Automated In Situ Seed Variety Identification via Deep Learning: A Case Study in Chickpea
PLANTS-BASEL(2021)
摘要
On-time seed variety recognition is critical to limit qualitative and quantitative yield loss and asynchronous crop production. The conventional method is a subjective and error-prone process, since it relies on human experts and usually requires accredited seed material. This paper presents a convolutional neural network (CNN) framework for automatic identification of chickpea varieties by using seed images in the visible spectrum (400-700 nm). Two low-cost devices were employed for image acquisition. Lighting and imaging (background, focus, angle, and camera-to-sample distance) conditions were variable. The VGG16 architecture was modified by a global average pooling layer, dense layers, a batch normalization layer, and a dropout layer. Distinguishing the intricate visual features of the diverse chickpea varieties and recognizing them according to these features was conceivable by the obtained model. A five-fold cross-validation was performed to evaluate the uncertainty and predictive efficiency of the CNN model. The modified deep learning model was able to recognize different chickpea seed varieties with an average classification accuracy of over 94%. In addition, the proposed vision-based model was very robust in seed variety identification, and independent of image acquisition device, light environment, and imaging settings. This opens the avenue for the extension into novel applications using mobile phones to acquire and process information in situ. The proposed procedure derives possibilities for deployment in the seed industry and mobile applications for fast and robust automated seed identification practices.
更多查看译文
关键词
Cicer arietinum,convolutional neural network,grad-CAM,image classification,ImageNet,VGGNet,VGG16
AI 理解论文
溯源树
样例
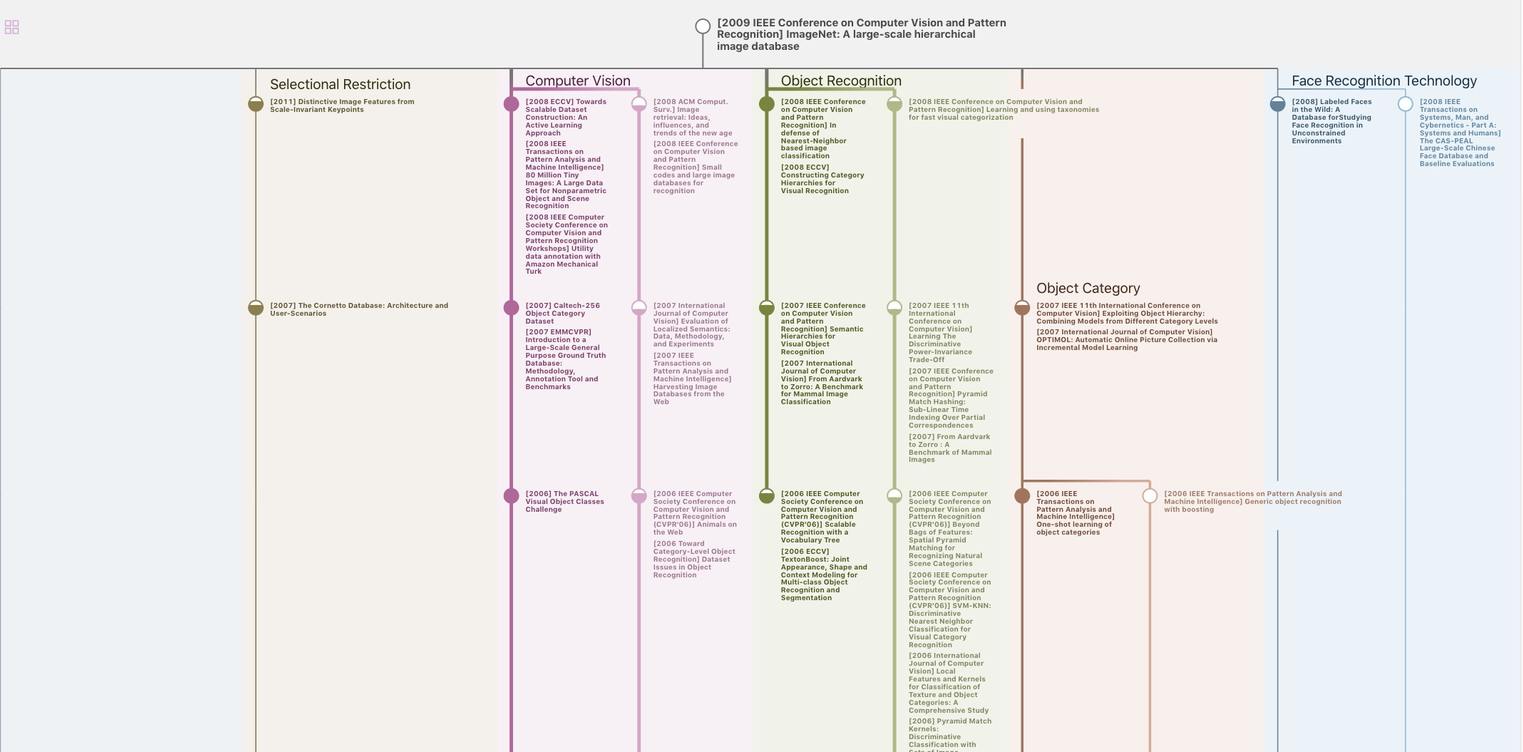
生成溯源树,研究论文发展脉络
Chat Paper
正在生成论文摘要