An improved Girvan–Newman community detection algorithm using trust-based centrality
JOURNAL OF AMBIENT INTELLIGENCE AND HUMANIZED COMPUTING(2021)
摘要
Accumulative structure or cluster-like shape is one of the important features of social networks. These structures and clusters are communities in a complex network and are fully detectable. Common group behaviors of different communities can be categorized using community detection methods. Categorize behavior allows the study of each part of the network to be done centrally. This paper uses trust-based centrality to detect the communities that make up the network. Centrality determines the relative importance of a node in the graph of social networks. Redefining the trust-based centrality makes it possible to change the position in the analysis of centrality and separates the local central nodes and global central nodes. Then, a trust-based algorithm is proposed to express the strength of trust penetration conceptually between nodes to extract communities in networks. This method has led to the achievement of a flexible and effective community detection method. The proposed algorithm is applied to four benchmark networks. The experiments consist of two independent parts. The first part is to use the proposed algorithm to detect clusters and communities. After that, the algorithm is compared with a Girvan–Newman inspired method. The second part is the implementation of the proposed algorithm with a large number of iterations with the aim of modularity maximization and comparing it with other community detection algorithms. Although, the modularity criterion has been used to validate and compare the solution quality in both independent parts of the experiments. The results show about 1.4–5.2% improvement in community detection.
更多查看译文
关键词
Community detection algorithm,Trust-based centrality,Social network,Complex network,Clustering,Girvan–Newman
AI 理解论文
溯源树
样例
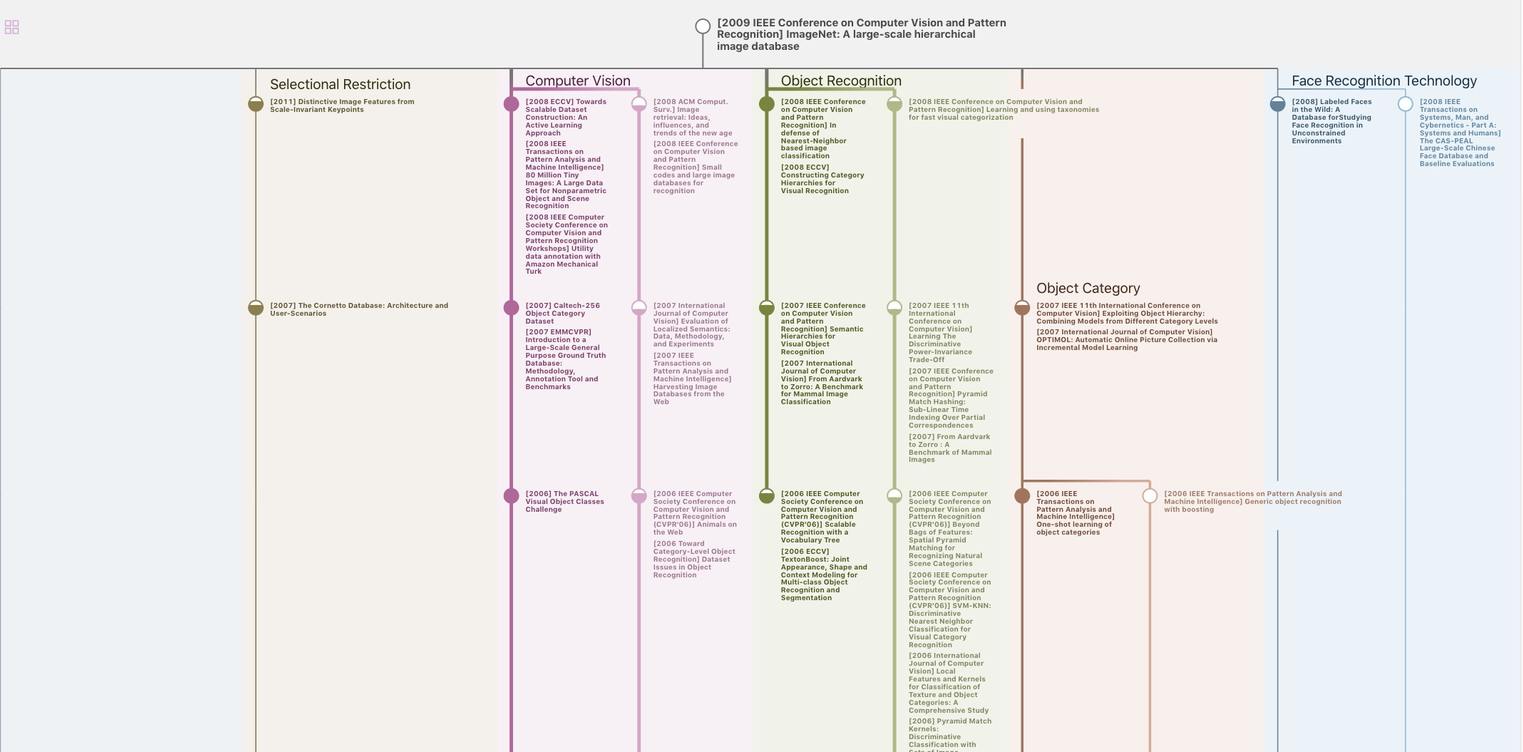
生成溯源树,研究论文发展脉络
Chat Paper
正在生成论文摘要