Critical Role Of Temporal Contexts In Evaluating Urban Cellular Automata Models
GISCIENCE & REMOTE SENSING(2021)
摘要
Cellular automata (CA)-based models have been extensively used in urban expansion modeling because of their simplicity, flexibility and intuitiveness. Previous studies on CA-based urban growth modeling have mainly focused on the process of spatial allocation of increased urban lands; however, the temporal contexts during the simulation have not been properly explored. In this study, we examined the influence of temporal contexts of initial seeds (i.e. urban extent maps), transition rules, and urban demands (i.e. urban areas) on the CA-based urban growth modeling in Beijing, China, over a long period of 1984-2013. Comparison of the annual model outputs with the time series data of annual urban extent maps from satellite observations revealed that the overall accuracy of urban growth modeling decreased by approximately 12%, with an increase in iterations from 1984-2013. By contrast, the value of the figure of merit (FoM) increased to 26.57%. The continuous change of FoM during the modeling suggests a "spin-up" effect, a rapid increase in FoM at the beginning of modeling, of CA-based urban growth models, and this effect is primarily attributed to the neighborhood component in CA. The effect of temporal contexts reflected by components of initial seeds and urban demands in CA-based urban growth models have considerable impacts on the model performance, i.e. the FoM increased by 7% when using actual urban demands during each iteration instead of the commonly used linear growth during the modeling period. Hence, we suggest that more efforts regarding the temporal contexts in CA-based modeling are required, to better understand error propagation and uncertainty assessment.
更多查看译文
关键词
Initial seeds, urban demands, transition rules, annual change, neighborhood
AI 理解论文
溯源树
样例
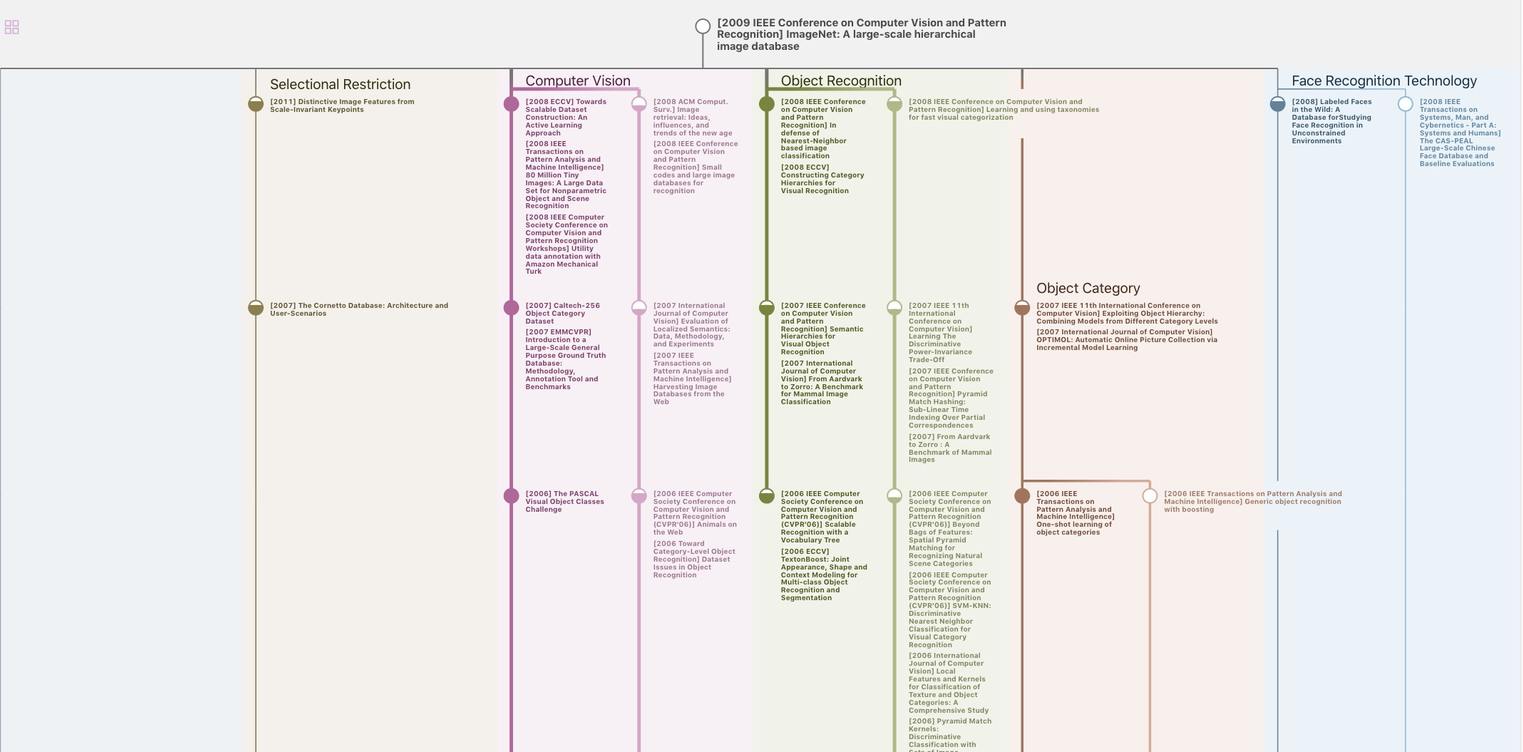
生成溯源树,研究论文发展脉络
Chat Paper
正在生成论文摘要