Deep learning on digital mammography for expert-level diagnosis accuracy in breast cancer detection
Multimedia Systems(2021)
摘要
Recently, computer-aided diagnosis (CAD) systems powered by deep learning (DL) algorithms have shown excellent performance in the evaluation of digital mammography for breast cancer diagnosis. However, such systems typically require pixel-level annotations by expert radiologists which is prohibitively time-consuming and expensive. Medical institutes would wonder if a high-performance breast cancer CAD system can be trained by exploring their own huge amount of historical imaging data and corresponding diagnosis reports, without additional annotations workload of their radiologists. In this study, we show that a DL classification model trained on historical mammograms with only image-level pathology labels (which can be automatically extracted from medical reports) can achieve surprisingly good diagnostic performance on newly incoming exams compared with experienced radiologists. A DL model called DenseNet was trained and cross-validated with 5979 historical exams acquired before September 2017 with biopsy-verified pathology and tested with 1194 newly obtained cases after that. For both cross-validation and test sets, the ROCs generated by DL predictions were above the ROCs generated by ratings from radiologists. For the suspicious cases which radiologists suggest biopsy (BI-RADS category 4 and 5), the DL model can reject 60% of false biopsies on benign breasts while keeping 95% sensitivity. For the mammograms based on which radiologists were not able to make a diagnosis (BI-RADS 0), the DL model still achieved an AUC score of 79%. Moreover, the model is able to localize lesions on mammograms although such information was not provided in the training phase. Finally, the impact of input image resolution and different DL model architectures on the diagnostic accuracy were also presented and analyzed.
更多查看译文
关键词
Deep learning, Digital mammography, Breast cancer, Diagnostic accuracy, Artificial intelligence
AI 理解论文
溯源树
样例
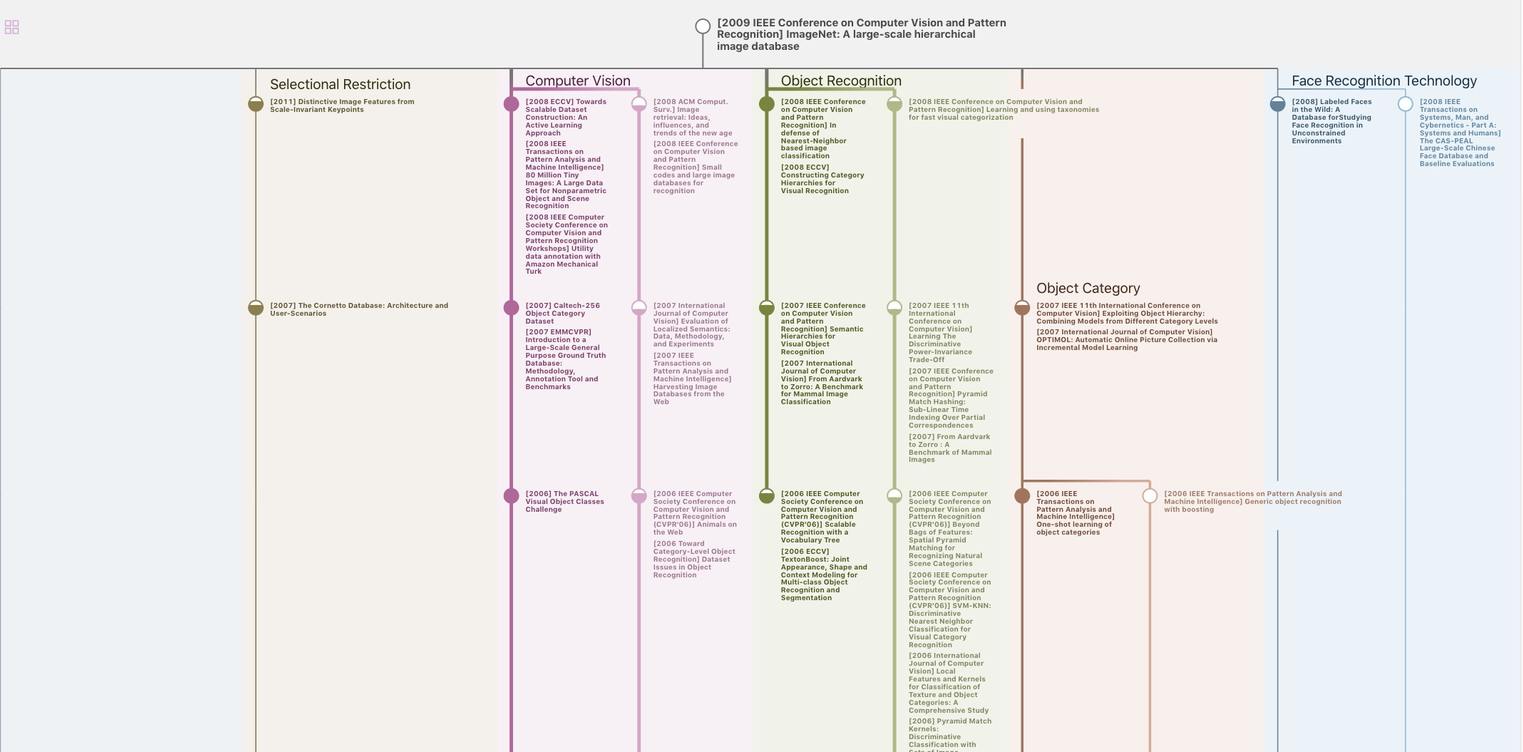
生成溯源树,研究论文发展脉络
Chat Paper
正在生成论文摘要