Ensemble of Supervised and Unsupervised Learning Models to Predict a Profitable Business Decision
2021 IEEE INTERNATIONAL IOT, ELECTRONICS AND MECHATRONICS CONFERENCE (IEMTRONICS)(2021)
摘要
Real-Estate rent prediction in housing market analysis plays a key role in calculating the Rate of Return - a salient index used to evaluate real-estate investment options. Accurate rent prediction in real estate investment can help in generating capital gains and guaranty a financial success. In this paper, we carry out a comprehensive analysis and study of seven machine learning algorithms for rent prediction, including Linear Regression, Multilayer Perceptron, Random Forest, KNN, Locally Weighted Learning, SMO, and KStar algorithms. We train new model for the US territory, including three house types of single-family, townhouse, and condo. Each data instance in the dataset has 21 internal attributes (e.g., area space, price, number of bed/bathroom, rent, school rating, so forth). A subset of the collected features selected by filter methods for the prediction models. We also employ a hierarchical clustering approach to cluster the data based on two factors of house type, and average rent estimate of zip codes. The empirical results suggest that the rent prediction models based on lazy learning algorithms lead to higher accuracy and lower prediction error compared to eager learning methods.
更多查看译文
关键词
housing analytics, applied machine learning, rent prediction, data mining
AI 理解论文
溯源树
样例
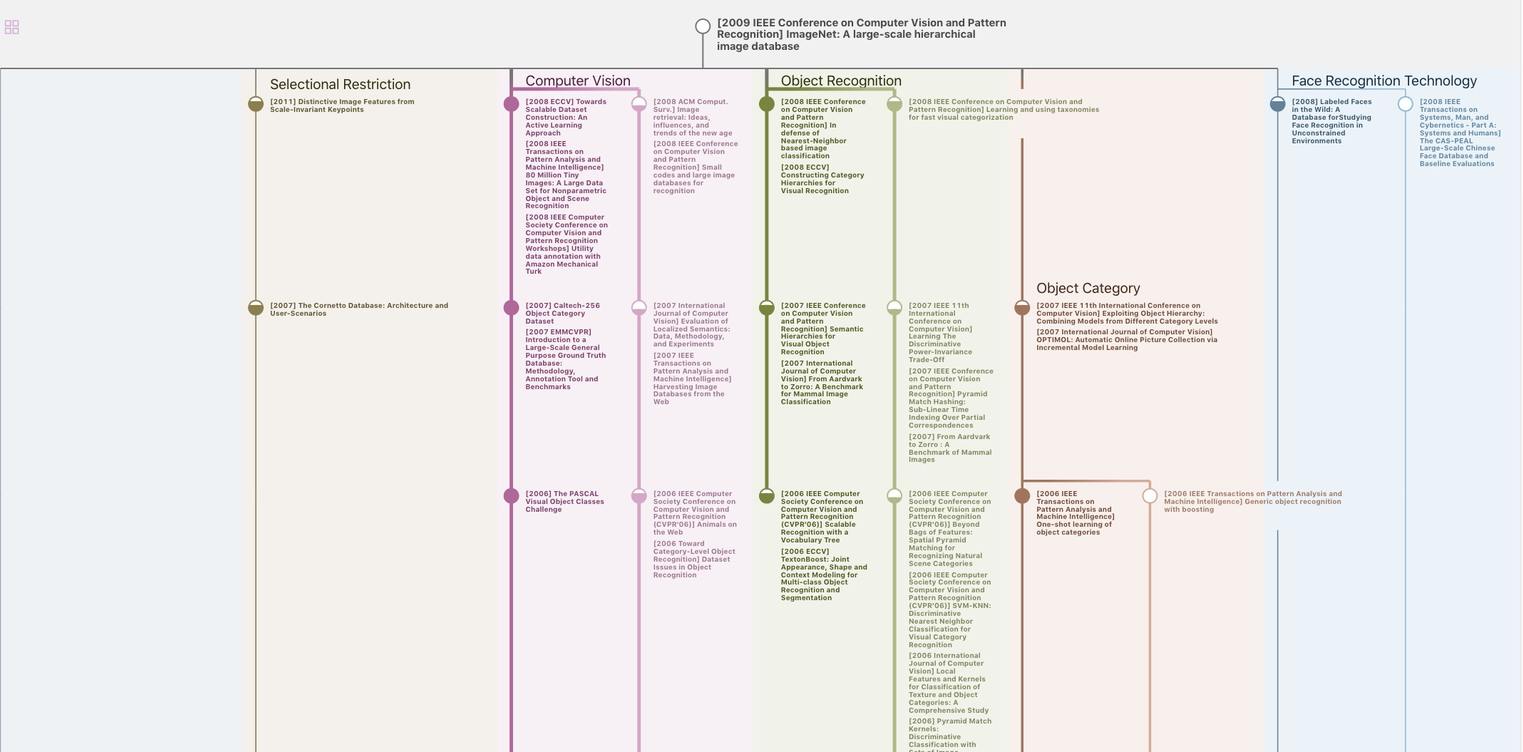
生成溯源树,研究论文发展脉络
Chat Paper
正在生成论文摘要