Robust Image Watermarking Against Jpeg Recompression For Websites
THIRTEENTH INTERNATIONAL CONFERENCE ON DIGITAL IMAGE PROCESSING (ICDIP 2021)(2021)
摘要
A robust image water larking method based on neural network is proposed for websites, such as the social networks (Facebook, Twitter), which adopts different JPEG recompression strategies for uploaded images. The basic network structure consists of three parts: the encoder inputs a cover image and watermark message, outputs the watermarked image and realizes watermark embedding; the decoder inputs a watermarked image, outputs extracted watermark message; the discriminator and the encoder constitute a generating antagonistic network, which promotes the encoder to generate watermarked images which are inseparable from the cover image. A noise layer is designed in the network structure to simulate the attacks that watermarking may be subjected to, and the whole network is trained end-to-end to make the watermarking model robust to the attacks. The experimental results show that the noise layer is designed to simulate JPEG recompression process reasonably, and the model is robust to different JPEG recompression processes on Facebook and Twitter, with the average bit error rate less than 1%. In fact, embedding watermark adds noise to the cover image; considering the influence of the counter sample on the deep neural network, this paper verifies the interference of embedding watermark to the target classification network. By adding the target classification module into the original network structure, the influence of watermark embedding on the target classification network is considered at the same time. The experimental results show that the target classification module is helpful to reduce the interference of watermark embedding on various target classification networks.
更多查看译文
关键词
robust image watermarking, JPEG recompression, target classification, neural network
AI 理解论文
溯源树
样例
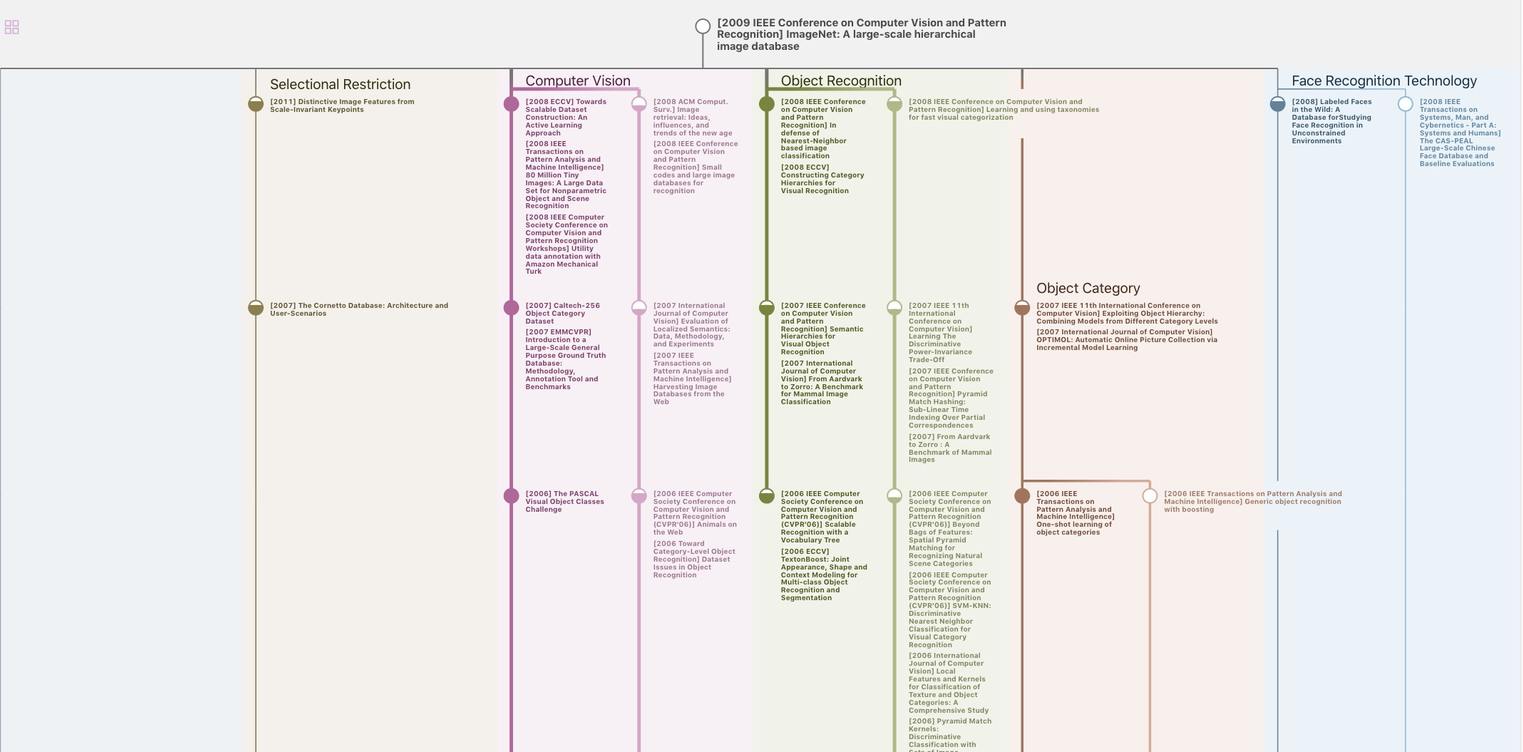
生成溯源树,研究论文发展脉络
Chat Paper
正在生成论文摘要