Jointly modelling multiple transplant outcomes by a competing risk model via functional principal component analysis
JOURNAL OF APPLIED STATISTICS(2023)
摘要
In many clinical studies, longitudinal biomarkers are often used to monitor the progression of a disease. For example, in a kidney transplant study, the glomerular filtration rate (GFR) is used as a longitudinal biomarker to monitor the progression of the kidney function and the patient's state of survival that is characterized by multiple time-to-event outcomes, such as kidney transplant failure and death. It is known that the joint modelling of longitudinal and survival data leads to a more accurate and comprehensive estimation of the covariates' effect. While most joint models use the longitudinal outcome as a covariate for predicting survival, very few models consider the further decomposition of the variation within the longitudinal trajectories and its effect on survival. We develop a joint model that uses functional principal component analysis (FPCA) to extract useful features from the longitudinal trajectories and adopt the competing risk model to handle multiple time-to-event outcomes. The longitudinal trajectories and the multiple time-to-event outcomes are linked via the shared functional features. The application of our model on a real kidney transplant data set reveals the significance of these functional features, and a simulation study is carried out to validate the accurateness of the estimation method.
更多查看译文
关键词
Competing risks,functional principal component analysis,joint model,latent variables,kidney transplant
AI 理解论文
溯源树
样例
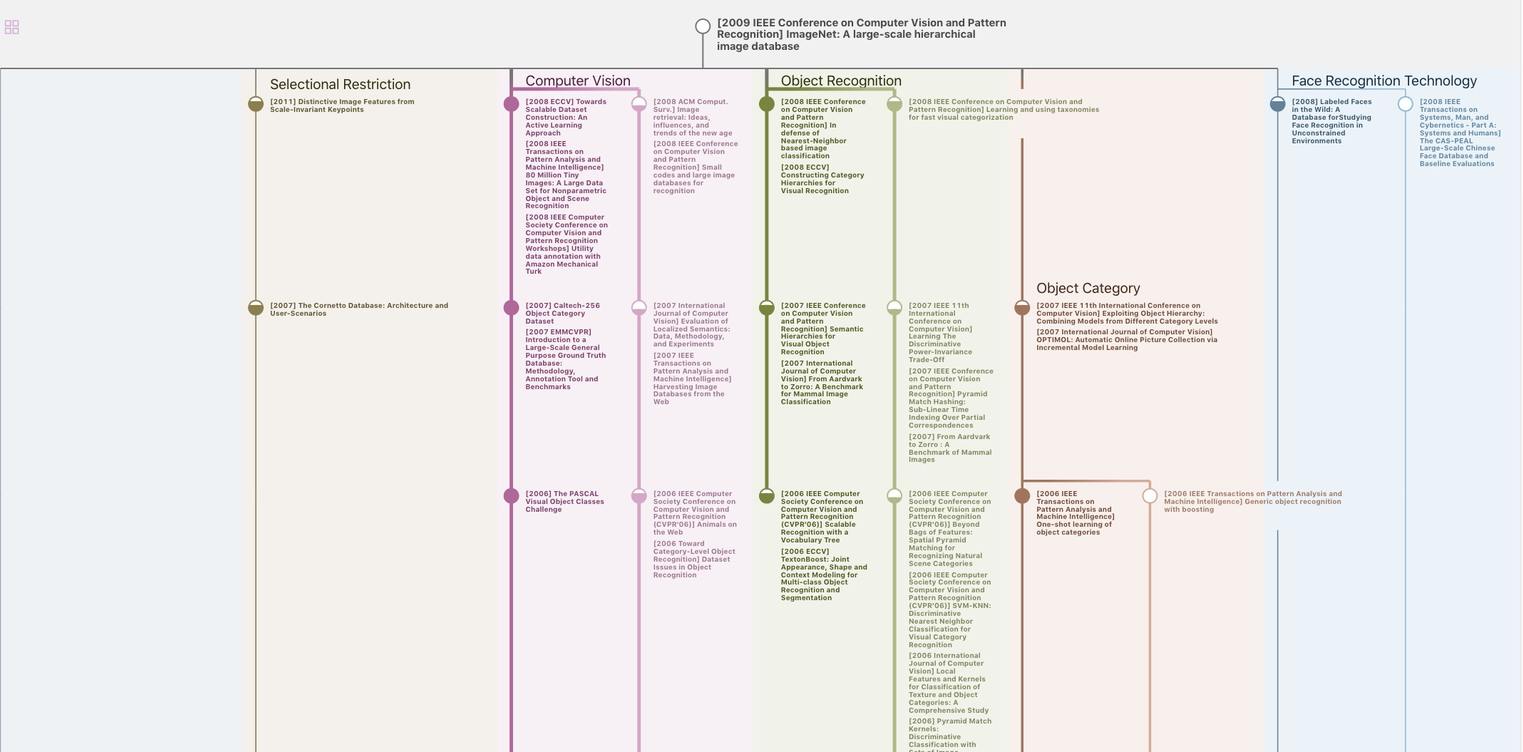
生成溯源树,研究论文发展脉络
Chat Paper
正在生成论文摘要