Multimodal temporal machine learning for Bipolar Disorder and Depression Recognition
Pattern Analysis and Applications(2021)
摘要
Mental disorder is a serious public health concern that affects the life of millions of people throughout the world. Early diagnosis is essential to ensure timely treatment and to improve the well-being of those affected by a mental disorder. In this paper, we present a novel multimodal framework to perform mental disorder recognition from videos. The proposed approach employs a combination of audio, video and textual modalities. Using recurrent neural network architectures, we incorporate the temporal information in the learning process and model the dynamic evolution of the features extracted for each patient. For multimodal fusion, we propose an efficient late fusion strategy based on a simple feed-forward neural network that we call adaptive nonlinear judge classifier . We evaluate the proposed framework on two mental disorder datasets. On both, the experimental results demonstrate that the proposed framework outperforms the state-of-the-art approaches. We also study the importance of each modality for mental disorder recognition and infer interesting conclusions about the temporal nature of each modality. Our findings demonstrate that careful consideration of the temporal evolution of each modality is of crucial importance to accurately perform mental disorder recognition.
更多查看译文
关键词
Multimodal, Mental disorder, Recurrent neural network, Machine learning
AI 理解论文
溯源树
样例
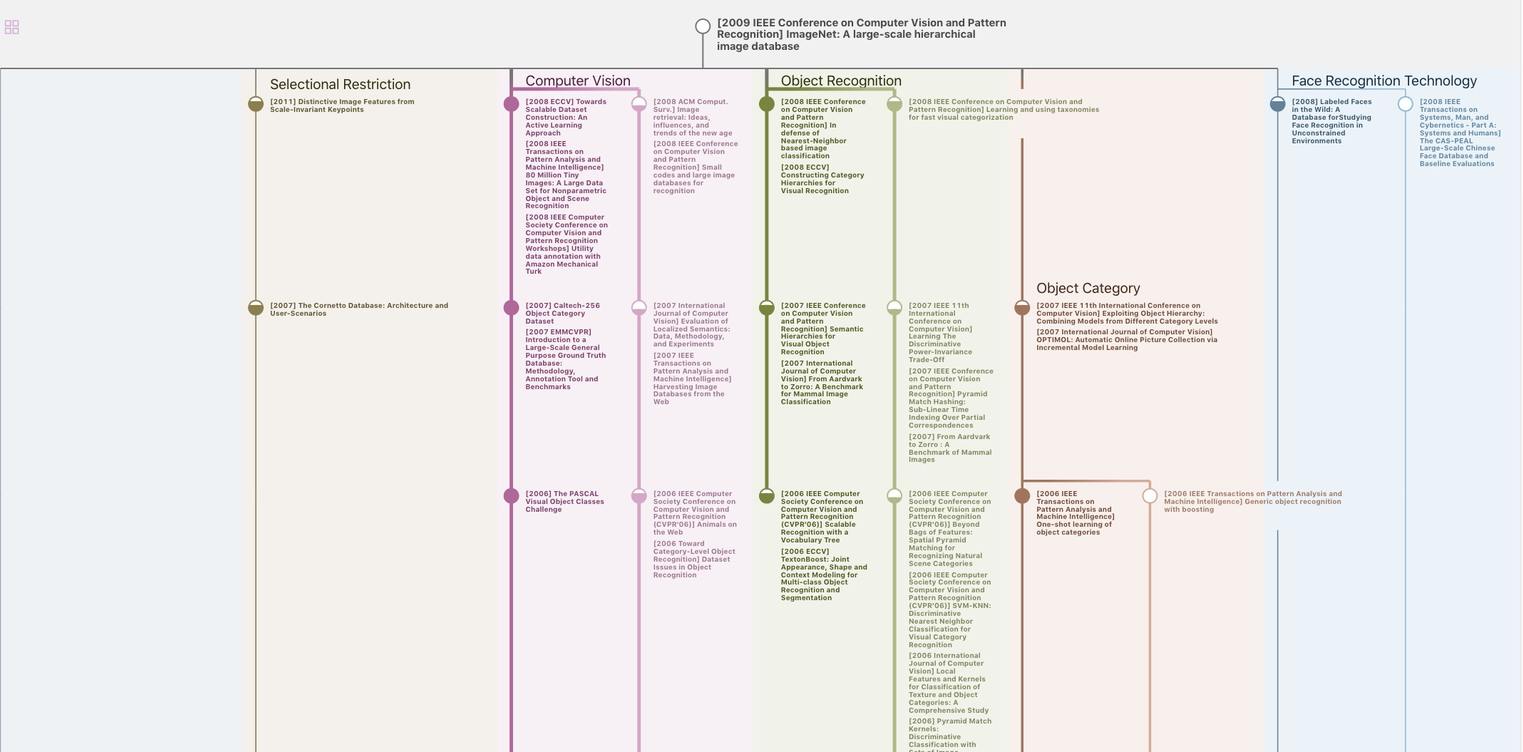
生成溯源树,研究论文发展脉络
Chat Paper
正在生成论文摘要