Deep Ensemble Learning With Non-Equivalent Costs Of Fault Severities For Rolling Bearing Diagnostics
JOURNAL OF MANUFACTURING SYSTEMS(2021)
摘要
Effective fault diagnostics on rolling bearings is vital to ensuring safe and reliable operations of industrial equipment. In recent years, enabled by Machine Learning (ML) algorithms, data-based fault diagnostics approaches have been steadily developed as promising solutions to support industries. However, each ML algorithm exhibits some shortcomings limiting its applicability in practice. To tackle this issue, in this paper, Deep Learning (DL) and Ensemble Learning (EL) algorithms are integrated as a novel Deep Ensemble Learning (DEL) approach. In the DEL approach, the training requirements for the DL algorithm are alleviated, and the accuracy for fault condition classifications is enhanced by the EL algorithm. The DEL approach is comprised of the following critical steps: (i) Convolutional Neural Networks (CNNs) are constructed to pre-process vibration signals of rolling bearings to extract fault-related preliminary features efficiently; (ii) decision trees are designed to optimise the extracted features by quantifying their importance contributing to the faults of rolling bearings; (iii) the EL algorithm, which is enabled by a Gradient Boosting Decision Tree (GBDT) algorithm and a Non-equivalent Cost Logistic Regression (NCLR) algorithm, is developed for fault condition classifications with optimised nonequivalent costs assigned to different fault severities. Case studies demonstrate that the DEL approach is superior to some other comparative ML approaches. The industrial applicability of the DEL approach is showcased via the case studies and analyses.
更多查看译文
关键词
Rolling bearing fault diagnosis, Convolutional Neural Network, Ensemble Learning, Gradient Boosting Decision Tree
AI 理解论文
溯源树
样例
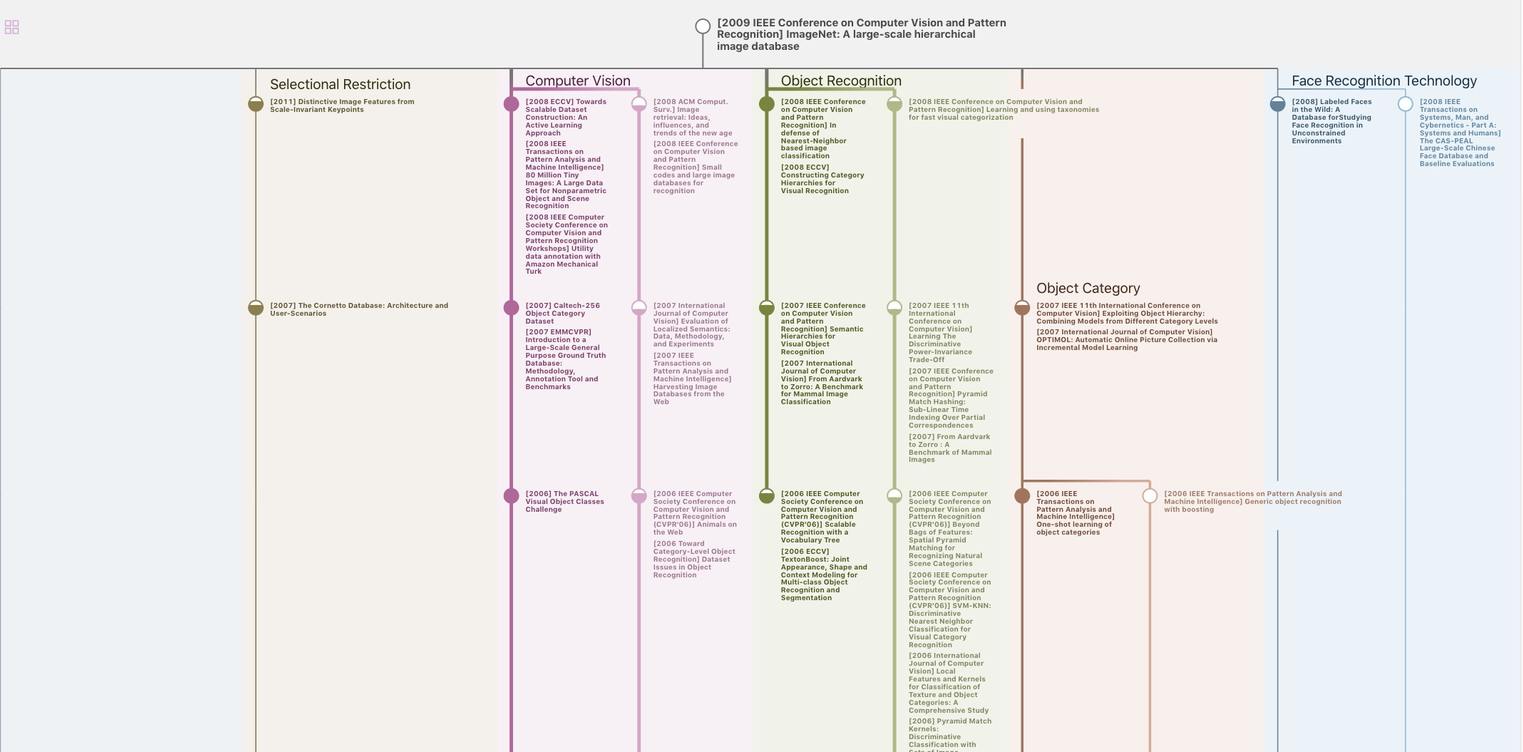
生成溯源树,研究论文发展脉络
Chat Paper
正在生成论文摘要