A forecasting framework for predicting perceived fatigue: Using time series methods to forecast ratings of perceived exertion with features from wearable sensors
APPLIED ERGONOMICS(2021)
摘要
Advancements in sensing and network technologies have increased the amount of data being collected to monitor the worker conditions. In this study, we consider the use of time series methods to forecast physical fatigue using subjective ratings of perceived exertion (RPE) and gait data from wearable sensors captured during a simulated in-lab manual material handling task (Lab Study 1) and a fatiguing squatting with intermittent walking cycle (Lab Study 2). To determine whether time series models can accurately forecast individual response and for how many time periods ahead, five models were compared: naive method, autoregression (AR), autoregressive integrated moving average (ARIMA), vector autoregression (VAR), and the vector error correction model (VECM). For forecasts of three or more time periods ahead, the VECM model that incorporates historical RPE and wearable sensor data outperformed the other models with median mean absolute error (MAE) < 1.24 and median MAE < 1.22 across all participants for Lab Study 1 and Lab Study 2, respectively. These results suggest that wearable sensor data can support forecasting a worker's condition and the forecasts obtained are as good as current state-of-the-art models using multiple sensors for current time prediction.
更多查看译文
关键词
Fatigue forecast,Material Handling,Vector Error Correction Model,Wearable Sensors
AI 理解论文
溯源树
样例
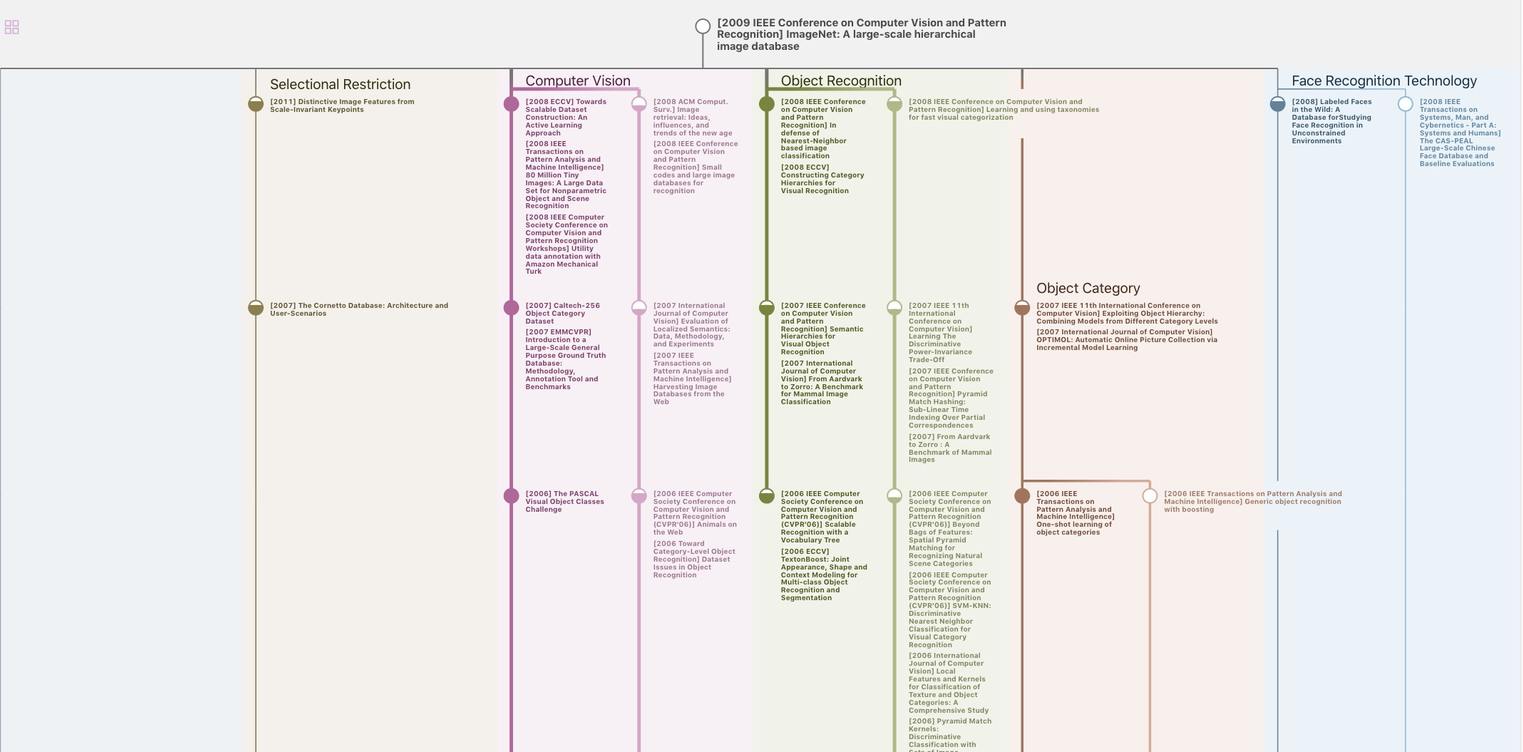
生成溯源树,研究论文发展脉络
Chat Paper
正在生成论文摘要