Electricity Load Forecast Considering Search Engine Indices
ELECTRIC POWER SYSTEMS RESEARCH(2021)
摘要
Accurate electricity load forecast plays an important role in the operation of a power system. Many factors influence the electricity load data such as air temperature, humidity and holidays, and they are taken as the explanatory variables in load forecasting cases traditionally. Search engine indices, a variable may be related to load data which has been never considered before in load forecasting, is discussed and utilized to increase the accuracy of power load prediction in this paper. Spearman's correlation coefficients and Granger test results verify the correlation between Google Trends (GT) and electricity load data. A methodology for processing GT time series with Hodrick-Prescott filter is proposed. To forecast electricity load with an adaptive network model in such a novel situation, we propose a long short-term memory neural network model based on quantum particle swarm algorithm. The performance of load forecast for Long Island region taking GT and weather data as input variables is compared with that taking only weather data as input variables, which shows that the introduction of GT improves short-term forecasting effectiveness significantly.
更多查看译文
关键词
Electricity load forecasting, Time series, Hodrick-Prescott Filter, Long short-term memory network, Quantum particle swarm optimization
AI 理解论文
溯源树
样例
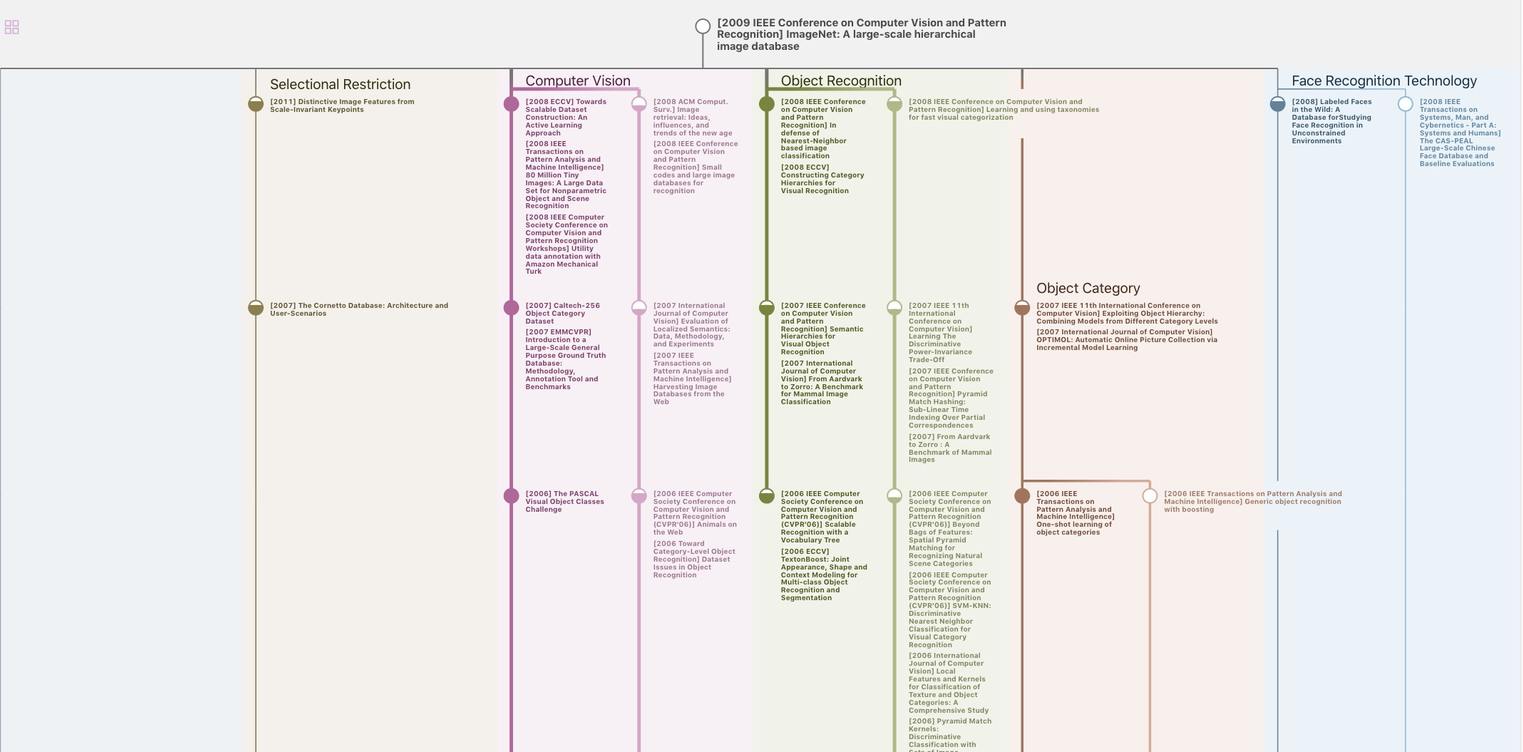
生成溯源树,研究论文发展脉络
Chat Paper
正在生成论文摘要