Classification Of Digital Modulated Covid-19 Images In The Presence Of Channel Noise Using 2d Convolutional Neural Networks
WIRELESS COMMUNICATIONS & MOBILE COMPUTING(2021)
摘要
The wireless environment poses a significant challenge to the propagation of signals. Different effects such as multipath scattering, noise, degradation, distortion, attenuation, and fading affect the distribution of signals adversely. Deep learning techniques can be used to differentiate among different modulated signals for reliable detection in a communication system. This study aims at distinguishing COVID-19 disease images that have been modulated by different digital modulation schemes and are then passed through different noise channels and classified using deep learning models. We proposed a comprehensive evaluation of different 2D Convolutional Neural Network (CNN) architectures for the task of multiclass (24-classes) classification of modulated images in the presence of noise and fading. It is used to differentiate between images modulated through Binary Phase Shift Keying, Quadrature Phase Shift Keying, 16- and 64-Quadrature Amplitude Modulation and passed through Additive White Gaussian Noise, Rayleigh, and Rician channels. We obtained mixed results under different settings such as data augmentation, disharmony between batch normalization (BN), and dropout (DO), as well as lack of BN in the network. In this study, we found that the best performing model is a 2D-CNN model using disharmony between BN and DO techniques trained using 10-fold cross-validation (CV) with a small value of DO before softmax and after every convolution and fully connected layer along with BN layers in the presence of data augmentation, while the least performing model is the 2D-CNN model trained using 5-fold CV without augmentation.
更多查看译文
AI 理解论文
溯源树
样例
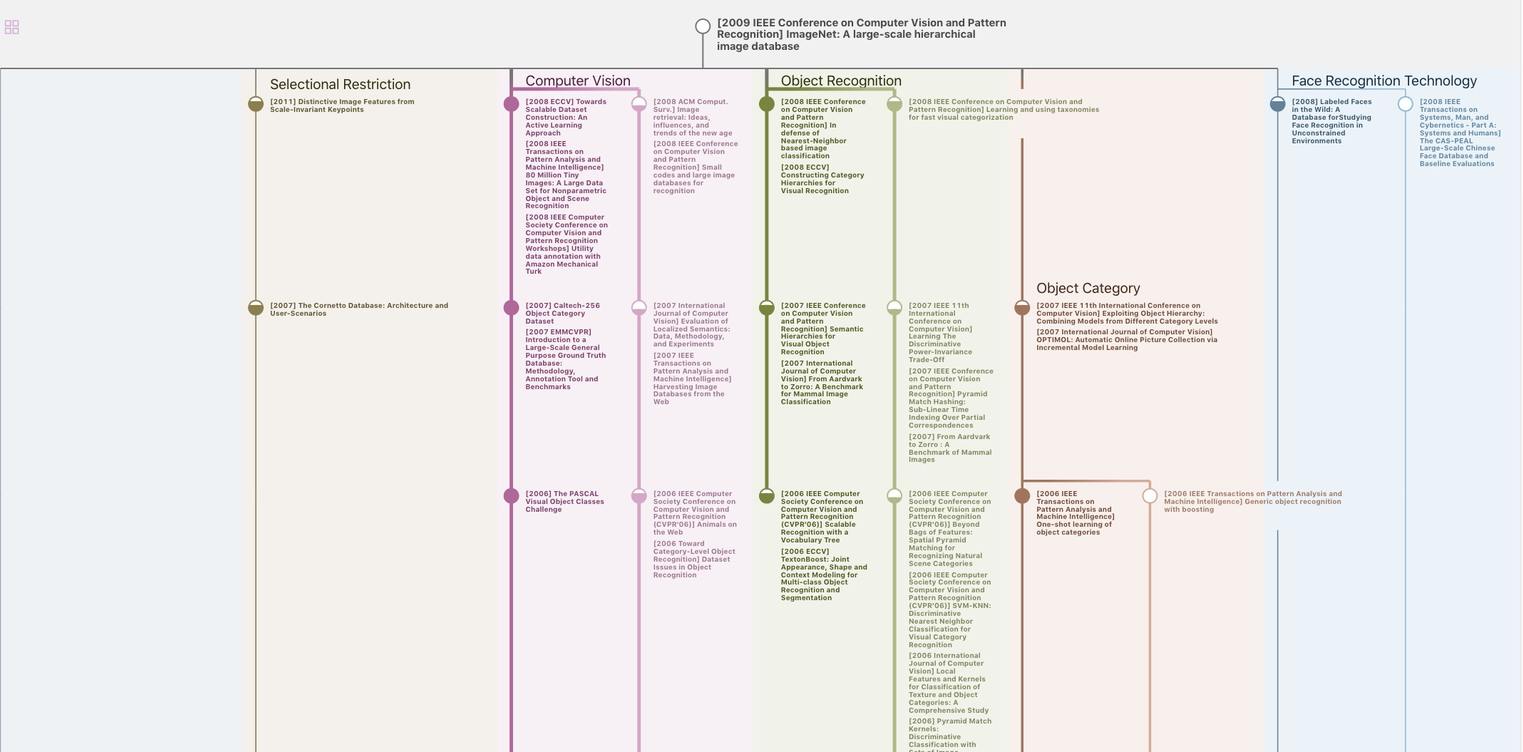
生成溯源树,研究论文发展脉络
Chat Paper
正在生成论文摘要