Deep reinforcement learning based multi-AUVs cooperative decision-making for attack–defense confrontation missions
Ocean Engineering(2021)
摘要
This paper mainly focuses on using deep reinforcement learning (RL) to deal with the cooperative decision-making problem of multiple Autonomous Underwater Vehicles (multi-AUVs) under limited perception and limited communication in attack–defense confrontation missions. Firstly, a novel Coding-Convolutional Network (CCN) is proposed, which can encode the raw sensor information of AUVs and extract representative features from limited perception. Secondly, utilizing the approximator of the state value function and policy function composed of CCN and fully connected network, we put forward a centralized decision-making architecture for multi-AUVs system based on actor–critic framework and give the corresponding algorithm combined with asynchronous training. Moreover, with respect to multi-AUVs attack–defense confrontation tasks, we develop a simulation platform to train and evaluate the proposed algorithms under different parameters. It can be directly observed from the visualization of the simulation that the algorithm enables multi-AUVs to complete the attack–defense confrontation missions excellently and generate some interesting collaborative behaviors. The average winning probability data of the simulation results also indicate that the designed method is feasible for multi-AUVs to achieve cooperative decision-making in attack–defense confrontation missions.
更多查看译文
关键词
Multi-AUVs,Deep reinforcement learning,Cooperative decision-making,Attack–defense confrontation
AI 理解论文
溯源树
样例
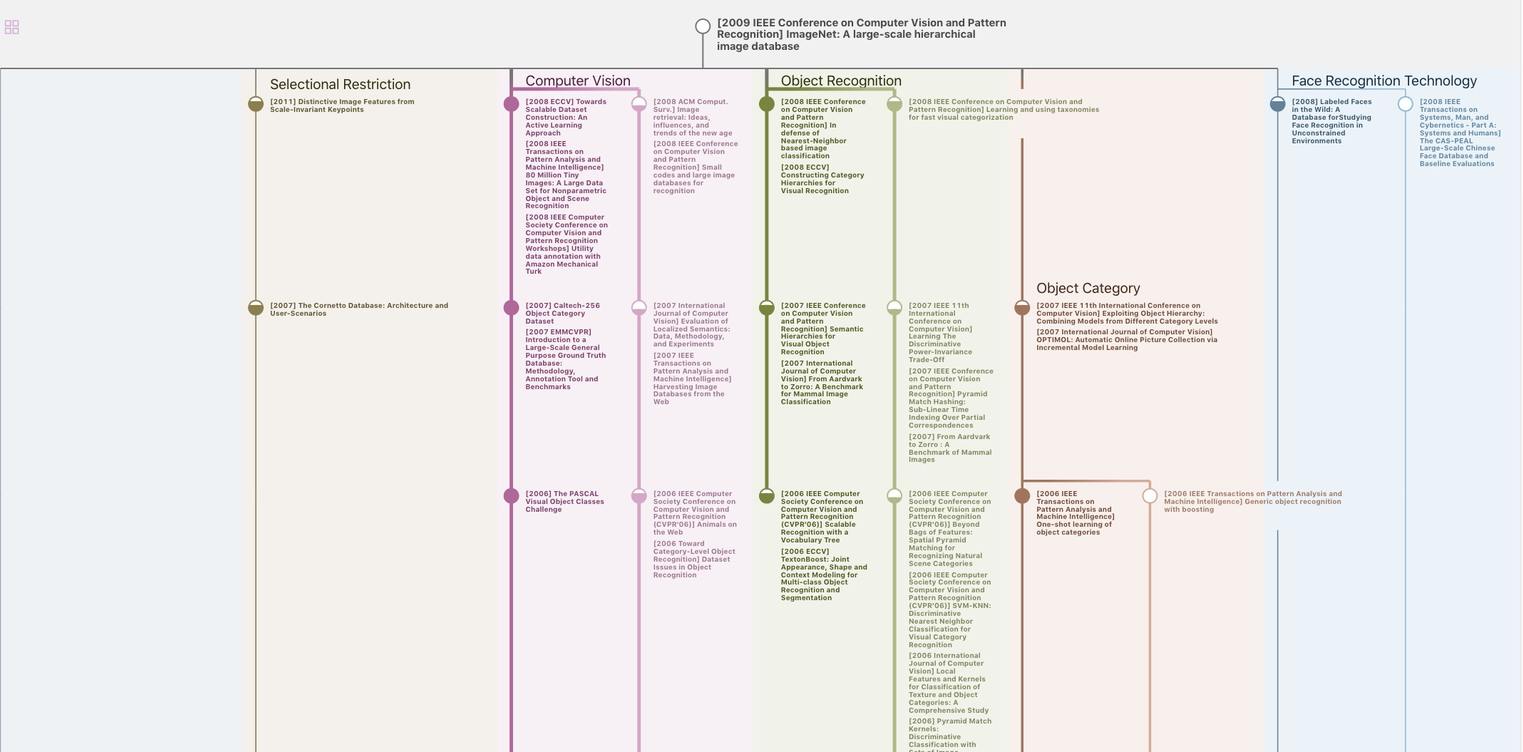
生成溯源树,研究论文发展脉络
Chat Paper
正在生成论文摘要