Multi-Stage Degradation Homogenization for Super-Resolution of Face Images With Extreme Degradations
IEEE TRANSACTIONS ON IMAGE PROCESSING(2021)
摘要
Face Super-Resolution (FSR) aims to infer High-Resolution (HR) face images from the captured Low-Resolution (LR) face image with the assistance of external information. Existing FSR methods are less effective for the LR face images captured with serious low-quality since the huge imaging/degradation gap caused by the different imaging scenarios (i.e., the complex practical imaging scenario that generates test LR images, the simple manual imaging degradation that generates the training LR images) is not considered in these algorithms. In this paper, we propose an image homogenization strategy via re-expression to solve this problem. In contrast to existing methods, we propose a homogenization projection in LR space and HR space as compensation for the classical LR/HR projection to formulate the FSR in a multi-stage framework. We then develop a re-expression process to bridge the gap between the complex degradation and the simple degradation, which can remove the heterogeneous factors such as serious noise and blur. To further improve the accuracy of the homogenization, we extract the image patch set that is invariant to degradation changes as Robust Neighbor Resources (RNR), with which these two homogenization projections re-express the input LR images and the initial inferred HR images successively. Both quantitative and qualitative results on the public datasets demonstrate the effectiveness of the proposed algorithm against the state-of-the-art methods.
更多查看译文
关键词
Degradation,Faces,Training,Imaging,Image reconstruction,Tools,Image quality,Face super-resolution,data re-expression,degradation gap,projection bridge
AI 理解论文
溯源树
样例
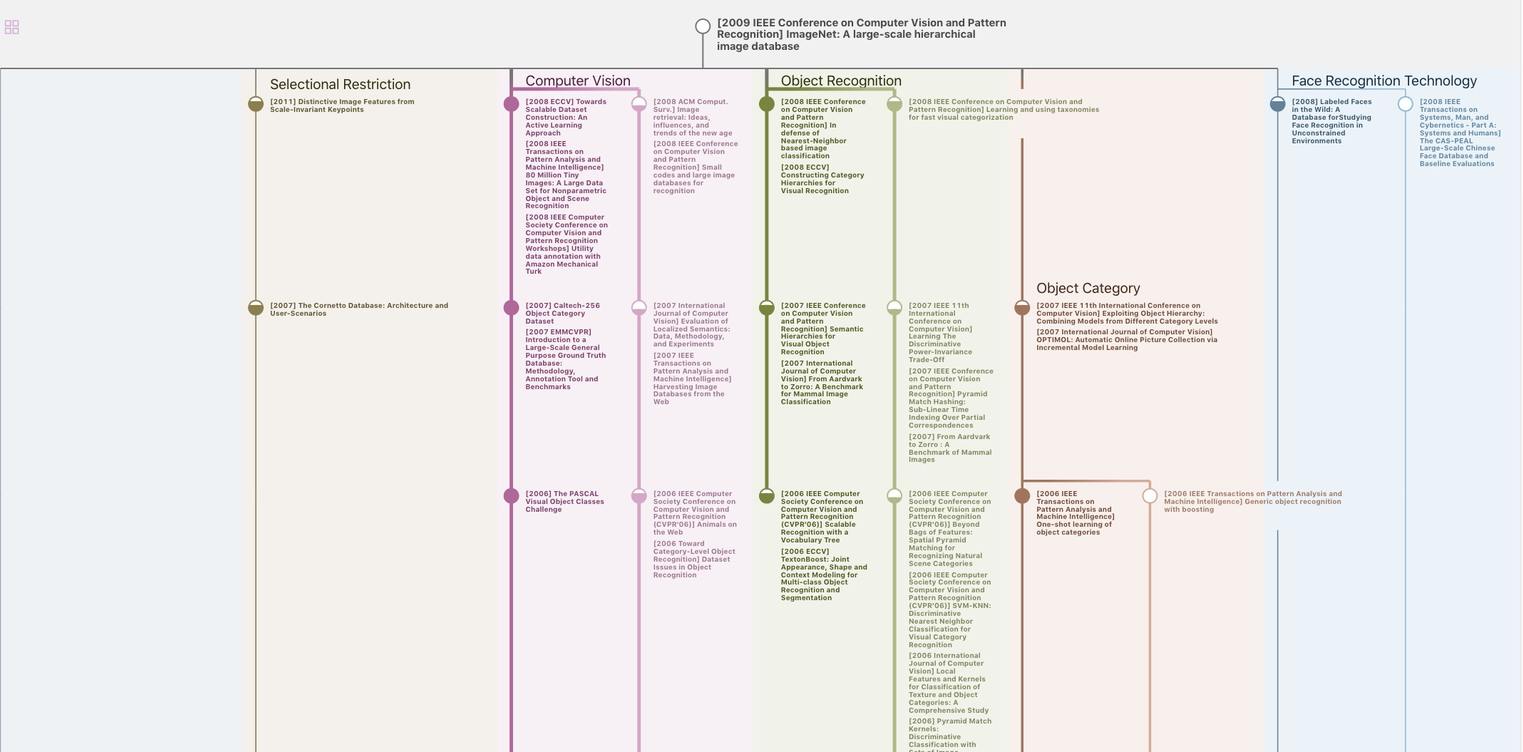
生成溯源树,研究论文发展脉络
Chat Paper
正在生成论文摘要