Dcr: A Unified Framework For Holistic/Partial Person Reid
IEEE TRANSACTIONS ON MULTIMEDIA(2021)
摘要
According to the occlusions, Person reidentification (ReID) can be divided into holistic person ReID and partial person ReID tasks. Occlusions commonly exist in the partial person ReID task but pose or observation perspective changes often occur in the holistic person ReID task; thus, many different network architectures have been designed for each task. However, this approach increases the cost in practice and hinders the development of ReID techniques. To solve this problem, in this work, a unified framework is proposed for holistic/partial person ReID, which can effectively and efficiently address changes in pose or observation perspective and the occlusions in both tasks. In detail, we first employ a fully convolutional network to generate feature maps for an arbitrarily sized image and then use spatial pyramid pooling to obtain its spatial pyramid feature. Thereafter, to efficiently solve the matching problem between the query image and gallery images, we build a deep spatial pyramid feature collaborative reconstruction model (DCR). Experimental results on two partial person ReID datasets and three holistic person ReID datasets demonstrate that DCR outperforms state-of-the-art approaches on both tasks and all datasets. Specifically, it outperforms all competitors with a large margin and achieves an improvement of 9.07% and 5.95% over DSR on Partial ReID and Partial-iLIDS datasets with Rank-1, respectively. Similarly, it also achieves an improvement of 5.08% over VPM on the DukeMTMC-ReID dataset with Rank-1. Additionally, the running time of our method for each query is more than 7x faster than that of DSR or DuATM methods.
更多查看译文
关键词
Task analysis, Cameras, Image reconstruction, Training, Feature extraction, Skeleton, Collaboration, Dcr, deep mutual learning, fcn, holistic person reid, partial person reid, unified framework
AI 理解论文
溯源树
样例
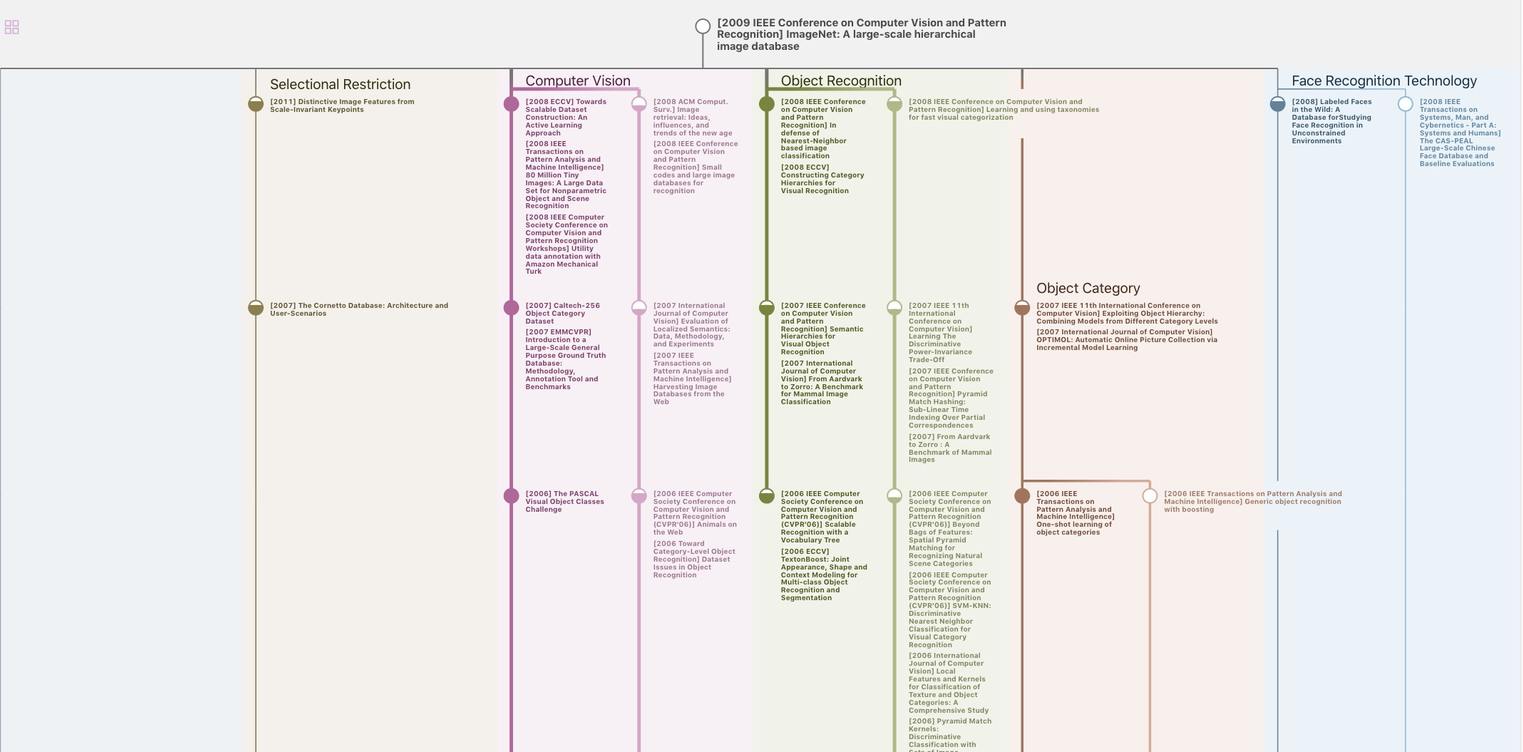
生成溯源树,研究论文发展脉络
Chat Paper
正在生成论文摘要