Mlpd: Multi-Label Pedestrian Detector In Multispectral Domain
IEEE ROBOTICS AND AUTOMATION LETTERS(2021)
摘要
Multispectral pedestrian detection has been actively studied as a promising multi-modality solution to handle illumination and weather changes. Most multi-modality approaches carry the assumption that all inputs are fully-overlapped. However, these kinds of data pairs are not common in practical applications due to the complexity of the existing sensor configuration. In this letter, we tackle multispectral pedestrian detection, where all input data are not paired. To this end, we propose a novel single-stage detection framework that leverages multi-label learning to learn input state-aware features by assigning a separate label according to the given state of the input image pair. We also present a novel augmentation strategy by applying geometric transformations to synthesize the unpaired multispectral images. In extensive experiments, we demonstrate the efficacy of the proposed method under various real-world conditions, such as fully-overlapped images and partially-overlapped images, in stereo-vision. Code and a demonstration video are available at https://github.com/sejong-rcv/MLPD-Multi-Label-Pedestrian-Detection.
更多查看译文
关键词
Deep learning for visual perception, object detection, multispectral pedestrian detection
AI 理解论文
溯源树
样例
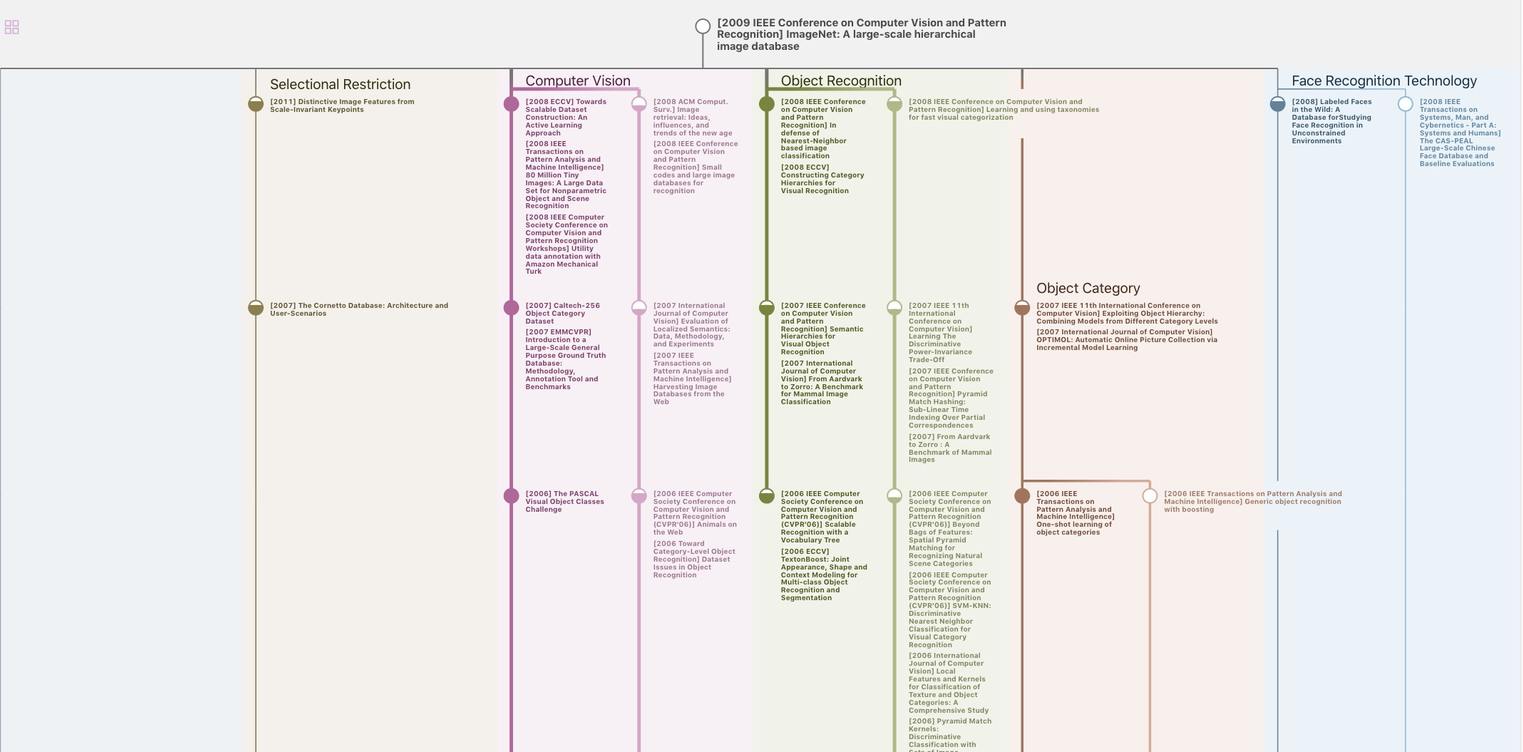
生成溯源树,研究论文发展脉络
Chat Paper
正在生成论文摘要