Variable selection in nonparametric functional concurrent regression
CANADIAN JOURNAL OF STATISTICS-REVUE CANADIENNE DE STATISTIQUE(2022)
摘要
We develop a new method for variable selection in nonparametric functional concurrent regression. The commonly used functional linear concurrent model (FLCM) is far too restrictive in assuming linearity of the covariate effects, which is not necessarily true in many real-world applications. The nonparametric functional concurrent model (NPFCM), on the other hand, is much more flexible and can capture complex dynamic relationships present between the response and the covariates. We extend the classically used variable selection methods, e.g., group LASSO, group SCAD and group MCP, to perform variable selection in NPFCM. We show via numerical simulations that the proposed variable selection method with the non-convex penalties can identify the true functional predictors with minimal false-positive rate and negligible false-negative rate. The proposed method also provides better out-of-sample prediction accuracy compared to the FLCM in the presence of nonlinear effects of the functional predictors. The proposed method's application is demonstrated by identifying the influential predictor variables in two real data studies: a dietary calcium absorption study, and some bike-sharing data.
更多查看译文
关键词
Functional data analysis, nonparametric functional concurrent regression, variable selection
AI 理解论文
溯源树
样例
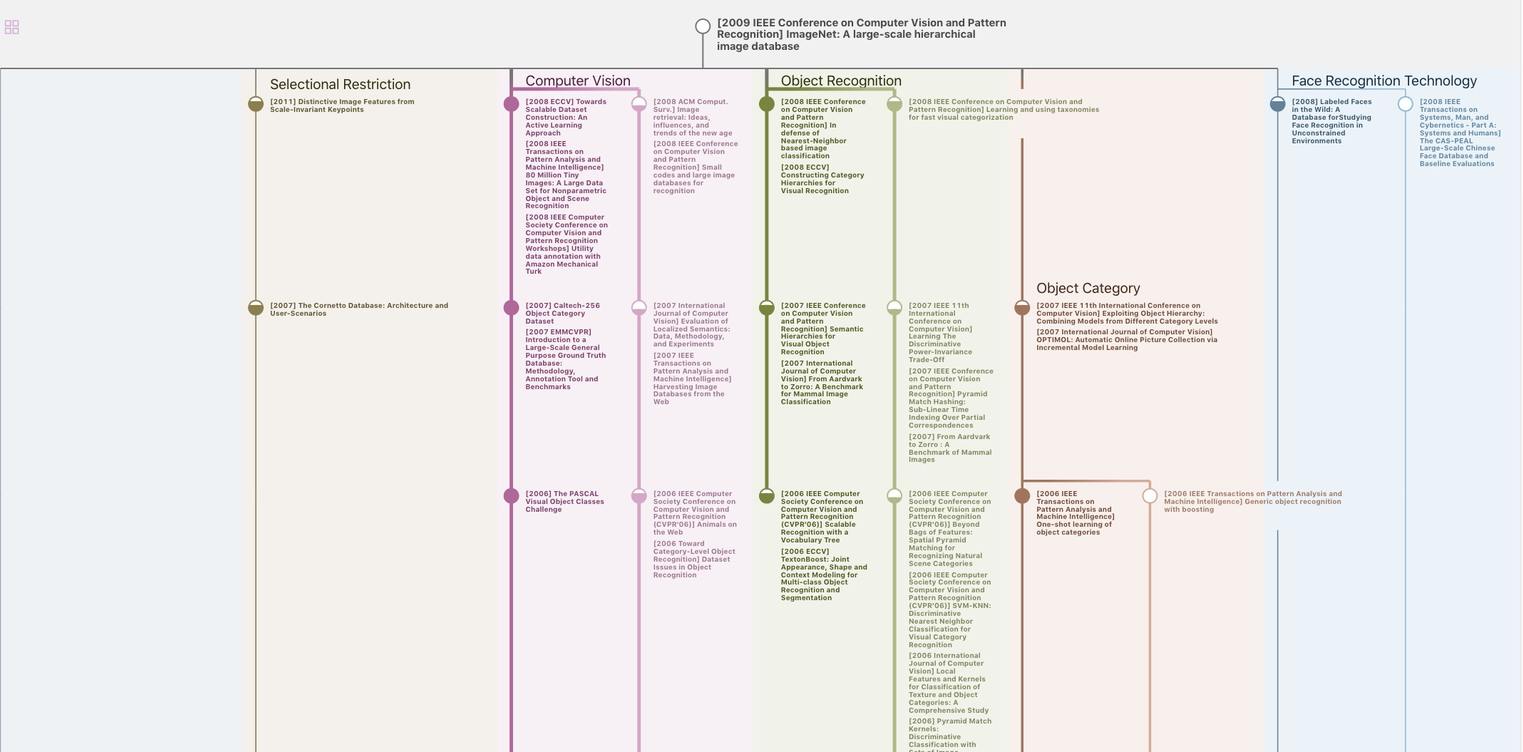
生成溯源树,研究论文发展脉络
Chat Paper
正在生成论文摘要