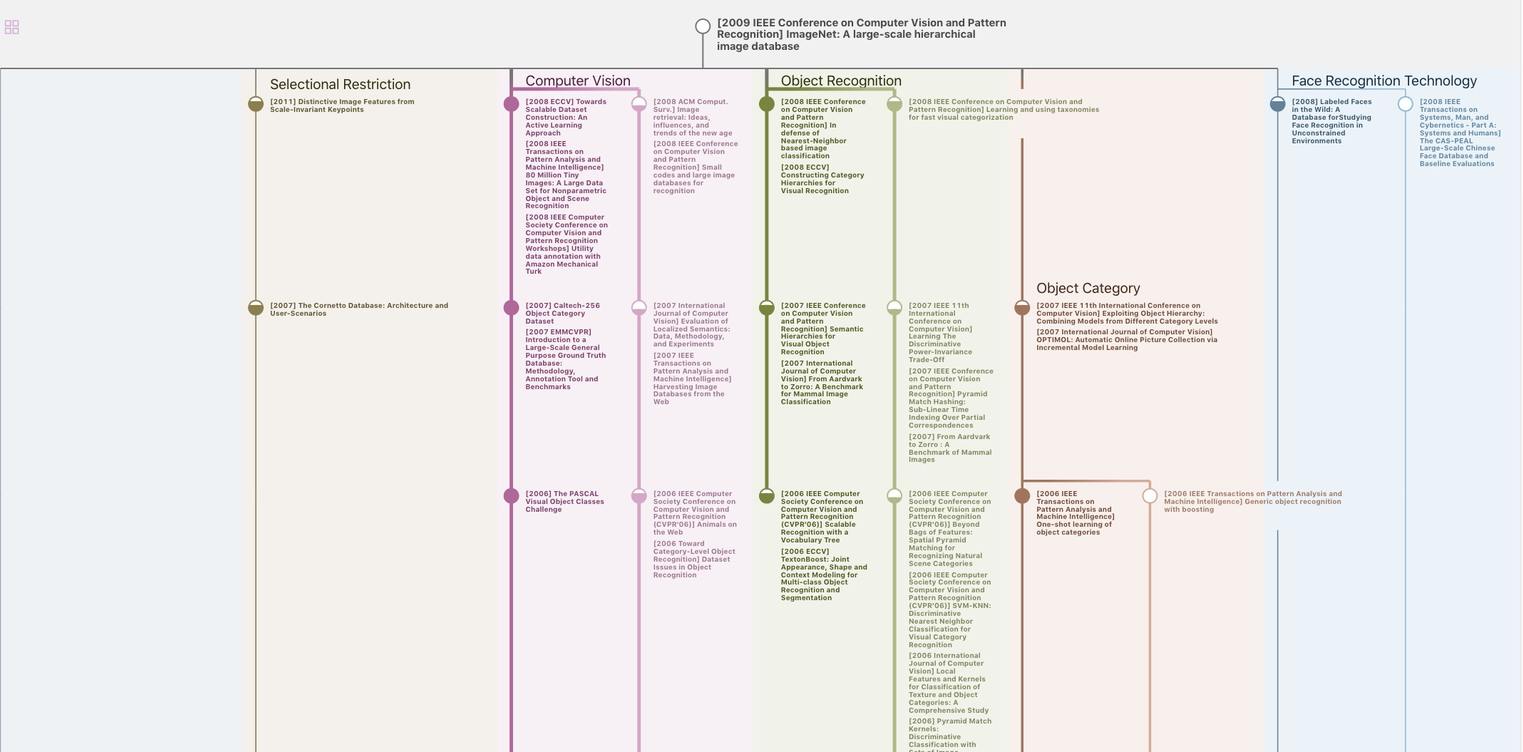
生成溯源树,研究论文发展脉络
Chat Paper
正在生成论文摘要