Multi-Objective Optimization With Multi-View Attention For A Knowledge-Graph Enhanced-Recommendation System
ELEKTROTEHNISKI VESTNIK(2021)
摘要
The recommendation system (RS) has become an essential component of various e-commerce platforms. Its purpose is to predict the next interaction item based on the user's information and his/her interaction history. As the existing methods have not flexibly used the external knowledge, the potential knowledge-level correlation between products can not be fully utilized. Therefore, the results recommended for users are limited to simple models and can not be reasonably extended. We propose a novel model which takes the multi-task learning with a multi-view attention for recommendation system (RS) and knowledge graph embedding (KGE) tasks simultaneously, motivated by the following. First, RS and KGE both involve embedding learning problems, one of which is at the item level and the other at the knowledge level. Second, these two tasks can help each other to improve. In other words, RS can incorporate the external knowledge from the knowledge graph, and KGE can be enhanced by learning a contextual information from RS. To improve the interactive process between these two tasks, we propose a novel multi-task learning scheme, which ingeniously employs a multi-view attention learned from various views on interacting these tasks more effectively and learning the embedding representation more comprehensively. The experiments conducted on several standard datasets demonstrate the effectiveness of the proposed method and an improvement in RS and KGE.
更多查看译文
关键词
neural networks, multi-task learning, multi-view attention, recommendation system (RS), knowledge graph (KG), knowledge graph embedding (KGE)
AI 理解论文
溯源树
样例
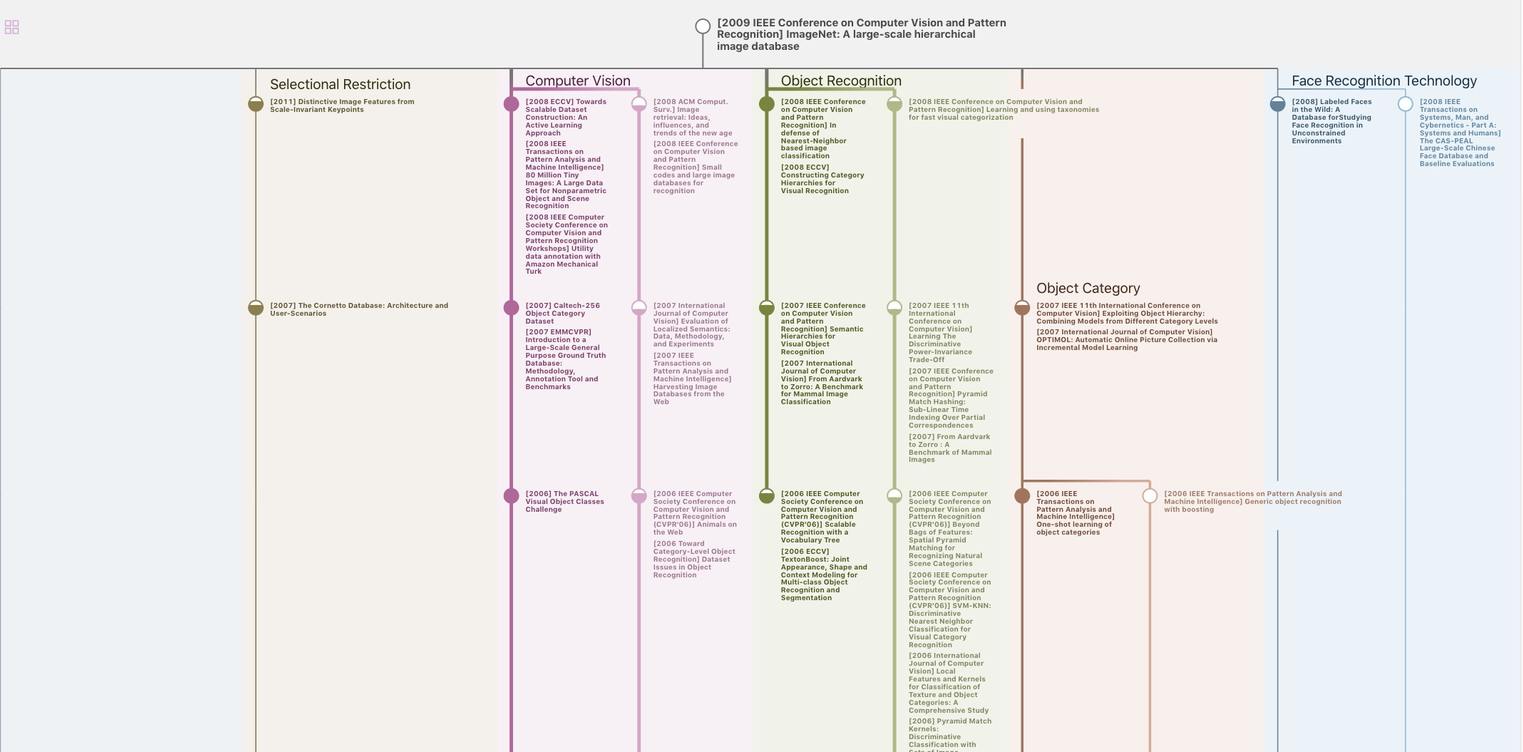
生成溯源树,研究论文发展脉络
Chat Paper
正在生成论文摘要